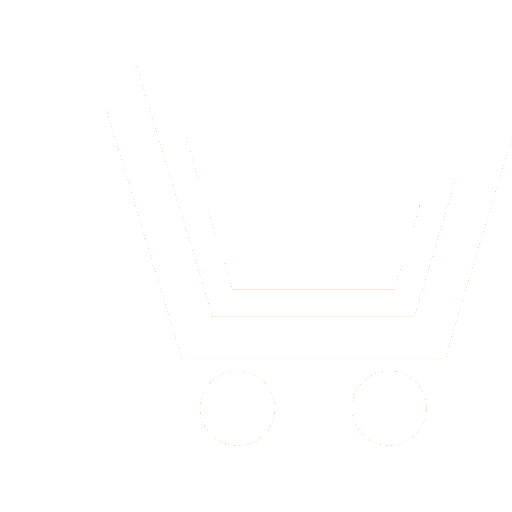
N.A. Andriyanov1, Ya.V. Kulichenko2
1,2 Financial University under the Government of the Russian Federation (Moscow, Russia)
Problem setting. Currently, there is a growing interest in the problem of face detection and recognition. On the one hand, researchers strive to make algorithms more and more accurate, on the other hand, more and more fast. At the same time, in recent years, the level of development of generative models has reached the point that computers are able to generate images that are almost impossible to distinguish from real ones. And when training identifiers of persons, there are difficulties in the legal sphere.
Target. The main purpose of this study is to demonstrate the possibility of developing algorithms for processing biometric information based on synthetic data. This includes tasks such as face detection and face recognition.
Results. The article considers the main types of generative image models. Variants of simulated images of human faces are presented. Further, on the prepared artificial base, the Viola-Jones detector and the YuNet networks were trained. At the same time, the accuracy metrics of the detectors exceed 90%.
Practical significance. The developed approaches to the generation of face images will allow improving and modifying solutions in the field of face identification systems, face detection, and highlighting key points. All this has not only a potential scientific novelty, but also a high practical significance for access control and management systems.
Andriyanov N.A., Kulichenko Ya.V. Applying generative image models to augment face detector training data. Neurocomputers. 2023. V. 25. № 4. Р. 7-15. DOI: https://doi.org/10.18127/j19998554-202305-02 (In Russian)
- Andriyanov N.A., Dementev V.E., Vasiliev K.K., Tashlinskiy A.G. Investigation of Methods for Increasing the Efficiency of Convolutional Neural Networks in Identifying Tennis Players. Pattern Recognition and Image Analysis. 2021. V. 31. № 3. P. 496–505. DOI 10.1134/S1054661821030032.
- Sanchez-Moreno A.S., Olivares-Mercado J., Hernandez-Suarez A., Toscano-Medina K., Sanchez-Perez G., Benitez-Garcia G. Efficient Face Recognition System for Operating in Unconstrained Environments. Journal of imaging. 2021. V. 7. № 9. P. 161. DOI 10.3390/jimaging7090161.
- Kortli Y., Jridi M., Al Falou A., Atri M. Face Recognition Systems: A Survey. Sensors. 2020. V. 20. № 2. P. 342. DOI 10.3390/s20020342.
- Abdulaev B.K., Vlasov A.I., Fatkhutdinov T.M. Automated neural network system for analyzing the emotional state of a per-son-operator. Neurocomputers. 2023. V. 25. № 2. Р. 41–57. DOI 10.18127/j19998554-202302-04 (In Russian)
- Andriyanov N.A. Doubly stochastic autoregressive image models: dis. ... candidate of Technical Sciences. Ulyanovsk State University. Ulyanovsk. 2017. 183 p. (in Russian)
- Vasiliev K.K., Dementiev V.E., Andrianov N.A. Analysis of the effectiveness of estimating the changing parameters of a doubly stochastic model. Radio Engineering. 2015. № 6. P. 12–15. (in Russian)
- Buslaev A., Iglovikov V.I., Khvedchenya E., Parinov A., Druzhinin M., Kalinin A.A. Albumentations: Fast and Flexible Image Augmentations. Information. 2020. V. 11. P. 125. DOI 10.3390/info11020125.
- Andriyanov N.A., Andriyanov D.A. The using of data augmentation in machine learning in image processing tasks in the face of data scarcity. Journal of Physics: Conference Series. 2020. V. 1661. P. 012018. DOI 10.1088/1742-6596/1661/1/012018.
- Toma R.N., Piltan F., Kim J.-M. A Deep Autoencoder-Based Convolution Neural Network Framework for Bearing Fault Classification in Induction Motors. Sensors 2021. V. 21. № 24. P. 8453. DOI 10.3390/s21248453.
- Kingma D.P., Welling M. An Introduction to Variational Autoencoders. ArXiv. 2019. DOI 10.1561/2200000056.
- Goodfellow I., Pouget-Abadie J., Mirza M., Xu B., Warde-Farley D., Ozair S., Courville A., Bengio Y. Generative Adversarial Networks. ArXiv. DOI 10.48550/arXiv.1406.2661
- Akhmad A., Andriyanov N.A., Soloviev V.I., Solomatin D.A. Application of deep learning for augmentation and generation of an underwater data set with industrial objects. Bulletin of the South Ural State University. Series: Computer technology, control, radio electronics. 2023. V. 23. № 2. P. 5–16. (in Russian)
- Labelled Faces in the Wild (LFW) Dataset. [Electronic resource] – Access mode: https://www.kaggle.com/datasets/jessicali9530/lfw-dataset, date of reference 15.06.2023.
- Xiang J., Zhu G. Joint Face Detection and Facial Expression Recognition with MTCNN. 4th International Conference on Information Science and Control Engineering. Changsha, China. 2017. P. 424–427. DOI 10.1109/ICISCE.2017.95.
- Wu W., Peng H., Yu S. YuNet: A Tiny Millisecond-level Face Detector. Machine Intelligence Research. 2023. DOI 10.1007/s11633-023-1423-y.