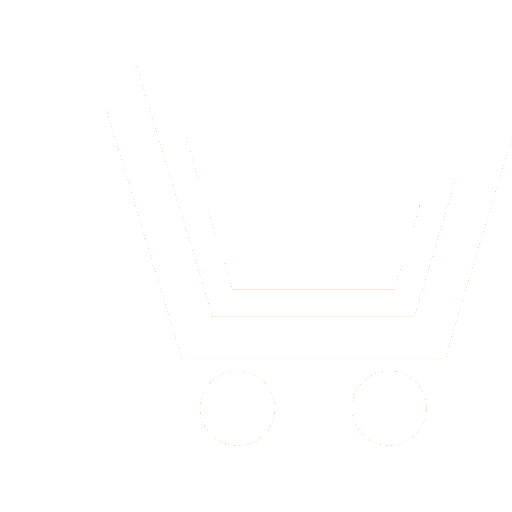
E.V. Egorova1, M.H. Aksayitov2, A.N. Ribakov3
1 MTU MIREA (Moscow, Russia)
2 JSC OKB Electroautomatics (Moscow, Russia)
3 FSUE VNIIA named after N.L. Dukhova (Moscow, Russia)
Neural networks make it possible to successfully solve the problems of recognizing radar information due to the ability to learn and approximate the optimal dividing surfaces, as well as to efficiently represent fuzzy features in the feature space, while the success of using neural networks in target recognition tasks is confirmed by numerous examples described in the literature. It is necessary to improve the methods of detecting and separating signals from interference, as well as to find new methods and means of recognition, taking into account modern radio masking of objects using associative memory algorithms. Currently, there are many different neural network models or paradigms devoted to the theory of neural networks, each direction requires taking into account its inherent features. In this regard, new neural network paradigms appear that are best suited to solving certain problems. For several decades, during which neural networks and the possibilities of their application to various fields have been actively and intensively studied, many variants of neural network architectures have been developed, and many different types of neural networks, most of which were obtained in the process of solving specific problems. Among the variety of achievements of neural network research, there are already important theoretical results that substantiate the possibility of using some models, assessing their properties and guaranteeing, in asymptotics, the effectiveness of their use for solving the problems posed. This article discusses the use of neural networks in the processing of radar information, which makes it possible to successfully solve recognition problems, due to the ability to learn and approximate the optimal dividing surfaces, as well as the effective representation of fuzzy features in the feature space, while the success of the use of neural networks in target recognition problems is confirmed by numerous examples. The relevance of studies based on the rational use of neural network technology, dedicated to the prevention of cases of deterioration in the quality of radar information in conditions of a large number of targets and a complex jamming environment, is determined. It should be noted that one of the most important among the tasks of radar is the task of detecting radar targets, in which, based on the signals received by the locator, after filtering them, a decision is made about the presence of a target. It is at this stage that the greatest difficulties of trajectory processing begin to appear, the ambiguity of identifying marks in successive surveys, which is successfully eliminated using the proposed version of associative memory, which allows reproducing the memorized image of a group when it moves arbitrarily, while requiring several orders of magnitude less computing power. than the traditional Hopfield neural network model. The reproduction of the marks memorized in the previous survey using the Hopfield network in subsequent surveys makes it possible to carry out reliable identification and form the trajectories of radar targets already in the first surveys after their appearance.
Egorova E.V., Aksyaitov M.H., Rybakov A.N. Application of the neural network model of Hopfield’s associative memory in the task of processing radar information. Nonlinear World. 2020. V. 18. № 4. 2020. P. 24−33. DOI: 10.18127/j20700970-20200403 (In Russian).
- Tatuzov A.L. Nejronnye seti v zadachah radiolokacii. Kn. 28. M.: Radiotehnika. 2009. S. 432.
- Aksjaitov M.H., Egorova E.V., Rybakov A.N. Raspoznavanie ob’ekta radiolokacionnogo izobrazhenija s ispol'zovniem nejrosetevyh struktur seti Hjemminga. Uspehi sovremennoj radiojelektroniki. 2019. № 6. S. 37−43 (In Russian).
- Vajncvajg M.N., Dimentman A.M. Associativnoe zapominajushhee ustrojstvo s rasshirennymi funkcional'nymi vozmozhnostjami. Voprosy kibernetiki. M.: Sovetskoe radio. 1979. Vyp. 49. S. 120−126 (In Russian).
- Galushkin A.I. Teorija nejronnyh setej. Nejrokomp'jutery i ih primenenie. Kn. 1. M.: IPRZhR. 2000. 416 s. (In Russian).
- Kwan C. Neural Network Control of Nonlinear Systems Using Multiple Models. DoD STTR Program. NAVY 98-005. USA. 1998.
- Krugloe V.V., Dli M.I., Golunov R.Ju. Nechetkaja logika i iskusstvennye nejronnye seti. M. 2001. S. 24 (In Russian).
- Panov V.V. O metodicheskih podhodah k kompleksnomu obosnovaniju i ocenke vooruzhenija i voennoj tehniki. Vooruzhenie. Politika. Konversija. 2002. № 1. S. 3−5 (In Russian).
- Tatuzov A.L. Neural Network Methods for Radar Processing. ITONIP'02. IEEE. 2002. V. 4. P. 1718−1723.
- Zhong S., Ghosh J. Decision Boundary Focussed Neural Network Classifier. Intelligent Engineering Systems Through Artificial Neural Networks. V. 10. ASME Press (ProcANNIE '00). 2000.
- Behar V.P., Kabakchiev C.A., Doukovska L.A. Adaptive CFAR PI Processor for Radar Target Detection in Pulse Jamming. Journal of VLSI Signal Processing. 2000. V. 26. P. 383−396.
- Hopfild D. Nejronnye seti i fizicheskie sistemy, projavljajushhie svojstva kollektivnyh vychislenij. Pod red. A.I. Galushkina. Nejrokomp'juternye seti: Istorija razvitija teorii. M.: IPRZhR. 2001 (In Russian).