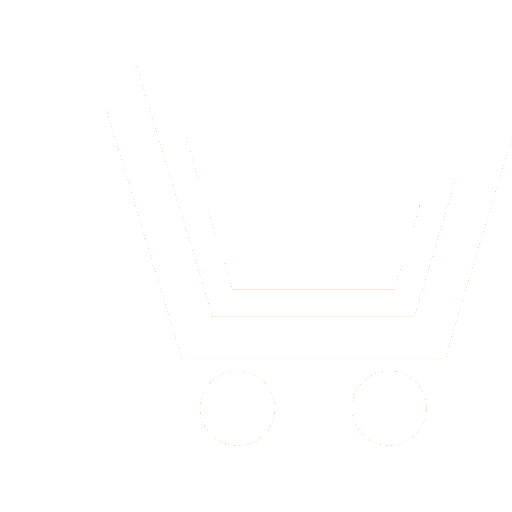
S.V. Prokopchina1, P.E. Ryabov2, E.Yu. Shchetinin3
1–3 Financial University under the Government of the Russian Federation (Moscow, Russia)
1 svprokopchina@fa.ru, 2 peryabov@fa.ru, 3 eyshchetinin@fa.ru
Machine learning is an essential component of the development of intelligent systems for various purposes. This article proposes a variant of machine learning for intelligent systems based on a regularizing Bayesian approach. The modes of learning in information processing in conditions of significant information uncertainty are considered. To form data sets, data and knowledge integration technologies are used to improve the efficiency of the systems and the quality of the solutions obtained.
Prokopchina S.V., Ryabov P.E., Shchetinin E.Yu. Machine learning of a convolutional neural network based on a regularizing Bayesian approach. Neurocomputers. 2024. V. 26. № 3. Р. 36-44 DOI: https://doi.org/10.18127/j19998554-202403-04 (In Russian)
- Wolf A. The Machine Learning Simplified: A Gentle Introduction to Supervised Learning. Leanpub. 2022. 111 p.
- Burkov A.A. Machine learning without unnecessary words. St. Petersburg: Peter. 2020. 192 p. (In Russian)
- Khamchichev G.A., Kozhomberdieva G.I. On setting up and learning possibilities of a neuro-fuzzy network based on a Bayesian logical-probabilistic model. Proceedings of the International Conference on Soft Computing and Measurements. 2021. P. 27–35. (In Russian)
- Kozhomberdieva G.I., Burakov D.P. Combining Bayesian and logical-probabilistic approaches for fuzzy inference systems implementation. Journal of Physics: Conference Series. 2020. V. 1703. P. 012042. DOI 10.1088/1742-6596/1703/1/012042.
- Prokopchina S.V. Intellectual measurements based on the regularizing Bayesian approach. M.: Scientific Library. 2021. 499 p. (In Russian)
- Prokopchina S.V. Methodological foundations for the creation of regularizing artificial intelligence methods to ensure the sustainability of solutions. Soft Measurements and Computing. 2023. V. 68. № 7-1. P. 5–23. DOI 10.36871/2618-9976.2023.07.001. (In Russian)
- Prokopchina S.V. Fundamentals of the theory of scaling in economics. M.: Scientific Library. 2021. 299 p. (In Russian)
- Milov V.R., Baranov V.G., Malyugin S.A. Bayesian methods of training neural networks. Neurocomputers. 2007. № 11. P. 14–19. (In Russian)