350 rub
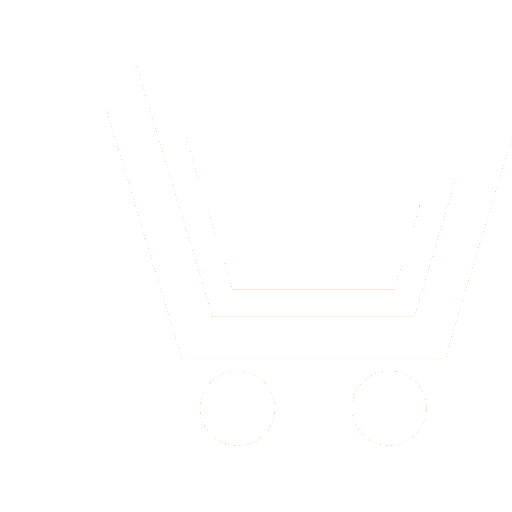
Journal Nonlinear World №11 for 2012 г.
Article in number:
Objects classification in the case analisys textured color images
Keywords:
classification
allocation of alpha channel
Bayesian estimation
robust estimation edge of objects on the textured images
Authors:
A.I. Sherstobitov, V.I. Marchuk, D.V. Timofeev, R.A. Sizyakin
Abstract:
In this paper we study methods for classifying objects in textured images. The nonlinear model of the mathematical component interaction processed signal. Introduced a nonlinear objective function of processing solutions for the problem of estimating the alpha channel, which separates the set of points from a variety of background object points by way its minimization for each pixel of the image. We study the method of classification algorithm set of points of the object and the background of the image by determining the alpha channel in a canonical form. Consider methods of classification based on Bayesian estimation method, gradient method, robust and spectral method. Are the advantages and disadvantages of the methods considered classification. The examples of the solution of the problem classification of the object on a color image and its separation on a textured background by different method, are presented. A comparative analysis of the processing, obtained by the methods of classification of objects on a set of textured images. As a criterion for evaluation of the values the probability of correct detection and false alarm probability, standard deviation of the true contour of the object from the estimate. The numerical values are presented assess the effectiveness of edge detection of objects in the processing of textured digital images in the table.
Pages: 769-776
References
- Фомин А.Ф., Новоселов О.Н., Плющев А.В. Отбраковка аномальных результатов измерений. М.: Энергоатомиздат. 1985.
- Гонсалес Р., Вудс Р. Цифровая обработка изображений. М.: Техносфера. 2005. 1072 с.
- Fattal R., Lischinski D., and Werman M. Gradient domain high dynamic range compression. In Proceedings of ACM SIGGRAPH. 2002. P. 249-256.
- Levin A., Lischinski D., Weiss Y. A closed form solution to natural image matting. in Proceedings of IEEE CVPR. 2006. P. 277-302.
- Levin A., Rav-Acha A., and Lischinski D. Spectral matting. Proc. of IEEE CVPR. 2007. P. 315-321.
- Ruzon M.A., Tomasi C. Alpha estimation in natural images. In CVPR. 2000. P. 18-25.
- Sun J., Jia J., Tang C.-K., Shum H.-Y. Poisson matting. in Proceedings of ACM SIG-GRAPH. 2004. P. 315-321.
- Wang J., Cohen M. Optimized color sampling for robust matting. Proc. of IEEE CVPR. 2007.
- Zomet A., Peleg S. Multi-sensor super resolution. In Proceedings of the IEEE Workshop on Applications of Computer Vision. 2002.
- Марчук В.И., Воронин В.В.Реконструкция значений утраченных пикселей изображений в условиях ограниченной априорной информации // Научно-технические ведомости Санкт-Петербургского государственного политехнического университета. 2009. № 72.С. 52-56.