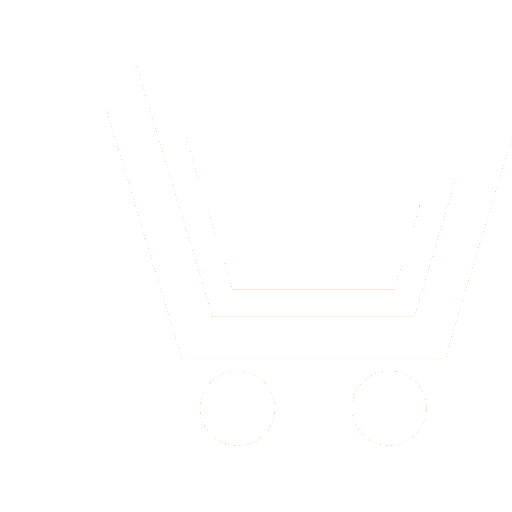
A.V. Arkhipov1, K.A. Muravyov2, A.A. Solodnjakov3, I.S. Potanin4
1–4 Bauman Moscow State Technical University (Moscow, Russia)
2 muravyov@bmstu.ru
Problem setting. The production process of any electronic device currently involves a large number of both software and hardware. In the manufacture of electronic products, elements are automatically installed on the board using robotic devices. Along with the installation of components, the quality of the PCB design is also of serious importance. Quality control at any stage of production is assigned to a person, which significantly reduces not only productivity, but also the quality of rejection. All this leads to the need to develop modern digital methods and technologies for automatic control of components of electronic products using artificial intelligence.
Target. The purpose of this work is to study methods for identifying defective areas of a printed circuit board topology that arise during its production, as well as the possibility of adjusting production line control algorithms.
Results. An analysis of modern literary and Internet sources was carried out, during which the advantages and disadvantages of using neural networks in the design of printed circuit boards were determined; an algorithm was also proposed for developing a technique for monitoring the topology of a printed circuit board; a program for applying the image analysis procedure was implemented and the software package was tested. As part of the work, it is proposed to monitor the line in real time, which makes it possible to adjust the indicators “online” by entering possible causes into the database, subsequent analysis and elimination.
Practical significance. The proposed solution will primarily improve the quality and increase the speed of modern printed circuit board production. The main indicator that it can provide is a reduction in the defect rate.
Arkhipov. А.V., Muravyov K.A., Solodnjakov A.A., Potanin I.S. The use of neural network technologies in the design of printed circuit boards. Neurocomputers. 2024. V. 26. № 3. Р. 45-54. DOI: https://doi.org/10.18127/j19998554-202403-05 (In Russian)
- Romashchenko M.A., Vasilchenko D.V., Rojnenko S.N. Development of a method for assessing the influence of electromagnetic interference on the functioning of electronic means in the process of their design. Radiotekhnika. 2021. V. 85. № 6. P. 57−61. DOI 10.18127/j00338486-202106-10 (In Russian)
- Romashchenko M.A., Vasilchenko D.V., Pukhov D.A. Using neural network algorithms for visual control of the topology of printed circuit boards. Bulletin of the Voronezh State Technical University. 2022. V. 18. № 3. P. 78–82. DOI 10.36622/VSTU.2022.18.3.010. (In Russian)
- Zaitsev P.A., Suraev A.I., Korotin T.A. Neural networks application in the design of printed circuit boards. Materials of the 59th Scientific Conference graduate students, undergraduates and students of BSUIR "Electronic systems and Technologies". Minsk: Scientific electronic publication. 2023. P. 139–141. (In Russian)
- Khomutskaya O.V., Kopylov D.A., Karpunova A.S. Analysis of PCB deformation using neural networks. Abstracts of the XXXI International Scientific and Technical Conference. "Modern technologies in the tasks of control, automation and information processing." Moscow: Moscow Aviation Institute (National Research University). 2022. p. 100. (In Russian)
- Cheremisin D.G., Mkrtchyan V.R. Application of neural networks in the production of printed circuit boards. Symbol of Science: international scientific journal. 2023. № 6-2. P.32–33. (In Russian)
- Velichkin V.A., Shikolenko I.A. Research and development of algorithms for visual assessment of malfunction of electronic circuits using artificial intelligence technologies. Original research. 2020. V.10. № 6. P. 47–52. (In Russian)
- The use of artificial intelligence to improve printed circuit boards. [Electronic resource] – Access mode: https://ai-news.ru/2022/10/primenenie_iskusstvennogo_intellekta_dlya_usovershenstvovaniya_pechatny.html, date of reference 23.10.2023. (In Russian)
- Neural networks: what they are and how they work. [Electronic resource]. – Access mode: https://dzen.ru/a/ZBs8kNkQFhyfcqvq, date of reference 09.10.2023. (In Russian)
- Buyanov A.I., Vlasov A.I., Zagoskin A.V. Application of neural network methods at defectoscopy of printed-circuit boards. Neurocomputers: development, application. 2002. № 3. P. 42–70 (In Russian)
- Vetoshkin N.V. Application of neural networks of object detection to detect visual defects of printed circuit boards of smart home systems. Collection of reports of scientific and technical conference on the results of research works of students of the Institute of Economics, Management and Information Systems in Construction and Real estate of the National Research University MGSU. 2020. pp.453–457. (In Russian)
- Viryasova A.Yu., Vlasov A.I., Gladkikh A.A. Neural network methods for integral structure non-destructive testing. Neurocomputers: development, application 2019. № 2. P. 54–67. DOI 10.18127/j19998554-201902-06. (In Russian)
- Vlasov A.I., Zavyalov N.V., Selivanov K.V., Skalchenkov I.I. Application of neural networks in detecting PCB defects. Neurocomputers. 2022. V. 24. № 6. Р. 5–19. DOI 10.18127/j19998554-202206-01. (In Russian)
- Bragina A.D., Gromov M.L. Automation system for tracing printed circuit boards based on neural networks. Materials of the 13th International Conference "New information technologies in the study of complex structures". Tomsk: National Research Tomsk State University. 2020. P. 79–80. (In Russian)
- Eremeev I.Yu., Neretina K.A., Pechurin V.V. Investigation of the possibility of using the YOLOv5 convolutional neural network in radio monitoring complexes for detecting OFDM signals. Electromagnetic waves and electronic systems. 2023. V. 28. № 3. P. 18−27. DOI 10.18127/j15604128-202303-03. (in Russian)