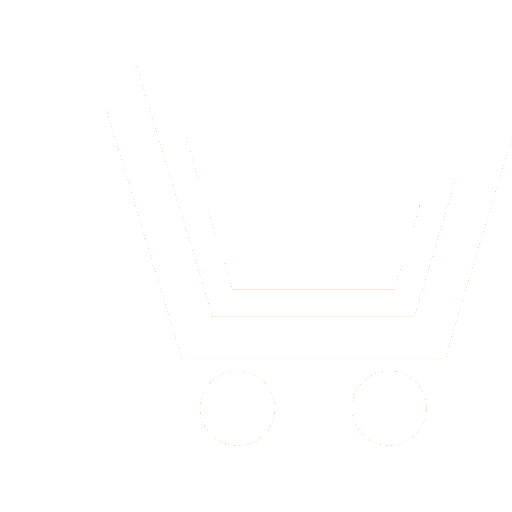
G.N. Каmyshova1
1 Financial University under the Government of the Russian Federation (Moscow, Russia)
Irrigated agriculture as part of the agricultural sector is becoming increasingly important in light of climate change and the growing need for food. At the same time, the tasks of optimizing various parameters involved in irrigation planning remain relevant to this day. Models based on artificial neural networks are finding more and more applications in solving such problems; therefore, the development of approaches to the use of neural network models for predicting parameters that allow optimizing irrigation is a very urgent task. The aim of the study is to develop a modern approach to parameter modeling based on artificial neural networks for crop irrigation optimization. As a result, neural network models are proposed for predicting the parameters involved in irrigation planning in Russian reclamation practice. A comparative analysis of the proposed models with classical methods used in irrigation practice has been carried out. It has been established that the operational planning of irrigation using the constructed models allows maintaining the moisture deficit in the soil within acceptable limits for most of the simulated growing season, while optimizing the use of water while maintaining crop yields. The problems and directions for further work on the development of neural network models of irrigated agriculture are outlined. The development of applied neural network models in the problems of irrigated agriculture is a fairly new direction for the Russian agricultural industry. Due to the accelerated development of digitalization, which makes it possible to collect huge amounts of data on production processes in agriculture, it is necessary to develop applied neural network modeling to increase its efficiency. The results obtained clearly demonstrate their capabilities in optimizing the production of agricultural products, namely, the generalizing ability of neural network models provides an opportunity to develop models for predicting moisture in large areas of irrigation and will reduce the impact of spatial and temporal variability on the crop.
Каmyshova G.N. Neural network models in crop irrigation optimization. Neurocomputers. 2022. V. 24. № 5. Р. 44-54.
DOI: https://doi.org/10.18127/j19998554-202205-05 (in Russian)
- Kvachantiradze Je. P. Model' prognoza vlazhnosti pochv i pravomernost' ee ispol'zovanija. Prirodoobustrojstvo. 2020. № 3. S. 14-20 (in Russian).
- Diao W., Liu G., Zhang H., Hu K., Jin X. Influences of Soil Bulk Density and Texture on Estimation of Surface Soil Moisture Using Spectral Feature Parameters and an Artificial Neural Network Algorithm. Agriculture. 2021. V. 11. Р. 710.
- Wang J., Peng J., Li H., Yin C., Liu W., Wang T., Zhang H. Soil Salinity Mapping Using Machine Learning Algorithms with the Sentinel-2 MSI in Arid Areas, China. Remote Sens. 2021. V. 13. Р. 305.
- Diez F.J., Navas-Gracia L.M., Chico-Santamarta L., Correa-Guimaraes A., Martínez-Rodríguez A. Prediction of Horizontal Daily Global Solar Irradiation Using Artificial Neural Networks (ANNs) in the Castile and León Region, Spain. Agronomy. 2020. V. 10. Р. 96.
- D’Emilio A., Aiello R., Consoli S., Vanella D., Iovino M. Artificial Neural Networks for Predicting the Water Retention Curve of Sicilian Agricultural Soils. Water. 2018. V. 10. Р. 1431.
- Cáceres G., Millán P., Pereira M., Lozano D. Smart Farm Irrigation: Model Predictive Control for Economic Optimal Irrigation in Agriculture. Agronomy. 2021. V. 11. Р. 1810.
- Kujawa S., Niedbała G. Artificial Neural Networks in Agriculture. Agriculture. 2021. V. 11. Р. 497.
- Glória A., Cardoso J., Sebastião P. Sustainable Irrigation System for Farming Supported by Machine Learning and Real-Time Sensor Data. Sensors. 2021. V. 21. Р. 3079.
- Kamyshova G.N., Soloviov D.A., Kolganov D.A., Korsak V.V., Terekhova. Neuromodeling in Irrigation Management for Sustainable Agriculture. Advances in Dynamical Systems and Applications. 2021. V. 16. № 1. P. 159-170.
- Kamyshova G., Solovyev D., Terekhova N., Kolganov D. Development of Approaches to the Intellectualization of Irrigation Control Systems. Smart Innovation, Systems and Technologies. 2022. V. 245. P. 359-369.
- Kamyshova G., Osipov A., Gataullin S., Korchagin S., Ignar S., Gataullin T., Terekhova N., Suvorov S. Artificial Neural Networks and Computer Vision's-Based Phytoindication Systems for Variable Rate Irrigation Improving. IEEE Access. 2022. V. 10. P. 8577-8589.
- Kamyshova G. N. Primenenie iskusstvennyh nejronnyh setej dlja upravlenija orosheniem. Agrarnyj nauchnyj zhurnal. 2021. № 4. S. 84-88 (In Russian).
- Bondarovich A.A., Pon'kina E.V., Shmidt G., Illiger P. Sravnitel'nyj analiz pokazatelej ob’ema i dostupnosti pochvennoj vlagi pri razlichnyh tehnologijah zemledelija v uslovijah suhoj stepi Zapadnoj Sibiri. Agrofizika. 2020. № 3. S. 6-16 (In Russian).
- Kravchuk A.V., Korsak V.V., Kudajbergenova I.R., Uldanov A.G. Ustanovlenie sloja uvlazhnenija po kornevoj sisteme kukuruzy. Evrazijskij Sojuz Uchenyh (ESU). 2020. № 5(74). S. 34-36 (In Russian).
- Pankova T. A. Povyshenie jeffektivnosti ispol'zovanija vodnyh resursov pri oroshenii ljucerny na temno-kashtanovyh pochvah suhostepnogo Zavolzh'ja. Saratov. FGBUN Nauchnyj i izdatel'skij centr «Nauka» RAN. 2017. 110 s. (In Russian).
- Kravchuk A.V., Korsak V.V., Kudajbergenova I.R. Parametry uvlazhnenija pochvy v provedenii jekologicheskih rezhimov oroshenija kul'tur. Vestnik uchebno-metodicheskogo ob’edinenija po obrazovaniju v oblasti prirodoobustrojstva i vodopol'zovanija. 2018. № 11. S. 126-130 (In Russian).
- Pankova T. A. Normirovanie oroshenija ljucerny v uslovijah Saratovskogo Zavolzh'ja. V kn.: Sb. statej nacion. nauch.-praktich. konf. «Agrarnaja nauka i obrazovanie: problemy i perspektivy» (g. Saratov, 20–21 marta 2021 g.). Pod red. E.B. Dudnikovoj. Saratov: OOO «Centr social'nyh agroinnovacij SGAU». 2021. S. 269-271 (In Russian).
- Hajkin S. Nejronnye seti: polnyj kurs. Izd. 2-e. M.; SPb: Dialektika. 2019. 1103 s. (In Russian).