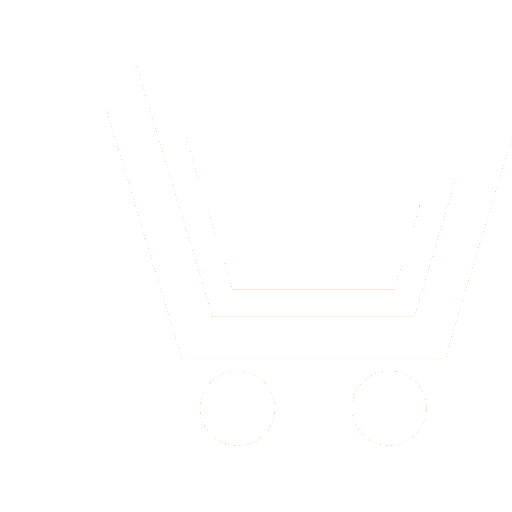
R.S. Ekhlakov1
1 Financial University under the Government of the Russian Federation (Moscow, Russia)
1 rsekhlakov@fa.ru
The problem of sustainable development of mobility in cities, as well as assessing and forecasting the state of traffic congestion plays a key role in reducing traffic congestion. For example, predicting travel time is one of the key parameters in route planning used in geographic information services. The gradual penetration of smart devices and high-speed Internet connections provides the opportunity to analyze data received from transport network participants in close to real time. It is necessary to develop algorithms for assessing and predicting the workload of large volumes of data using machine learning methods, which will improve the accuracy based on existing solutions. Machine learning helps identify and take into account patterns to improve route analysis and travel time calculations. Increasing the efficiency of assessing and predicting the congestion of the transport network to improve the quality of the laid route in close to real time. A model has been developed for assessing and forecasting the congestion of the transport network based on anonymous data from vehicle drivers in close to real time. A comparison of methods for forecasting workload using ma-chine and deep learning is presented. Research can serve as a starting point for further study of the problem in order to achieve even greater accuracy in predicting the congestion of the transport network, as well as creating an online service for building rational routes in close to real time.
Ekhlakov R.S. Real-time model for assessing and forecasting transport network congestion. Neurocomputers. 2024. V. 26. № 6. Р. 126-134. DOI: https://doi.org/10.18127/j19998554-202406-15 (In Russian)
- Vyugina A.S., Kublik E.I., Chipchagov M.S., Labintsev A.I. Automated city security systems based on sound data analysis. Information-measuring and Control Systems. 2022. V. 20. № 6. P. 50−57. DOI 10.18127/j20700814-202206-06. (in Russian)
- Yekhlakov R.S. Modern modeling traffic flow methods. Multicriteria asses sment of route rationality model. Scientific and Technical Bulletin of the Volga region. 2023. № 11. P. 70–73. (In Russian)
- Marosin V.S., Gusev S.A. Network planning models in the management of truck transportation. Materials of the 4th International Scientific and Practical Conference "Information technologies and innovations in transport". Orel: Oryol State University named after I.S. Turgenev. 2019. P. 65–70. (In Russian)
- Shvetsov V.I. Mathematical modeling of traffic flows. Automation and Remote Control. 2003. V. 64. № 11. P. 1651–1689. DOI 10.1023/A:1027348026919.
- Ekhlakov R. Modern Methods of Traffic Flow Modeling: A Graph Load Calculation Model Based on Real-Time Data. Intelligent Decision Technologies. 2023. P. 302–309. DOI 10.1007/978-981-99-2969-6_27.
- Duan Y., L.V. Y., Wang F.-Y. Travel time prediction with LSTM neural network. IEEE 19th International Conference on Intelligent Transportation Systems. 2016. P. 1053–1058. DOI 10.1109/ITSC.2016.7795686.
- Liu Y., Wang Y., Yang X. Zhang L. Short-term travel time prediction by deep learning: A comparison of different LSTM-DNN models. IEEE 20th International Conference on Intelligent Transportation Systems. 2017. P. 1–8. DOI 10.1109/ITSC.2017.8317886.
- Fu R., Zhang Z., Li L. Using LSTM and GRU neural network methods for traffic flow prediction. 31st Youth Academic Annual Conference of Chinese Association of Automation. 2016. P. 324–328. DOI 10.1109/YAC.2016.7804912.
- Yu H., Wu Z., Wang S., Wang Y., Ma X. Spatiotemporal recurrent convolutional networks for traffic prediction in transportation networks. Sensors. 2017. V. 17. № 7. P. 1501. DOI 10.3390/s17071501.
- Cui Z., Henrickson K., Ke R., Wang Y. High-Order Graph Convolutional Recurrent Neural Network: A Deep Learning Framework for Network-Scale Traffic Learning and Forecasting. [Electronic resource] – Access mode: https://arxiv.org/pdf/1802.07007v1, date of reference 25.08.2024.