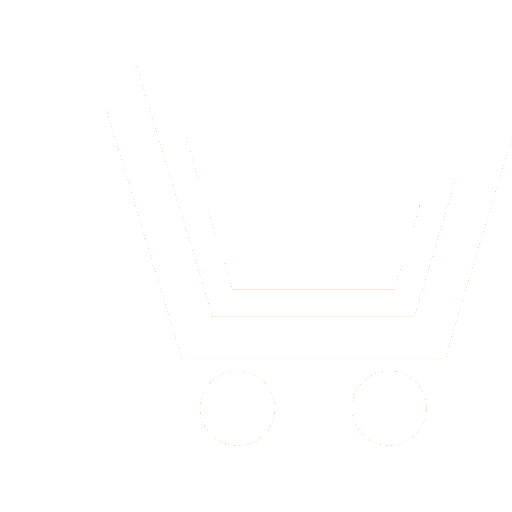
A.N. Alyunov1
1 Financial University under the Government of the Russian Federation (Moscow, Russia)
Problem setting. A large number of problems of analyzing the state of power transformers at electrical substations are solved on the basis of mathematical models, the validity of which is indisputable. The disadvantage of standard diagnostic methods for current-carrying parts of transformers is the requirement to remove voltage and disconnect from the electrical network. Currently used diagnostic methods without stress relief require improvement in terms of improving accuracy, speed and providing predictive response. In addition, the use of direct solution methods in the diagnostic process leads to errors in assessing the state of the transformer, since many influencing operational factors are not taken into account. In particular, the existing methods do not provide for the influence of the service life of a power transformer on its electrical characteristics. Consequently, the development of algorithms for identifying parameters of mathematical models of power transformers is required, while the use of machine learning methods to solve the problem under consideration will reduce the impact of these disadvantages of known solutions. Machine learning can identify and take into account the laws unaccounted for by the mathematical model of the transformer.
Target. To develop a method for diagnosing electrical parts of power transformers using machine learning methods and tools.
Results. It is proposed to use a machine learning model based on the "random forest" algorithm for operational diagnostics of power transformers in electrical networks. The data obtained in real time on the electrical operating modes of a power transformer is used to determine the parameters of the electrical parts of transformers. To assess the current diagnostic condition of the transformer, the results obtained are compared with the specified reference values.
Practical significance. The proposed approach makes it possible to obtain estimates of the state of the transformer with high accuracy – the approximate error is 0.5% for the resistances included in the mathematical model of a serviceable power transformer.
Alyunov A.N. Diagnostics of power transformers electrical parts using machine learning methods. Neurocomputers. 2023. V. 25. № 5.
Р. 23-33. DOI: https://doi.org/10.18127/j19998554-202305-04 (In Russian)
- Kolesnikov I.E., Korzhov A.V., Gorshkov K.E. Digital Program for Diagnosing the Status of a Power Transformer. Global Smart Industry Conference. Chelyabinsk, Russia. 2020. P. 315–321. DOI 10.1109/GloSIC50886.2020.9267867.
- Wang G., Liu Y., Chen X., Sui H., Ma C., Zhang J., Liu Y. Power transformer fault diagnosis system based on Internet of Things. Eurasip Journal on Wireless Communications and Networking. 2021. V. 2021. № 1. DOI 10.1186/s13638-020-01871-6.
- Tahir M., Tenbohlen S. Transformer Winding Fault Classification and Condition Assessment Based on Random Forest Using FRA. Energies. 2023. V. 16. № 9. P. 3714. DOI 10.3390/en16093714.
- Vita V., Fotis G., Chobanov V., Pavlatos C., Mladenov V. Predictive Maintenance for Distribution System Operators in Increasing Transformers’ Reliability. Electronics. 2023. V. 12. № 6. P. 1356. DOI 10.3390/electronics12061356.
- Zhang D., Chu Z., Gui Q., Wu F., Yang H., Ma Y., Tao W. Transformer maintenance decision based on condition monitoring and fuzzy probability hybrid reliability assessment. IET Generation, Transmission and Distribution. 2023. V. 17. № 1. P. 976–992. DOI 10.1049/gtd2.12718.
- Esmaeili Nezhad A., Samimi M. A review of the applications of machine learning in the condition monitoring of transformers. Energy Systems. 2022. DOI 10.1007/s12667-022-00532-5.
- Khalyasmaa A.I., Matrenin P.V., Eroshenko S.A., Manusov V.Z., Bramm A.M., Romanov A.M. Data Mining Applied to Decision Support Systems for Power Transformers’ Health Diagnostics. Mathematics. 2022. V. 10. № 14. P. 2486. DOI 10.3390/math10142486.
- Aizpurua J.I., McArthur S.D.J., Stewart B.G., Lambert B., Cross J.G., Catterson V.M. Adaptive Power Transformer Lifetime Predictions Through Machine Learning and Uncertainty Modeling in Nuclear Power Plants. IEEE Transactions on Industrial Electronics. 2019. V. 66. № 6. P. 4726–4737. DOI 10.1109/TIE.2018.2860532.
- Levin V.M., Yahya A.A. Support for Decision-Making to Ensure Reliable Operation of Transformers as Part of a Responsible Power Facility. International Multi-Conference on Industrial Engineering and Modern Technologies. Vladivostok, Russia. 2020. P. 1–6. DOI 10.1109/FarEastCon50210.2020.9271626.
- Ab Ghani S., Khiar M.S.A., Chairul I.S., Rahim N.H., Kamaruzaini M.H. Comparative study of electrical test methods on detecting transformer faults. Indonesian Journal of Electrical Engineering and Computer Science. 2022. V. 25. № 2. P. 755–762. DOI 10.11591/ijeecs.v25.i2.pp755-762.
- Suo C, Ren Y, Zhang W, Li Y, Wang Y, Ke Y. Evaluation Method for Winding Performance of Distribution Transformer. Energies. 2021. V. 14. № 18. P. 5832. DOI 10.3390/en14185832.
- Chernomordov S.V., Druzhinina O.V., Masina O.N., Petrov A.A. Application of machine learning methods in problems of neural network modeling of controlled technical systems. Neurocomputers. 2022. V. 24. № 1. Р. 25–35. DOI 10.18127/j19998554-202201-03.
(In Russian) - Lu W., Shi C., Fu H., Xu Y. Fault Diagnosis Method for Power Transformers Based on Improved Golden Jackal Optimization Algorithm and Random Configuration Network. IEEE Access. 2023. V. 11. P. 35336–35351. DOI 10.1109/ACCESS.2023.3265469.
- Elahi O., Behkam R., Gharehpetian G.B., Mohammadi F. Diagnosing Disk-Space Variation in Distribution Power Transformer Windings Using Group Method of Data Handling Artificial Neural Networks. Energies. 2022. V. 15. № 23. P. 8885. DOI 10.3390/en15238885.
- Andreeva O.N., Novikov E.S. Models and methods of monitoring and predictive management of technogenic risks at electronic industry enterprises. Science Intensive Technologies. 2019. V. 20. № 1. P. 47–54. DOI 10.18127/j19998465-201901-06. (In Russian)
- Smerdin A., Ermachkov G., Nezevak V., Sidorov O., Golubkov A. Use of sensor networking technology to build a power transformer monitoring system. E3S Web of Conferences. 2020. V. 224. № 02021. P. 11. DOI 10.1051/e3sconf/202022402021.
- Biçen Y. An Internet of Things (IoT) based Monitoring System for Oil-immersed Transformers. Balkan Journal of Electrical and Computer Engineering. 2019. V. 7. № 3. P. 226–234. DOI 10.17694/bajece.524921.
- Ecaterina M., Ion S. Parameter Identification for Two-Windings Power Transformers. International Conference on Electromechanical and Energy Systems. Craiova, Romania. 2019. P. 1–6. DOI 10.1109/SIELMEN.2019.8905914.
- Silva A.F., Silveira E.G., Alipio R. Artificial Neural Network Applied to Differential Protection of Power Transformers. Journal of Control, Automation and Electrical Systems. 2022. V. 33. № 3. P. 850–857. DOI 10.1007/s40313-021-00845-3.
- Nassim A., Khalid Y. Simulink model of transformer differential protection using phase angle difference based algorithm. International Journal of Power Electronics and Drive Systems. 2020. V. 11. № 2. P. 1088–1098. DOI 10.11591/ijpeds.v11.i2.pp1088-1098.
- Mobeen F., Ahmad S., Shoukat A., Batool S. A Generalized Modeling and Analysis for Transformer Parameterization. 16th International Conference on Emerging Technologies. Islamabad, Pakistan. 2021. P. 1–7. DOI 10.1109/ICET54505.2021.9689869.
- Arias Velásquez R.M., Mejía Lara J.V. Root Cause Analysis Improved with Machine Learning for Failure Analysis in Power Transformers. Engineering Failure Analysis. 2020. V. 115. P. 104684. DOI 10.1016/j.engfailanal.2020.104684.
- Zhou L., Hu T. Multifactorial Condition Assessment for Power Transformers. IET Generation, Transmission & Distribution. 2020. V. 14. № 9. P. 1607–1615. DOI 10.1049/iet-gtd.2019.0727.
- Yan C., Li M., Liu W. Transformer Fault Diagnosis Based on BP-Adaboost and PNN Series Connection. Mathematical Problems in Engineering. 2019. V. 2019. P. 1019845. DOI 10.1155/2019/1019845.
- Wu Y., Gu L., Zhang X., Wang J. An On-Line Identification Method for Short-Circuit Impedance of Transformer Winding Based on Sudden Short Circuit Test. Frontier Computing. 2019. V. 542. P. 1365–1375. DOI 10.1007/978-981-13-3648-5_176.
- Tian F., Jing Z., Zhao H., Zhang E., Liu J. A Synthetic Condition Assessment Model for Power Transformers Using the Fuzzy Evidence Fusion Method. Energies. 2019. V. 12. № 5. P. 857. DOI 10.3390/en12050857.
- Tan G., Liu D., Shi Y., Yang, Z. Condition Assessment Method for Power Transformers Based on Variable Weight Principle and Fuzzy Comprehensive Evaluation. 7th International Forum on Electrical Engineering and Automation. Hefei, China. 2020. P. 883–888. DOI 10.1109/ifeea51475.2020.00185.
- Rexhepi V., Nakov P. Condition Assessment of Power Transformers Status Based on Moisture Level Using Fuzzy Logic Techniques. Journal of Mechatronics, Electrical Power, and Vehicular Technology. 2018. V. 9. № 1. P. 17–24. DOI 10.14203/j.mev. 2018.v9.17-24.
- Lin Z., Tang S., Peng G., Zhang Y., Zhong Z. An Artificial Neural Network Model with Yager Composition Theory for Transformer State Assessment. 2nd Advanced Information Technology, Electronic & Automation Control Conference. Chongqing, China. 2017. P. 652–655. DOI 10.1109/iaeac.2017.8054097.
- Khalyasmaa A., Eroshenko S.A., Tashchilin V., Seguin C., Ehlinger L., Vibhute R.R., Atluri S.R. Machine Learning Algorithms for Power Transformers Technical State Assessment. International Multi-Conference on Engineering, Computer and Information Sciences. Novosibirsk. 2019. P. 601–606. DOI 10.1109/SIBIRCON48586.2019.8958395.
- Kari T., Gao W., Zhao D., Abiderexiti K., Mo W., Wang Y. Hybrid Feature Selection Approach for Power Transformer Fault Diagnosis Based on Support Vector Machine and Genetic Algorithm. IET Generation, Transmission and Distribution. 2018. V. 12. № 21. P. 5672–5680. DOI 10.1049/iet-gtd.2018.5482.
- Schäfer F. Multi-Year Time-Series-Based power system planning with hybrid optimization and supervised learning methods. Kassel: Kassel University Press. 2021. DOI 10.17170/kobra-202101213009.