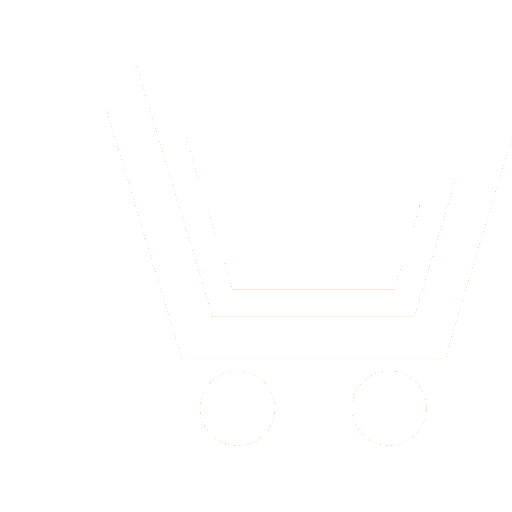
V.P. Zhalnin1, V. Aiguzhin2, M.E. Apakov3, A.M. Panfilkin4
1–4 Bauman Moscow State Technical University (Moscow, Russia)
This paper is devoted to the analysis of memristive technologies for the implementation of neural network element base. Modern trends are characterized by the fact that the speed of processors exceeds the speed of memory by several orders of magnitude, and the amount of information for processing is only growing, the process of data transfer between computing units and memory become a major stoppers and "bottle-neck" in various computing systems. Recent developments in the field of processing-in-memory (PIM) present promising solutions to problems, in particular related to the field of machine learning. Memristive-based implementations of neural network components can provide energy-efficient neuromorphic computing due to their synaptic behavior. This paper discusses the design of various memristor-based neural networking system architectures. Potential applications and perspectives of a memristor-based neural network system are discussed.
Target is an analysis of the prospects for the application of memristive technologies in the field of neural networks, as well as a review of current practical solutions based on memristors using the PIM approach.
The tendency in the field of neural network development, as well as hardware concepts and physical capabilities of memristor-based neurocomputing systems are considered. It is shown that at the moment memristor technologies are suitable for the creation of competitive systems on the example of specific modern solutions. A comparison of the considered architectural implementations with traditional solutions is made. Recommendations on the prospects for further development of memristor-based neurocomputing are given.
The results of the work can be used to create different types of computing systems and build architectural solutions based on memristor element base. These examples of basic, and not only basic, structures and solutions based on memristors can serve as a basis for future promising developments, which can be presented to the consumer market of neuromorphic computing complexes of domestic design.
Zhalnin V.P., Aiguzhin V., Apakov M.E., Panfilkin A.M. Memristive technologies for the implementation of a neural network element base. Neurocomputers. 2022. V. 24. № 6. Р. 53-66. DOI: https://doi.org/10.18127/j19998554-202206-06 (In Russian).
- Rubakov S.V. Sovremennyye metody analiza dannykh. Nauka. Innovatsii. Obrazovaniye. 2008. T. 3. № 4. S. 165–176. (in Russian).
- Gafarov F.M., Galimyanov A.F. Iskusstvennyye neyronnyye seti i prilozheniya: Ucheb. posobiye. Kazan: Izd-vo Kazan. un-ta. 2018. 121 s. (in Russian).
- He K., Zhang X., Ren S., Sun J. Deep residual learning for image recognition. Proceedings of the IEEE Computer Society Conference on Computer Vision and Pattern Recognition. 2016. Article № 7780459. P. 770–778.
- Krizhevsky A., Sutskever I., Hinton G.E. ImageNet classification with deep convolutional neural networks. Advances in Neural Information Processing Systems. 2012. № 2. P. 1097–1105.
- Girshic R., Donahue J., Darrell T., Malik J. Rich feature hierarchies for accurate object detection and semantic segmentation. Proceedings of the IEEE Computer Society Conference on Computer Vision and Pattern Recognition. 2014. Article № 6909475. P. 580–587.
- Kourou K., Exarchos T.P., Exarchos K.P., Karamouzis M.V., Fotiadis D.I. Machine learning applications in cancer prognosis and prediction. Computational and Structural Biotechnology Journal. 2015. V. 13. P. 8–17.
- Ismael A.M., Şengür A. Deep learning approaches for COVID-19 detection based on chest X-ray images. Expert Systems with Applications. 2021. V. 164. Article № 114054.
- Murphy E.A., Ehrhardt B., Gregson C.L. et al. Machine learning outperforms clinical experts in classification of hip fractures. Sci. Rep. 12. 2022. V. 2058. https://doi.org/10.1038/s41598-022-06018-9
- Fischer T., Krauss C. Deep learning with long short-term memory networks for financial market predictions. European Journal of Operational Research. 2018. V. 270 (2). P. 654–669.
- Chong E., Han C., Park F.C. Deep learning networks for stock market analysis and prediction: Methodology, data representations, and case studies. Expert Systems with Applications. 2017. V. 83. P. 187–205.
- Maier H.R., Dandy G.C. Neural networks for the prediction and forecasting of water resources variables: A review of modelling issues and applications. Environmental Modelling and Software. 2000. V. 15 (1). P. 101–124.
- Voyant C., Notton G., Kalogirou S., Nivet M.-L., Paoli C., Motte F., Fouilloy A. Machine learning methods for solar radiation forecasting. A review Renewable Energy. 2017. V. 105. P. 569–582.
- Biryukov G.I., Zhalnin V.P., Laptev D.V., Repnikov P.O. Osobennosti realizatsii svertochnykh neyronnykh setey na programmiruyemoy logicheskoy integralnoy skheme ARTIX-7. Neyrokompyutery: razrabotka. primeneniye. 2020. T. 22. № 3. S. 26–35. (in Russian).
- Shakhnov V.A., Vlasov A.I., Polyakov Yu.A., Kuznetsov A.S. Neyrokompyutery: arkhitektura i skhemotekhnika. M.: Izd-vo Mashinostroyeniye. 2000. Ser. 9 Prilozheniye k zhurnalu "Informatsionnyye tekhnologii". 24 s. (in Russian).
- Vlasov A.I. Apparatnaya realizatsiya neyrovychislitelnykh upravlyayushchikh sistem. Pribory i sistemy. Upravleniye. kontrol. diagnostika. 1999. № 2. S. 61–65. (in Russian).
- Vlasov A.I., Zhalnin V.P., Shakhnov V.A., Alyabyev I.O. Vozmozhnosti primeneniya perspektivnoy neyrosetevoy elementnoy bazy na osnove neorganicheskikh memristorov. Neyrokompyutery i ikh primeneniye. XVII Vserossiyskaya nauchnaya konferentsiya. Tezisy dokladov. 2019. S. 242–245. (in Russian).
- Patent USA № 10373051 from 20 october, 2015. Resistive processing unit.
- Li C., Hu M., Li Y., Jiang H., Ge N., Montgomery E., Zhang J., Song W., Dávila N., Graves C. E., Li Z., Strachan J. P., Lin P., Wang Z., Barnell M., Wu Q., Williams R. S., Yang J. J., Xia Q. Analogue signal and image processing with large memristor crossbars. Nature Electronics. 2018. V. 1. № 1. P. 52–59.
- Hu M., Graves C.E., Li C., Li Y., Ge N., Montgomery E., Dávila N., Jiang H., Williams R. S., Yang J.J., Xia O., Strachan J.P. Memristor−based analog computation and neural network classification with a dot product engine. Advanced Materials. 2018. V. 30. № 9. P. 1705914.
- Vlasov A.I., Prisyazhnuk S.P., Zhalnin V.P. Analysis of memristor modules as an element base of microprocessor control systems: contradictions and prospects. Proceedings –- 2020 International Conference on Industrial Engineering, Applications and Manufacturing, ICIEAM 2020. 2020. № 9111917.
- Strukov D., Snider G., Stewart D. et al. The missing memristor found. Nature. 2008. V. 453. P. 80–83.
- Wang Z. et al. Memristors with diffusive dynamics as synaptic emulators for neuromorphic computing. Nat. Mater. 2016. V. 16. P. 101–108.
- Li Y. et al. Ultrafast synaptic events in a chalcogenide memristor. Sci. Rep. 2013. V. 1619.
- Zhalnin V.P., Pigina D.V., Khabarov R.A. Memristivnaya pamyat v mikro i nanoelektronike. Tekhnologii inzhenernykh i informatsionnykh sistem. 2019. № 4. S. 74–80. (in Russian).
- Vlasov A.I., Zhalnin V.P., Shakhnov V.A. Methods for improvement of the consistency and durability of the inorganic memristor structures. International Journal of Nanotechnology. 2019. V. 16. № 1-3. P. 187-195
- Zidan M.A., Strachan J.P., Lu W.D. The future of electronics based on memristive systems. Nat. Electron. 2018. V. 1. P. 22–29.
- Zahoor F., AzniZulkifli T.Z. & Khanday F.A. Resistive Random Access Memory (RRAM): an Overview of Materials, Switching Mechanism, Performance, Multilevel Cell (mlc) Storage and Modeling and Applications. Nanoscale Res. Lett. 2020. V. 15. P. 90.
- Xiao T.P., Bennett C.H., Feinberg B., Agarwal S., Marinella M.J. Analog architectures for neural network acceleration based on non-volatile memory. Appl. Phys. 2020. Rev. 7. P. 031301.
- Irmanova A., James A.P. Multi-level memristive memory with resistive networks. 2017 IEEE Asia Pacific Conference on Postgraduate Research in Microelectronics and Electronics (PrimeAsia). 2017. P. 69–72.
- Kim H., Sah M.P., Yang C., Chua L.O. Memristor-based multilevel memory in Cellular nanoscale networks and their applications (CNNA). 2010 12th international workshop on IEEE. 2010. P. 1–6.
- Sahebkarkhorasani S. A non-destructive crossbar architecture of multilevel memory-based resistor. 2015.
- Duan S., Hu X., Wang L., Li C. Analog memristive memory with applications in audio signal processing. Science China Information Sciences. 2014. V. 57. № 4. P. 1–15.
- Rabbani P., Dehghani R., Shahpari N. A multilevel memristor-cmos memory cell as a reram. Microelectronics Journal. 2015. V. 46.
№ 12. P. 1283–1290. - Shafiee A. et al. ISAAC: A Convolutional Neural Network Accelerator with In-Situ Analog Arithmetic in Crossbars. 2016 ACM/IEEE 43rd Annual International Symposium on Computer Architecture (ISCA). 2016. P. 14–26.
- Chen Y., Luo T., Liu S., Zhang S., He L., Wang J., Li L., Chen T., Xu Z., Sun N. et al. DaDianNao: A Machine-Learning Supercomputer. Proceedings of MICRO-47. 2014.
- Chi P. et al. PRIME: A Novel Processing-in-Memory Architecture for Neural Network Computation in ReRAM-Based Main Memory. 2016 ACM/IEEE 43rd Annual International Symposium on Computer Architecture (ISCA). 2016. P. 27–39.
- Chen T. et al. DianNao: A small-footprint high-throughput accelerator for ubiquitous machine-learning. Proc. ASP-LOS. 2014.
- Nag A., Balasubramonian R., Srikumar V., Walker R., Shafiee A., Strachan J., Muralimanohar N. Newton: Gravitating towards the physical limits of crossbar acceleration. IEEE Micro 38. 2018. P. 41–49.
- Ankit A., Hajj I.E., Chalamalasetti S.R., Ndu G., Foltin M., Williams R.S., Faraboschi P., Hwu W.-M.W., Strachan J.P., Roy K., Milojicic D.S. PUMA: A programmable ultra-efficient memristor-based accelerator for machine learning inference. Proceedings of the Twenty-Fourth International Conference on Architectural Support for Programming Languages and Operating Systems (ASPLOS '19). ACM. New York. 2019. P. 715–731.
- Liu X. et al 2015 Reno: A high-efficient reconfigurable neuromorphic computing accelerator design. 52 ACM/EDAC/IEEE Design Automation Conf. P. 1–6.
- Bojnordi M.N., Ipek E. Memristive boltzmann machine: a hardware accelerator for combinatorial optimization and deep learning. IEEE Int. Symp. on High Performance Computer Architecture. 2016. P. 1–13.