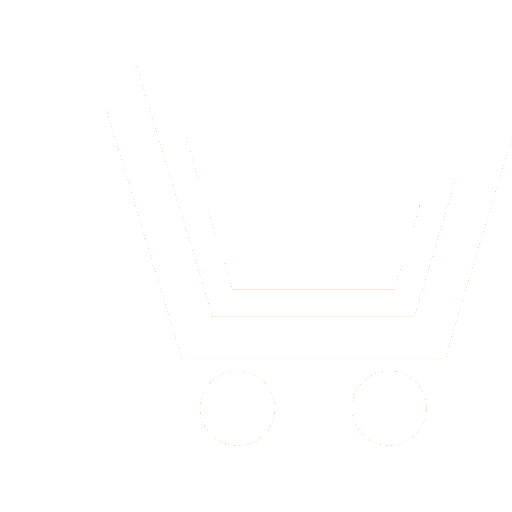
G.S. Ivanova1, Y.S. Petrova2
1,2 Bauman Moscow State Technical University (Moscow, Russia)
New valuable knowledge waits to be extracted from rich collections of thousands of paintings currently circulating in the public domain. However, manual analysis by art critics and historians is ineffective for data of such volumes. To solve this problem, computer vision technology is widely used in scientific works. At the moment, no widely used computer vision method suits the tasks of art critics and historians accurately. Therefore, analysis of existing solutions and problem revealing, that hinder the development of a comprehensive method is required. There are varying tasks in the subject area, from improving the user experience in museums to studying the perception of art. The majority of them include systematization of images by creating an informative embedding space.
Target is the study of modern computer vision methods of systematization of paintings in order to assess the state of the subject area and identify promising methods for refinement and use in applied tasks.
A comparison of publicly available paintings and fine art datasets has been carried out. The most voluminous and most commonly used datasets for evaluation of hypotheses have been identified. The existing approaches to the systematization of paintings have been analyzed. A common stage has been highlighted for the absolute majority of methods – creation of image embeddings space. The embedding retrieval methods proposed in scientific papers have been analyzed considering: the structure of input data, the features of processing algorithm, the final quality of recognition in applied problems and the credibility of datasets being used to determine accuracy of models. A group classification of these methods is proposed, which differs from known analogues by focusing on the amount and wholeness of information that is used to create an embedding. Based on the results of the analysis, the main problems of the subject area are formulated: strong influence of human factor and skill level of the annotators on the dataset, non-equality of neural and artistic styles, limitation of the possibility of analyzing the texture of brushstrokes due to low resolution, unsuitability of image analysis models for artistic canvas use. It is proposed to use a specialist-verified reference dataset to evaluate hypotheses in order to reduce the influence of the human factor. It is recommended to collect the high-resolution images dataset in order to preserve the possibility of brushstroke analysis. Methods for the context-aware embeddings retrieval are proposed as a potential solution to the problems of determining artistic style and art movement.
The results can be used to create software for painting systematization, as well as to refine old and create new methods of painting systematization. It is possible to obtain a reliable and reproducible assessment of the emerging methods by using the recommended datasets. The proposed solutions for domain problems can be used as hypotheses to improve the quality of recognition.
Ivanova G.S., Petrova Y.S. Analysis of computer vision methods for painting systematization. Neurocomputers. 2022. V. 24. № 6.
Р. 20-29. DOI: https://doi.org/10.18127/j19998554-202206-02 (In Russian).
- Zalyayeva L.M. Razvitiye khudozhestvennoy kultury i sovremennyye it-tekhnologii. Materialy Vseross. nauchno-praktich. konf. «Mnogogrannyy mir traditsionnoy kultury i narodnogo khudozhestvennogo tvorchestva» v ramkakh Vseross. konkursa AR/VR «Khakaton v sfere kultury». Kazan: Kazanskiy gos. in-t kultury. 2021. S. 254–257. (in Russian).
- Panov N.V., Komkov I.B., Savelyev A.V., Loginova N.A. Osoznaniye iskusstva neyronnymi setyami. organizuyemoye posredstvom vzaimodeystviya immunoelementov sistemy s prostranstvom. Neyrokompyutery: razrabotka. primeneniye. 2021. T. 23. № 4. S. 50–62.
(in Russian). - Lapteva V.I. Tsifrovizatsiya v muzeynykh issledovaniyakh: na materiale kollektsii metstso-tinto Ekaterinburgskogo muzeya izobrazitelnykh iskusstv. Sb. materialov Vseross. nauchno-praktich. konf. Kulturologicheskiye chteniya – 2020. Kulturnyy kod v epokhu globalizatsii: tsifrovizatsiya obshchestva i obrazovaniya (s mezhdunar.). Ekaterinburg: Uralskiy federalnyy un-t im. pervogo Prezidenta Rossii B.N. Eltsina. 2020. S. 198–203. (in Russian).
- Gorbacheva A.G. Iskusstvennyy intellekt i sovremennoye iskusstvo: novyye vozmozhnosti i vyzovy. Chelovek.RU. 2018. № 13. S. 145–154. (in Russian).
- Achlioptas P. et al. ArtEmis: Affective Language for Visual Art. arXiv preprint at arXiv.2101.07396. 2021.
- Jenicek T., Chum O. Linking Art through Human Poses. arXiv preprint arXiv.1907.03537. 2019.
- Hamilton M. et al. MosAIc: Finding Artistic Connections across Culture with Conditional Image Retrieval. arXiv preprint arXiv.2007.07177. 2020.
- Ufer N., Lang S., Ommer B. Object Retrieval and Localization in Large Art Collections using Deep Multi-Style Feature Fusion and Iterative Voting. Computer Vision – ECCV 2020 Workshops Lecture Notes in Computer Science. ECCV. 2020. V. 12536.
- Pancaroglu D. Artist, Style And Year Classification Using Face Recognition And Clustering With Convolutional Neural Networks. SIPO. AIRCC Publishing Corporation. 2020.
- Mao H., Cheung M., She J. DeepArt: Learning Joint Representations of Visual Arts. Proceedings of the 25th ACM international conference on Multimedia. 2017. P. 1183–1191.
- Elgammal A., Mazzone M., Liu B., Kim D., Elhoseiny M. The Shape of Art History in the Eyes of the Machine. arXiv preprint arXiv.1801.07729. 2018.
- Garcia N., Renoust B., Nakashima Y. Context-Aware Embeddings for Automatic Art Analysis. Proceedings of the 2019 on International Conference on Multimedia Retrieval. ICMR. 2019. P. 25–33.
- El Vaigh C.B. et al. GCNBoost: Artwork Classification by Label Propagation through a Knowledge Graph. arXiv preprint arXiv.2105.11852. 2021.
- Castellano G., Sansaro G., Vessio G. Integrating Contextual Knowledge to Visual Features for Fine Art Classification. arXiv preprint arXiv.2105.15028. 2021.
- Efthymiou A., Rudinac S., Kackovic M., Worring M., Wijnberg N. Graph Neural Networks for Knowledge Enhanced Visual Representation of Paintings. arXiv preprint arXiv.2105.08190. 2021.
- Shen X., Efros A., Joulin A., Aubry M. Learning Co-segmentation by Segment Swapping for Retrieval and Discovery //arXiv preprint arXiv.2110.15904. 2021.
- Castellano G., Vessio G. A deep learning approach to clustering visual arts. arXiv preprint arXiv.2106.06234. 2021.
- Shaik S., Bucher B., et al. Learning Portrait Style Representations. arXiv preprint arXiv.2012.04153. 2020.
- Parés F. et al.The MAMe Dataset: On the relevance of High Resolution and Variable Shape image properties. arXiv preprint at arXiv.2007.13693. 2020.
- Dominguez V., Messina P., Parra D., Mery D., Trattner C., Soto A. Comparing Neural and Attractiveness-based Visual Features for Artwork Recommendation. Proceedings of the 2nd Workshop on Deep Learning for Recommender Systems. DLRS. 2017. P. 55–59.
- Messina P., Dominguez V., Parra D., Trattner C., Soto A. Exploring Content-based Artwork Recommendation with Metadata and Visual Features //arXiv preprint arXiv.1706.05786. 2017.
- Ruta D. et al. StyleBabel: Artistic Style Tagging and Captioning //arXiv preprint arXiv.2203.05321. 2022.
- Zhdanova V.A. Neyronnyye seti v iskusstve: mezhdu imitatsiyey i verifikatsiyey. Sb. materialov 2-y molodezhnyy konvent: materialy mezhdunar. studencheskoy konf. «Mnogomernost obshchestva: chelovek v sotsialnom vzaimodeystvii». Ekaterin-burg: Uralskiy federalnyy un-t im. pervogo Prezidenta Rossii B.N. Eltsina. 2018. S. 29–32. (in Russian).
- Boulton P., Hall P. Artistic Domain Generalisation Methods are Limited by their Deep Representations. arXiv preprint arXiv.1907.12622. 2019.
- Zahedi M.A., Gholamrezaei N., Doboli A. How Deep is Your Art: An Experimental Study on the Limits of Artistic Understanding in a Single-Task, Single-Modality Neural Network. arXiv preprint arXiv.2203.16031. 2022.
- Ivanova G.S., Golovkov A.A., Umnov A.V., Petrova Ya.S. i dr. Metody mashinnogo obucheniya v zadache diagnostirovaniya rentgenovskikh mammologicheskikh snimkov. Dinamika slozhnykh sistem – XXI vek. 2019. T. 13. № 1. S. 25–32. (in Russian).