350 rub
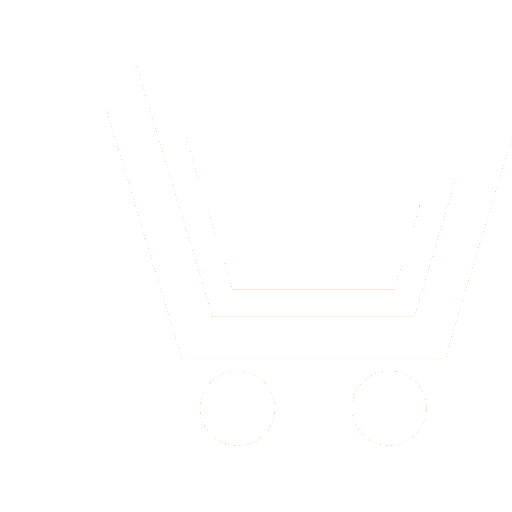
Journal Nonlinear World №5 for 2009 г.
Article in number:
Information Clusters as Dissipative Systems
Authors:
V.A. Raikhlin, D.O. Shagejev
Abstract:
The question on existence "fast" (i.e. with significant growth of system performance) attractors behind a side of scalability in database clusters is discussed. Interest to this question is caused observable in one of early works as the phenomenon "lost" with synchronous development of system. If the hypothesis "fast" attractors in due course will prove to be true, it will have serious consequences. The main task of clause is certain as development of the approach to change of cluster-s topology with the set number of units in dynamics of its work.
The hypothesis which has laid down in a basis of "a new sight" at a problem of load balancing in parallel cluster DBMS is formulated. This hypothesis connects expediency dynamic cluster reconfiguration with change of operating query stream parameters as primary parameters of system and an architecture choice model. They define a degree of influence on cluster-s productivity all factors (external and internal) in aggregate. Character of this influence remains to unknown persons. Therefore the developed model is local, is fair only for the set query stream, the scheme of a DB, its volume and a used platform.
The offered temporal-indistinct procedural model with use of the genetic search approach of the relevant knowledge base has shown the efficiency. It allows to predict the moments of reconfiguration. Rather low sensitivity of model distinction of architecture with close performance levels speaks a choice only three linguistic "granules". But it is not necessary to consider it its lack for at the specified distinctions reconfiguration is not obligatory
Pages: 323
References
- Анохин П.К. Принципиальные вопросы общей теории функциональных систем // Принципы системной организации функций. М.: Наука. 1973.
- Николис Г., Пригожин И. Познание сложного. М.: УРС. 2003.
- Райхлин В.А. Моделирование машин баз данных распределенной архитектуры // Программирование. 1996. №2.
- Райхлин В.А., Абрамов Е.В. К теории моделей синтеза кластеров баз данных // Вестник КГТУ им. А.Н. Туполева. 2004. №1.
- Главные компоненты временных рядов: метод «Гусеница» / под ред. Д.А. Данилова и А.А. Жиглявского. СПб.: Санкт-Петербургский университет, 1997.
- Абрамов Е.В. Параллельная СУБД Clusterix. Разработка прототипа и его натурное исследование // Вестник КГТУ им. А.Н. Туполева. 2006. №2.
- Райхлин В.А., Абрамов Е.В. Кластеры баз данных. Моделирование эволюции // Вестник КГТУ им. А.Н. Туполева. 2006. №3.
- Райхлин В.А., Абрамов Е.В., Шагеев Д.О. Эволюционное моделирование процесса выбора архитектуры кластеров баз данных // Труды 8-й Междунар. конф. «Высокопроизводит. паралл. вычисления на кластерных системах» HPC-2008. (Казань, ноябрь 17 - 19, 2008). Казань: Изд. КГТУ, 2008. С. 249 - 256.
- Воеводин В.В., Воеводин Вл.В. Параллельные вычисления. СПб.: БХВ-Петербург. 2004.
- Райхлин В.А.Конструктивное моделирование систем. Казань: ФЭН (Наука). 2005.
- Тейз А., Грибомон П., Луи Ж. и др. Логический подход к искусственному интеллекту: от классической логики к логическому программированию. М.: Мир. 1990.
- Тейз А., Грибомон П., Юлен Г. и др. Логический подход к искусственному интеллекту: от модальной логики к логике баз данных. М.: Мир. 1998.
- Малышев Н.Г., Берштейн Л.С., Боженюк А.В. Нечеткие модели для экспертных систем в САПР. М.: Энергоатомиздат. 1991.
- Аверкин А.Н., Батыршин И.З., Блишун А.Ф. Нечеткие множества в моделях управления и искусственного интеллекта / под ред. Д.А. Поспелова. М: Наука. 1986.
- Лукашин Ю.П. Адаптивные методы краткосрочного прогнозирования временных рядов. М.: Финансы и статистика, 2003.
- Дуброва Т.А. Статистические методы прогнозировангия. М.: ЮНИТИ. 2003.
- Holland John H. Adaptation in Natural and Artificial Systems: An Introductory Analysis with Application to Biology, Control and Artificial Intelligence. USA: University of Michigan. 1975.
- Soraya Rana. Examining the Role of Local Optima and Schema Processing in Genetic Search, 1999.