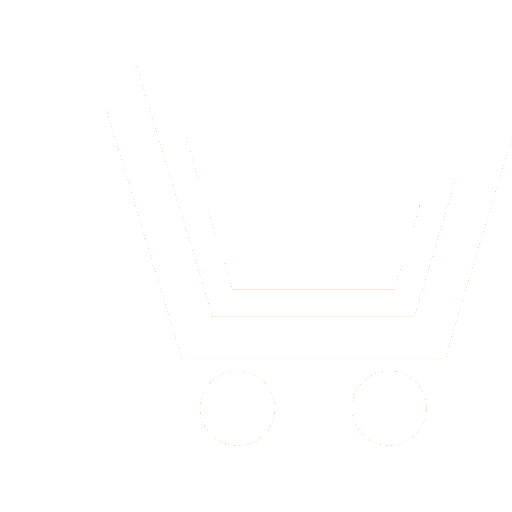
G.G. Bizhanova1, Z.A. Bashirova2, M.V. Ivanova3, G.G. Zaripova4, I.A. Gareev5, L.D. Sharipova6, A.V. Sidelnikov7
1–7 Ufa University of Science and Technology (Ufa, Russia)
1 bizhanova111@mail.ru
One of the problems of multisensor systems is the uncontrolled shift in sensor responses over time during continuous electrode operation, which leads to systematic errors in recognizing multicomponent solutions. During prolonged operation of multisensor electrochemical systems, there is a temporal drift of sensor signals, which makes it difficult to accurately recognize analyzed multicomponent objects and leads to identification errors.
Goal – development and approbation of a new voltammetric method for recognizing food products using a two-sensor system, which allows solving the problem of temporal drift of signals and improving the accuracy of sample identification during prolonged operation of multisensor systems.
A two-sensor system of copper/aluminum microelectrodes is proposed for a new voltammetric method of recognizing food products, using apples from different manufacturers as an example, based on voltammetric time series data. The electrochemical responses of the voltammetric cell during the analysis of apples in the temporal mode were studied by the principal component method to identify characteristic «fingerprint» signals. It was found that the temporal responses of the sensors during contact with different parts of the apples (from the core to the peel) differ due to variations in chemical composition in this direction. At least 9 temporal characteristic signals were found and quantitatively described for each apple variety, forming a mathematical image of the variety/origin, invariant over time. The proposed approach solves the problem of uncontrolled temporal drift of sensor responses during prolonged operation, which causes systematic errors in the recognition of multicomponent solutions. The proposed solutions can improve the analytical characteristics of rapid control methods for the quality of food raw materials using voltammetric «electronic tongues/noses».
The practical significance lies in the possibility of increasing the accuracy and reproducibility of the results of rapid quality analysis of food products by eliminating the temporal drift of sensor signals and forming stable «fingerprints» for object identification.
Bizhanova G.G., Bashirova Z.A., Ivanova M.V., Zaripova G.G., Gareev I.A., Sharipova L.D., Sidelnikov A.V. Improving electrochemical approaches to ensure food safety and quality using temporal machine learning techniques in the development of user interfaces. Neurocomputers. 2024. V. 26. № 5. Р. 5-16. DOI: https://doi.org/10.18127/j19998554-202405-01 (In Russian)
- Winquist F., Wide P., Lundstrom I. An electronic tongue based on voltammetry. Analytica Chimica Acta. 1997. V. 357. № 1-2. P. 21–31. DOI 10.1016/S0003-2670(97)00498-4.
- Campos I., Alcañiz M., Aguado D., Barat R., Ferrer J., Gil L., Marrakchi M., Martínez-Máñez R., Soto J., Vivancos J.L. A voltammetric electronic tongue as tool for water quality monitoring in wastewater treatment plants. Water Research. 2012. V. 46. P. 2605–2614. DOI 10.1016/j.watres.2012.02.029.
- Hayashi N., Ujihara T., Chen R., Irie K., Ikezaki H. Objective evaluation methods for the bitter and astringent taste intensities of black and oolong teas by a taste sensor. Food Research International. 2013. V. 53. № 2. P. 816–821. DOI 10.1016/j.foodres.2013.01.017.
- Maksutova E.I., Bizhanova G.G., Ivanova M.V., Syakaev R.S., Sidelnikov A.V. Film sensors based on PANI and their composite modifications in the electrochemical analysis of some ecotoxicants. Materials of the XV International Scientific and Practical Conference "Actual problems and prospects for the development of world science and technology: the state, problems and solutions". 2023. P. 197–202. (In Russian)
- Grigoriev Yu.A. Technologies of analytical processing of big data. Information-measuring and control systems. 2016. V. 14. № 12. P. 59–68. (In Russian)
- Rodionova O.E., Pomerantsev A.L. Chemometrics in analytical chemistry. [Electronic resource] – Access mode: https://dalab.unn.ru/Si-tePCAnarod/chemometrics_review.pdf, date of reference 17.01.2024. (In Russian)
- Esbensen K. Analysis of multidimensional data. Selected chapters. Translated from the English by S.V. Kucheryavsky, edited by O.E. Rodionova. Kazan: KazGASU. 2008. 158 p. (In Russian)
- Sidel'nikov A., Bikmeev D., Kudasheva F., Maystrenko V. Voltammetric identification of motor oils using electronic tongue on carbon-paste «electrode basis». Journal of Analytical Chemistry. 2013. V. 68. № 2. P. 140–147. DOI 10.1134/S1061934813020159.
- Holmin S., Krantz-Rülcker C., Lundström I., Winquist F. Drift correction of electronic tongue responses. Measurement Science and Technology. 2001. V. 12. № 8. P. 1348–1354. DOI 10.1088/0957-0233/12/8/350.
- Sinha S., Bhardwaj R., Sahu N., Ahuja H., Sharma R., Mukhiya R. Temperature and temporal drift compensation for Al2O3-gate ISFET-based pH sensor using machine learning techniques. Microelectronics Journal. 2020. V. 97. P. 104710. DOI 10.1016/j.mejo.2020. 104710.