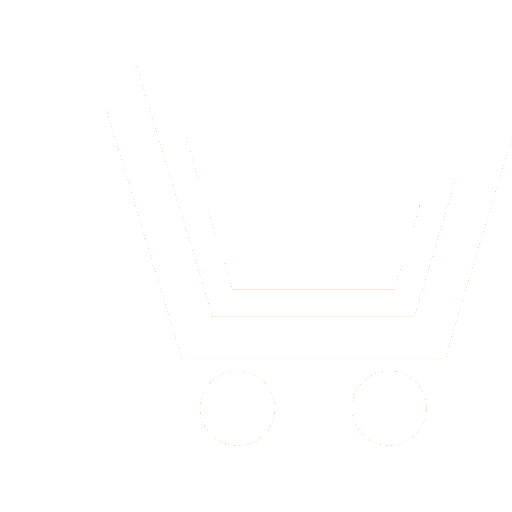
V.A. Yemelyanov1, G.V. Feklin2, N.Yu. Yemelyanova3
1-3 Financial University under the Government of the Russian Federation (Moscow, Russia)
The article substantiates the relevance of complex automated diagnostics of the metals state according to all characteristics. An algorithm for automating the metallographic quality control of metals is proposed and described, based on the use of neural networks for recognizing images of metal microstructures and the case-based reasoning approach for determining the metal grade. An approach to preprocessing images of metal microstructures is described. The structure of a neural network to determine the quantitative characteristics of metals has been developed. The results of the functioning of neural networks for determining the quantitative characteristics of metals are presented. The high accuracy of determining the characteristics of metals using neural networks is noted. Software has been developed for automated recognition of images of metal microstructures and for determining the grade of metal. Comparative results of metallographic analysis using the developed tools are demonstrated, in which there is a significant reduction in time spent on the analysis of metallographic images, as well as an increase in the accuracy of determining the quantitative characteristics of metals.
Yemelyanov V.A., Feklin G.V., Yemelyanova N.Yu. Application of artificial intelligence technologies to assess the quality of metals in a metallurgical production. Neurocomputers. 2022. V. 24. № 5. Р. 28-35. DOI: https://doi.org/10.18127/j19998554-202205-03
(in Russian)
- Smallman R.E., Ashbee K.H.G. Modern Metallography: The Commonwealth and International Library: Metallurgy Division. Elsevier. 2013. 224 p.
- Gordon Y., Kumar S., Freislich M., Yaroshenko Y. The modern technology of iron and steel production and possible ways of their development. Steel in Translation. 2015. V. 45. № 9. Р. 627-634.
- Colpaert H. Metallography of Steels: Interpretation of Structure and the Effects of Processing. ASM International. USA. 2018. 699 p.
- Martyushev N., Skeeba V. The method of quantitative automatic metallographic analysis. Journal of Physics: Conference Series. 803. 012094. 2017. DOI:10.1088/1742-6596/803/1/012094.
- DeCost B.L., Holm E.A. A computer vision approach for automated analysis and classification of microstructural image data. Computational Materials Science. 2015. № 110. Р. 126-133.
- Bezyazychnyi V.F., Palamar’ I.N., Azikov N.S. Automation of Analysis of the Structure of a Material with Details Based on Controlled Functional Systems. Journal of Machinery Manufacture and Reliability. 2020. № 49. Р. 341–346.
- Wu W.-H., Lee J.-C., Wang Y.-M. A Study of Defect Detection Techniques for Metallographic Images. Sensors. 2020. № 20. Р. 5593.
- Dali Chen, Yang Liu, Shixin Liu, Fang Liu, Yangquan Chen. Framework of Specific Description Generation for Aluminum Alloy Metallographic Image Based on Visual and Language Information Fusion. Symmetry. 2020. № 12. Р. 771.
- Niezgoda S.R., Kanjarla A.K., Kalidindi S.R. Novel microstructure quantification framework for databasing, visualization, and analysis of microstructure data. Integrating Materials and Manufacturing Innovation. 2013. V. 2. № 1. Р. 1-27.
- Lin J., Ma L., Yao Y. Segmentation of casting defect regions for the extraction of microstructural properties. Eng. Appl. Artif. Intell. 2019. V. 85. Р. 150–163.
- Azimi S.M., Britz D., Engstler M., Fritz M., Mücklich F. Advanced steel microstructure classification by deep learning methods. Sci. Rep. 2018. № 8. Р. 2128.
- Ma B., Ban X., Huang H., Chen Y., Liu W., Zhi Y. Deep learning-based image segmentation for al-la alloy microscopic images. Symmetry. 2018. № 10. Р. 107.
- Injuhin M.V., Korzhavyj A.P., Maksimov V.V., Shatalov V.K. Jeffektivnaja tehnologija izvlechenija cvetnyh metallov iz zhidkih othodov proizvodstva. Naukoemkie tehnologii. 2021. T. 22. № 1. S. 13−20 (in Russian).