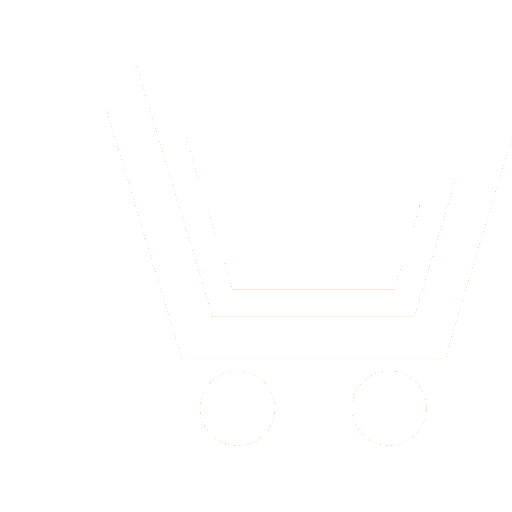
I.V. Statsеnko1, N.A. Andriyanov2, O.S. Shishkin3
1–3 Financial University under the Government of the Russian Federation (Moscow, Russia)
1,3 foreth35@gmail.com, 2 naandriyanov@fa.ru
When synthetic data is used in the training sample, models are trained much worse on the generation task. This phenomenon is called Model collapse. The solution of this problem will potentially provide models with much more data for training while maintaining high generation quality. This applies to both text and image models.
The main goal of this paper is to study the phenomenon of Model collapse in more detail. To review the existing methods of combating this phenomenon and to form directions for further research of our team.
The existing methods of combating Model collapse at the moment of writing the article are considered. The disadvantages of these methods are revealed. The directions for future research are outlined. Thus, the approaches to struggle with deep learning models collapse are systematized.
Since synthetic data are ubiquitous, it is important to know methods to improve model learning when encountering such data. For researchers in the field, possible topics for future work are identified. The results will be useful both to specialists who lack data for some narrow problem to be solved and to experts in the field of training large generative models.
Statsеnko I.V., Andriyanov N.A., Shishkin O.S. Current state of research on the collapse problem of deep learning models. Neurocomputers. 2024. V. 26. № 6. Р. 55-64. DOI: https://doi.org/10.18127/j19998554-202406-08 (In Russian)
- Andriyanov N.A., Kulichenko Ya.V. Applying generative image models to augment face detector training data. Neurocomputers. 2023. V. 25. № 4. Р. 7–15. DOI 10.18127/j19998554-202305-02. (In Russian)
- Burygin A.O., Panin I.G. Dual defect detection and generation system in planar surface. Neurocomputers. 2024. V. 26. № 3. Р. 55–67. DOI 10.18127/j19998554-202403-06. (In Russian)
- Kuznetsov A.V., Dimitrov D.V., Groshev A.Yu., Paramonov P.P., Maltseva A.A. Computer vision technologies in the synthesis of high-quality multimedia content. Reports of the Russian Academy of Sciences. Mathematics, computer science, management processes. 2022. V. 508. № 1. P. 109–110. DOI 10.31857/S2686954322070141. (In Russian)
- Andriyanov N.A. Pseudogradient procedures in problems of estimating parameters of image models. Proceedings of the 26th International Conference. Crimean conference "Microwave equipment and telecommunication technologies". 2016. P. 2705–2710. (In Russian)
- Andriyanov N., Andriyanov D. Pattern Recognition on Radar Images Using Augmentation. Ural Symposium on Biomedical Engineering, Radioelectronics and Information Technology. 2020. P. 0289–0291. DOI 10.1109/USBEREIT48449.2020.9117669.
- Shumailov I., Shumaylov Z., Zhao Y., Gal Y., Papernot N., Anderson R. The Curse of Recursion: Training on Generated Data Makes Models Forget. [Electronic resource] – Access mode: https://arxiv.org/pdf/2305.17493, date of reference 15.04.2024.
- LeCun Y., Cortes C., Burges C.J. MNIST handwritten digit database В: ATT Labs. [Electronic resource] – Access mode: https://yann.lecun.com/exdb/mnist/, date of reference 15.06.2024.
- Kingma D.P., Welling M. Auto-Encoding Variational Bayes. [Electronic resource] – Access mode: https://arxiv.org/pdf/1312.6114, date of reference 15.04.2024.
- Gerstgrasser M., Schaeffer R., Dey A., Rafailov R., Pai D., Sleight H., Hughes J., Korbak T., Agrawal R., Gromov A., Roberts D.A., Yang D., Donoho D.L., KoyejoIs S. Model Collapse Inevitable? Breaking the Curse of Recursion by Accumulating Real and Synthetic Data. [Electronic resource] – Access mode: https://arxiv.org/html/2404.01413v2, date of reference 15.04.2024.
- Eldan R., Li Y. TinyStories: How Small Can Language Models Be and Still Speak Coherent English? [Electronic resource] – Access mode: https://arxiv.org/pdf/2305.07759, date of reference 15.04.2024.
- Xu M., Yu L., Song Y., Shi C., Ermon S., Tang J. GeoDiff: A Geometric Diffusion Model for Molecular Conformation Generation. [Electronic resource] – Access mode: https://arxiv.org/pdf/2203.02923, date of reference 15.04.2024.
- Axelrod S., Gomez-Bombarelli R. GEOM, energy-annotated molecular conformations for property prediction and molecular generation. Scientific Data. 2022. V. 9. № 1. P. 185. DOI 10.1038/s41597-022-01288-4.
- Liu Z., Luo P., Wang X., Tang X. Deep Learning Face Attributes in the Wild. IEEE International Conference on Computer Vision. 2015. P. 3730–3738. DOI 10.1109/ICCV.2015.425.
- Gillman N., Freeman M., Aggarwal D., Hsu C.-H., Luo C., Tian Y., Sun C. Self-Correcting Self-Consuming Loops for Generative Model Training. [Electronic resource] – Access mode: https://arxiv.org/pdf/2402.07087, date of reference 15.04.2024.
- Saunders W., Yeh C., Wu J., Bills S., Ouyang L., Ward J., Leike J. Self-critiquing models for assisting human evaluators. [Electronic resource] – Access mode: https://arxiv.org/pdf/2206.05802, date of reference 15.04.2024.
- Welleck S., Lu X., West P., Brahman F., Shen T., Khashabi D., Choi Y. Generating Sequences by Learning to Self-Correct. [Electronic resource] – Access mode: https://arxiv.org/pdf/2211.00053, date of reference 15.04.2024.
- Tevet G., Raab S., Gordon B., Shafir Y., Cohen-Or D., Bermano A.H. Human Motion Diffusion Model. [Electronic resource] – Access mode: https://arxiv.org/pdf/2209.14916, date of reference 15.04.2024.
- Ghorbani S., Mahdaviani K., Thaler A., Kording K., Cook D.Ja., Blohm G., Troje N.F. MoVi: A large multi-purpose human motion and video dataset. PLoS ONE. 2021. V. 16. № 6. P. e0253157. DOI 10.1371/journal.pone.0253157.
- Ho J., Jain A., Abbeel P. Denoising Diffusion Probabilistic Models. [Electronic resource] – Access mode: https://arxiv.org/pdf/2006. 11239, date of reference 15.04.2024.
- Luo Z., Hachiuma R., Yuan Y., Kitani K. Dynamics-Regulated Kinematic Policy for Egocentric Pose Estimation. [Electronic resource] – Access mode: https://arxiv.org/pdf/2106.05969, date of reference 15.04.2024.