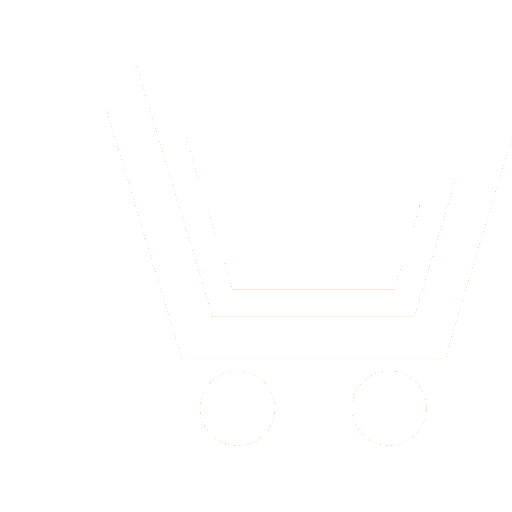
R.A. Kochkarov1, A.M. Poykalaynen2
1,2 Financial University under the Government of the Russian Federation (Moscow, Russia)
1 rkochkarov@fa.ru, 2 ampojkalajnen@fa.ru
Advances in artificial intelligence technologies offer new opportunities to improve medical practice. One of these opportunities is the use of large language models (LLM) in combination with machine translation (MT) technologies to solve problems related to medical documentation, search and summarization of medical information, and preparation of educational materials for medical professionals. The purpose of this work is to demonstrate the capabilities of the Biomistral model together with the Seamless M4T v2 machine translation model for processing medical texts in languages such as Chinese, Japanese, Korean and Arabic. The study conducted experiments using a corpus of texts collected from open inter-net sources such as PubMed. The results showed that the combination of LLM and machine translation models can significantly improve the quality of translation, summarization, and preparation of medical texts. The implementation of these technologies can significantly improve the efficiency and accessibility of medical services, especially in regions with multilingual populations, by improving the quality of medical documentation and educational materials for medical professionals.
Kochkarov R.A., Poykalaynen A.M. Application of large language models and machine translation technologies in health care. Neurocomputers. 2024. V. 26. № 6. Р. 31-38. DOI: https://doi.org/10.18127/j19998554-202406-05 (In Russian)
- Zhuleva S.Yu., Kroshilin A.V., Kroshilina S.V. Development of a decision support system for the organization of a medical worker's working time based on artificial intelligence methods. Biomedicine Radioengineering. 2023. V. 26. № 3. Р. 55–60. DOI 10.18127/ j15604136-202303-07. (In Russian)
- Brown T.B., Mann B., Ryder N., Subbiah M., Kaplan J., Dhariwal P., Neelakantan A., Shyam P., Sastry G., Askell A., Agarwal S., Herbert-Voss A., Krueger G., Henighan T., Child R., Ramesh A., Ziegler D.M., Wu J., Winter C., Hesse C., Chen M., Sigler E., Litwin M., Gray S., Chess B., Clark J., Berner C., McCandlish S., Radford A., Sutskever I., Amodei D. Language Models are Few-Shot Learners. [Electronic resource] – Access mode: https://arxiv.org/pdf/2005.14165, date of reference 21.06.24.
- Lee J., Yoon W., Kim S., Kim D., Kim S., So C.H., Kang J. BioBERT: a pre-trained biomedical language representation model for biomedical text mining. Bioinformatics. 2020. V. 36. № 4. P. 1234–1240. DOI 10.1093/bioinformatics/btz682.
- Biomistral: A Large Language Model for Biomedical Texts. Biomistral Documentation. [Electronic resource] – Access mode: https://bio-mistral.ai/documentation, date of reference 21.06.24.
- SeamlessM4T: Massively Multilingual & Multimodal Machine Translation. [Electronic resource] – Access mode: https://arxiv.org/pdf/ 2308.11596, date of reference 21.06.24.
- PubMed. "Home – PubMed – NCBI." National Center for Biotechnology Information. [Electronic resource] – Access mode: https://pub-med.ncbi.nlm.nih.gov/, date of reference 21.06.24.
- Papineni K., Roukos S., Ward T., Zhu W.-J. BLEU: a method for automatic evaluation of machine translation. Proceedings of the 40th Annual Meeting on Association for Computational Linguistics. 2002. P. 311–318. DOI 10.3115/1073083.1073135.
- Lin C.-Y. ROUGE: A Package for Automatic Evaluation of Summaries. Proceedings of the Workshop on Text Summarization Branches Out. 2004. P. 74–81.
- Lavie A., Agarwal A. METEOR: An Automatic Metric for MT Evaluation with High Levels of Correlation with Human Judgments. Proceedings of the Second Workshop on Statistical Machine Translation. 2007. P. 228–231.
- Liu Y., Ott M., Goyal N., Du J., Joshi M., Chen D., Levy O., Lewis M., Zettlemoyer L., Stoyanov V. RoBERTa: A Robustly Optimized BERT Pretraining Approach. [Electronic resource] – Access mode: https://arxiv.org/pdf/1907.11692, date of reference 21.06.24.
- Yang Z., Dai Z., Yang Y., Carbonell J., Salakhutdinov R., Le Q.V. XLNet: Generalized Autoregressive Pretraining for Language Understanding. [Electronic resource] – Access mode: https://arxiv.org/pdf/1906.08237, date of reference 21.06.24.
- Bharati S., Mondal M.R.H., Podder P., Surya Prasath V.B. Federated learning: Applications, challenges and future directions. [Electronic resource] – Access mode: https://arxiv.org/pdf/2205.09513, date of reference 21.06.24.
- Abadi M., Chu A., Goodfellow I., McMahan H.B., Mironov I., Talwar K., Zhang L. Deep Learning with Differential Privacy. [Electronic resource] – Access mode: https://arxiv.org/pdf/1607.00133, date of reference 21.06.24.