350 rub
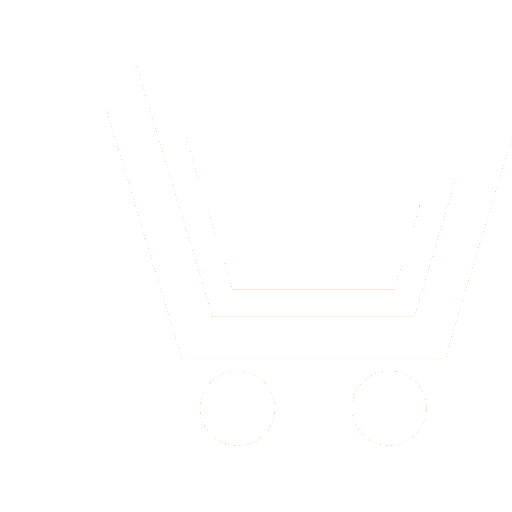
Journal Nonlinear World №4 for 2014 г.
Article in number:
Nonlinear dynamical models of the network traffic
Authors:
A. V. Kolesnikov - Post-graduate student of the - Theoretical Informatics and Computer Technologies - department, Bauman Moscow State Technical University
I. P. Ivanov - Ph.D. (Eng.), vice-rector on informatization, head of the - Theoretical Informatics and Computer Technologies - department, Bauman Moscow State Technical University
M. A. Basarab - Dr. Sc. (Phys.-Math.), professor of «Information Security» department, Bauman Moscow State Technical University
I. P. Ivanov - Ph.D. (Eng.), vice-rector on informatization, head of the - Theoretical Informatics and Computer Technologies - department, Bauman Moscow State Technical University
M. A. Basarab - Dr. Sc. (Phys.-Math.), professor of «Information Security» department, Bauman Moscow State Technical University
Abstract:
In computer processing and storage of information it is necessary to exchange data between the actors. So, since late 70s the rapid development of computer networks and related equipment begins. Local and wide area networks evolve every year. The number of connected subscribers increases, as well as the total amount of transmitted information. This fast development trend raises a number of problems. Intensive exchange of data requires increased computing power and can lead to a reduction in the quality of service (QoS). Network topology, transportation protocol, different types of web services and many other reasons can influence on characteristics of data traffic. So the actual mathematical models of traffic which could help to optimize the network load are needed. They are also will be useful in developing software and hardware tools for increasing network reliability. Obviously, that macro parameters of network traffic are determined by administrators. But the parameters on timescales of microseconds are defined by hardware and transportation environment. So research of servers hardware and operating system behavior could be another useful instrument in the task of network load optimization. Investigation of the memory layout, CPU, operating system state and other characteristics of the host may reveal hidden correlations, cycles and phase transitions in the network traffic distribution. In this work, on the example of a Bauman MSTU corporative network server, methods for nonlinear analysis of incoming and outgoing traffic dynamical characteristics are considered. The Lyapunov exponent and Hurst exponent of the network traffic, characterizing chaotic properties of the processes, were evaluated. Phase diagrams of traffic were investigated and attractors were revealed, allowing detailed analysis of the impact of load on the network traffic capacity. For all processes, the self-similarity was found, confirming the possibility of using fractal models for working with data, including the prediction of the behavior of the time series. A simulation model of a computer network is developed and its main characteristics are investigated. Conclusions about the applicability of the developed model to study the real corporate network are made.
Pages: 44-56
References
- Ivanov I. P., Boychenko M. K. Monitoring of Corporative Network Resources. Vestnik MGTU. Priborostroenie. 2010. No. 2. P. 114‑120. (in Russian).
- Boychenko M. K., Ivanov I. P., Kondratiev A. Yu. Availability of Resources of Transport Subsystems of Corporative Networks. Vestnik MGTU. Priborostroenie. 2010. No. 3. P. 103‑118. (in Russian).
- Stallings W. Data and Computer Communications. Upper Saddle River, NJ: Prentice Hall, 2010.
- Tanenbaum A. Computer Networks. Upper Saddle River, NJ: Prentice Hall, 1981.
- Kashin M.M., Development of the Method for SIP-networks Overload Control on the Base of the Signal Traffic Forecast. Cand. Sci. (Eng.) dissertation. Samara, 2011. (in Russian).
- Heyman, D. P., Sobel M. J. Stochastic Models in Operations Research. V. I. Stochastic Processes and Operating Characteristics. New York: McGraw-Hill, 1982.
- Leland W. E., Taqqu M. S., Willinger W., Wilson D. V. On the Self-Similar Nature of Ethernet Traffic (Extended Version). IEEE/ACM Transactions on Networking. V.2. No.1. February 1994.
- Willinger W., Taqqu M. S., Sherman R., Wilson D. V. Self-Similarity Through High-Variability: Statistical Analysis of Ethernet LAN Traffic at the Source Level. IEEE/ACM Transactions on Networking. V. 5, No. 1. Feb. 1997. P. 71‑86.
- Vinogradov N. A., Lesnaya N. N., Savchenko A. S., Kolisnik O. V. Investigation of Characteristics of Useful Capacity under Conditions of Variable Load. Problemy informatizatsii ta upravlinnya. Kiev: National Aviation University, 2009. Issue 4 (28). P.28‑31 (in Russian).
- Shelukhin I. O., Tenyakshev A. M., Osin A. V. Fractal Processes in Telecommunications. Moscow: Radiotekhnika, 2003. (in Russian).
- Shelukhin I.O., Osin A. V., Smolskii S. M. Self-similarity and Fractals. Telecommunication Applications. Moscow: Fizmatlit, 2008 (in Russian).
- Basarab M. A., Ivanov I. P., Kolesnikov A. V., Kolobaev L. I. University Corporative Network Traffic Analysis Based on the Methods of Nonlinear Dynamics // Proc. of the Tenth Intern. Conf. - Computer Data Analysis and Modeling: Theoretical and Applied Stochastics?, Minsk, Sept. 10-14, 2013. V.2. Minsk: Publ. center of BSU, 2013. P.99-105.
- Hunt G. A. Random Fourier transforms. Trans. Amer. Math. Soc. 1951. V. 71. P. 38‑69.
- Hurst H., Black R., Long-Term Storage: An Experimental Study. London: Constable, 1965.
- Hurst H. E. Long-Term Storage Capacity of Reservoirs. Transactions of the American Society of Civil Engineering. No. 116. 1951. P. 770-799.
- User manual Zabbix. http://www.zabbix.com/en/documentation.php.
- Kirillov D.S., Korob O.V., Mitin N.A., Orlov Yu.N., Pleshakov R.V. Distribution of the Hurst Exponent of a Nonstationary Marked Time Series. Keldysh Institute of Applied Mathematics. Preprints. 2013. No. 11.
- Schroeder M. Fractals, Chaos, Power Laws: Minutes from an Infinite Paradise. NY: W.H. Freeman and Co., 1991.
- Rosenstein M. T., Collins J. J., De Luca C. J. A Practical Method for Calculating Largest Lyapunov Exponents from Small Data Sets. Physica D. 1993. V.65. P. 117-134.
- Ivanov I. P., Mathematical models, methods of analysis and management in corporate computer networks, BMSTU, PhD issue 2010.
- Konnova N. S. Digital Signal Processing of Blood Flow Volume Velocity Doppler Sensor for Transients in Microcirculatory Vessels.Science and Education. Electronic scientific and technical periodical. No. 12, December 2012, DOI: 10.7463/1212.0506267.
- Osowski S. Sieci neuronowe do przetwarzania informacji. Warszawa: Oficyna Wydawnicza Politechniki Warszawskiej, 2000.