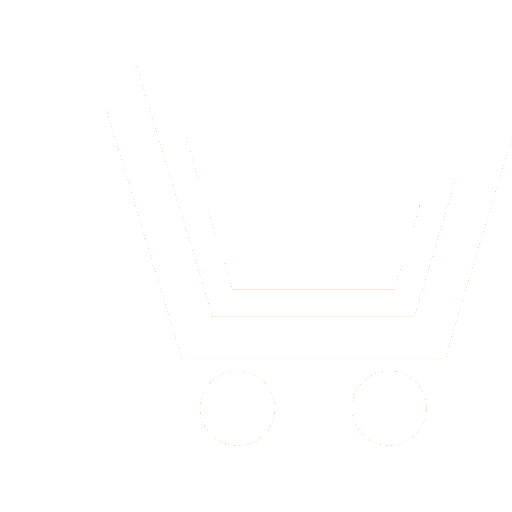
K.D. Rusakov1, A.O. Iskhakova2, R.V. Meshcheryakov3
1-3 V. A. Trapeznikov Institute of Control Sciences of Russian Academy of Sciences (Moscow, Russia)
In order to counteract the manifestation of aggression, pressure and other forms of destructive influence on the individual and group consciousness of users, the need arose to solve the problem of detecting destructive content, such as explicit nudity, violence, content of concern, drugs, alcohol, is relevant in the context of a fast pace of digitalization and generation of heterogeneous Internet content. The aim of the work was to analyze the existing developments in the field of detecting destructive heterogeneous content and to solve the problem of the minimum available information. The proposed system for detecting destructive content is based on a convolutional neural network that returns the probabilities of different classes of content. Next, the class corresponding to the maximum probability is selected. The experimental result shows that the proposed model provides an average top1 accuracy of 80% for all classes when tested on our sets of images. The practical significance of the obtained solution for detecting destructive content in images will reduce the volume (up to 10%), and, accordingly, increase the quality of the work carried out to recognize destructive content.
Rusakov K.D., Iskhakova A.O., Meshcheryakov R.V. Recognition of destructive multimedia content in the socio-cyberphysical Internet monitoring system by a single frame. Neurocomputers. 2022. V. 24. № 3. Р. 5-17. DOI: https://doi.org/10.18127/j19998554-202203-01 (in Russian)
- Kulagina I.V., Ishakova A.O., Galin R.R. Modelirovanie praktik agressii v socio-kiberfizicheskoj srede. Vestnik tomskogo gosudarstvennogo universiteta. Filosofija. Sociologija. Politologija. 2019. № 52. S. 147-161 (in Russian).
- Vlasenko M.S. Obespechenie informacionnoj bezopasnosti nesovershennoletnih v seti internet: sovremennoe sostojanie i sovershenstvovanie pravovogo regulirovanija. Vestnik Volzhskogo universiteta im. V.N. Tatishheva. 2019. T. 1. № 3. S. 98-105 (in Russian).
- Kazarin O.V., Skiba V.Ju., Sharjapov R.A. Novye raznovidnosti ugroz mezhdunarodnoj informacionnoj bezopasnosti. Vestnik RGGU. Serija: Dokumentovedenie i arhivovedenie. Informatika. Zashhita informacii i informacionnaja bezopasnost'. 2016. № 1(3). S. 54-72 (in Russian).
- Iskhakova A., Iskhakov A., Meshcheryakov R. Research of the estimated emotional components for the content analysis. Journal of Physics Conference Series. V. 1203(1). Article 012065. DOI:10.1088/1742-6596/1203/1/012065.
- Ohapkin V.P., Ohapkina E.P., Ishakova A.O., Ishakov A.Ju. Destruktivnoe informacionno-psihologicheskoe vozdejstvie v social'nyh setjah. Modelirovanie, optimizacija i informacionnye tehnologii. 2020. T. 8. № 1(28). S. 22-23 (in Russian).
- Levonevskii D., Shumskaya O., Velichko A., Uzdiaev M., Malov D. Methods for Determination of Psychophysiological Condition of User Within Smart Environment Based on Complex Analysis of Heterogeneous Data / Ronzhin A., Shishlakov V. (eds). Proceedings of 14th International Conference on Electromechanics and Robotics “Zavalishin's Readings”. Smart Innovation, Systems and Technologies. V. 154. Springer. Singapore. DOI: 10.1007/978-981-13-9267-2_42.
- Iskhakova А.O., Rusakov K.D., Iskhakov A.Y., Mamchenko M.V. Detection of destructive multimedia content in the socio-cyberphysical Internet monitoring system. Management of Large Systems: Proceedings of XVII All-Russian School-Conference of Young Scientists. Moscow-Zvenigorod. September 06-09 2021. Moscow: ICS RAS. 2021. P. 202-212.
- Lin Y.C., Tseng H.W., Fuh C.S. Pornography detection using support vector machine. Proceedings of the 16th IPPR Conference on Computer Vision, Graphics and Image Processing (CVGIP '03). 2003. V. 19. Р. 123–130.
- Zhu H., Zhou S., Wang J., Yin Z. An algorithm of pornographic image detection. Proceedings of the 4th International Conference on Image and Graphics (ICIG '07). August 2007. Р. 801–804.
- Kakumanu P., Makrogiannis S., Bourbakis N. A survey of skin-color modeling and detection methods. Pattern Recognition. 2007. V. 40. № 3. Р. 1106–1122.
- Basilio J.A.M., Torres G.A., Perez G.S., Medina L.K.T., Meana H.M.P., Hernadez E.E. Explicit content image detection. Signal and Image Processing: International Journal. 2010. V. 1. № 2. Р. 47–58.
- Marcial-Basilio J.A., Aguilar-Torres G., Sánchez-Pérez G., et al. Detection of pornographic digital images. International Journal of Computers. 2011. V. 5. № 2. Р. 298–305.
- Basilio J.A.M., Torres G.A., Pérez G.S., Medina L.K.T., Meana H.M.P. Explicit image detection using YCbCr space color model as skin detection. Applications of Mathematics and Computer Engineering. 2011. Р. 123–128.
- Sumon S.A., Goni R., Hashem N.B., Shahria T., Rahman R.M. Violence Detection by Pretrained Modules with Different Deep Learning Approaches. Vietnam Journal of Computer Science. 2020. V. 7. № 1. P. 19–40.
- Sa Sajjad M., Khan S., Muhammad K., Wu W., Ullah A., Baik S.W. Multi-Grade Brain Tumor Classification using Deep CNN with Extensive Data Augmentation. Journal of Computational Science. 2019. V. 30. P. 174-182.
- Zihang D., et al. CoAtNet: Marrying Convolution and Attention for All Data Sizes. arXiv preprint arXiv:2106.04803 (2021).
- Sandler M., Howard A., Zhu M., Zhmoginov A., Chen L.-C. Mobilenetv2: Inverted residuals and linear bottlenecks. Proceedings of the IEEE conference on computer vision and pattern recognition. 2018. Р. 4510–4520.
- Sifre L. Rigid-motion scattering for image classification. Ph.D. thesis section 6.2. 2014.
- Ribani R., Marengoni M. A Survey of Transfer Learning for Convolutional Neural Networks. 2019 32nd SIBGRAPI Conference on Graphics, Patterns and Images Tutorials (SIBGRAPI-T). 2019. Р. 47-57.
- Zhang Z. Improved Adam Optimizer for Deep Neural Networks. 2018 IEEE/ACM 26th International Symposium on Quality of Service (IWQoS). 2018. Р. 1-2.
- Smith L.N. Cyclical learning rates for training neural networks. 2017 IEEE winter conference on applications of computer vision (WACV). 2017.