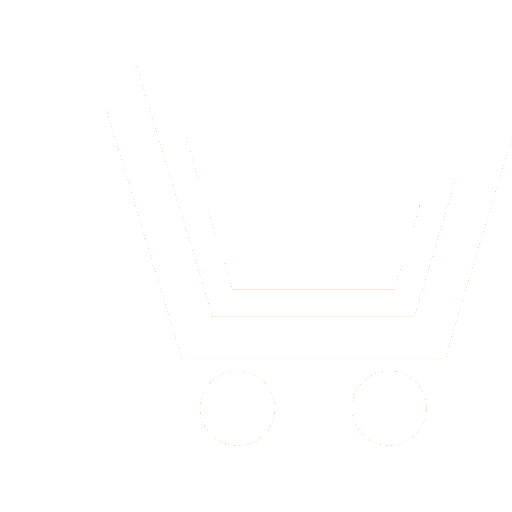
N.V. Cheltsov1, M.V. Petuhov2, A.V. Lavrov3, V.A. Solovjev4
1–4 Bauman Moscow State Technical University (Moscow, Russia)
This article deals with the digital processing of a matrix radar image. The information received from the radar scanner needs to be transformed to enable visual perception. The article describes the main methods of digital processing of matrix data, presents the images transformed by them.
The aim of the research – development of a radar data processing algorithm that identifies the contours and edges of examined objects.
The authors propose an algorithm for isolating the geometric structure of the scanned area. The difference between the processing method and the known analogues is based on the nature of the change in the values of the array being processed and consists in the double operation of extracting the gradient of the distribution of values.The software implementation of the algorithm is made in C++ using methods from an open library of computer vision. The efficiency of the algorithm was estimated based on comparison with the algorithms for determining edges based on linear filtering and neural networks.
The results of the work can be used to create software for mobile short-range radar devices. Imaging from object boundaries and their edges provides spatial perception of the image by the operator, and free areas are available for rendering additional information. This solution allows you to combine scanning devices and thereby increase the information value of the result.
Cheltsov N.V., Petuhov M.V., Lavrov A.V., Solovjev V.A. Neural network processing of radarscanning data array. Neurocomputers. 2021. V. 23. № 6. Р. 32−47. DOI: https://doi.org/10.18127/j19998554-202106-04 (in Russian).
- Attestatsiya algoritmov opredeleniya vesovykh koeffitsiyentov pokazateley kachestva [Elektronnyy resurs]. Elektronnyy nauchnyy zhurnal «Sovremennyye problemy nauki i obrazovaniya». URL: http://www.science-education.ru/106-7335 (data obrashcheniya: 10.12.2021) (in Russian).
- Bayesovskaya teoriya klassifikatsii i metody vosstanovleniya plotnosti [Elektronnyy resurs]. Mashinnoye obucheniye. URL: http://www.MachineLearning.ru/wiki/images/f/fc/Voron-ML-Metric-slides.pdf (data obrashcheniya: 05.12.2021) (in Russian).
- Blekuell D., Girshik M.A. Teoriya igr i statisticheskikh resheniy. M.: Izd-vo inostrannoy literatury. 1958. 374 s. (in Russian).
- Gorelik A.L., Skripkin V.A. Metody raspoznavaniya. M.: Vysshaya shkola. 1977. 262 s. (in Russian).
- Gorelik A.L., Barabash Yu.L., Krivosheyev O.V., Epshteyn S. Selektsiya i raspoznavaniye na osnove lokatsionnoy informatsii. M.: Radio i svyaz. 1981. 240 s. (in Russian).
- Gruzman I.S., Kirichuk V.S. Tsifrovaya obrabotka izobrazheniy v informatsionnykh sistemakh: Uchebnoye posobiye. Novosibirsk: Izd-vo NGU. 1974. S. 76. (in Russian).
- Kiselev N.V. Metody postroyeniya sistem raspoznavaniya i klassifikatsii negaussovykh signalov. L.: Izd-vo Leningr. Universiteta. 1986. S. 188. (in Russian).
- Kuzmin S.Z. Tsifrovaya radiolokatsiya. Vvedeniye v teoriyu. Kiyev: KViTs. 2000. 428 s. (in Russian).
- Metody opredeleniya znacheniy vesovykh koeffitsiyentov [Elektronnyy resurs]. Matmetody.rf. URL: http://matmetody.rf/metodiopredeleniya-znacheniy-vesovih-koeffitsientov/ (data obrashcheniya: 30.11.2021) (in Russian).
- Senin A.G. Raspoznavaniye sluchaynykh signalov. Novosibirsk: Nauka. Sibirskoye otdeleniye. 1974. S. 76. (in Russian).
- Tatuzov A.L. Neyronnyye seti v zadachakh radiolokatsii. Kn. 28. M.: Radiotekhnika. 2009. 432 s. (in Russian).
- Frenks L. Teoriya signalov. M.: Sovetskoye radio. 1974. 344 s. (in Russian).
- Fomin V.N. Rekurrentnoye otsenivaniye i adaptivnaya filtratsiya. M.: Nauka. 1984. 288 s. (in Russian).
- Fukunaga K. Vvedeniye v statisticheskuyu teoriyu raspoznavaniya obrazov. M.: Nauka. glavnaya redaktsiya fiziko-matematicheskoy literatury. 1979. 368 s. (in Russian).
- Chernova N.I. Matematicheskaya statistika: Uchebnoye posobiye. M.: Novosib. gos. un-t. 2007. 148 s. (in Russian).
- Shirman Ya.D., Manzhos V.N. Teoriya i tekhnika obrabotki radiolokatsionnoy informatsii na fone pomekh. M. Radio i svyaz. 1981. 416 s. (in Russian).
- Viryasova A.Yu., Vlasov A.I., Gladkikh A.A. Neyrosetevyye metody defektoskopii integralnykh struktur. Neyro- kompyutery: razrabotka. primeneniye. 2019. № 2. S. 54–67. (in Russian).
- Buyanov A.I., Vlasov A.I., Zagoskin A.V. Primeneniye neyrosetevykh metodov pri defektoskopii pechatnykh plat. Neyrokompyutery: razrabotka. primeneniye. 2002. № 3. S. 42–70. (in Russian).
- Gridnev V.N., Vlasov A.I., Konstantinov P., Yudin A.V. Neyrosetevyye metody defektoskopii pechatnykh plat. Elektronnyye komponenty. 2004. № 8. S. 148–155. (in Russian).
- Averianikhin A.E., Vlasov A.I., Evdokimova E.V. Ierarkhicheskaya piramidalnaya subdiskretizatsiya v glubokikh svertochnykh setyakh dlya raspoznavaniya vizualnykh obrazov. Neyrokompyutery: razrabotka. primeneniye. 2021. T. 23. № 1. S. 17–31. (in Russian).
- Shakhnov V.A., Vlasov A.I., Polyakov Yu.A., Kuznetsov A.S. Neyrokompyutery: arkhitektura i skhemotekhnika. Ser. Prilozheniye k zhurnalu «Informatsionnyye tekhnologii». № 9. Mashinostroyeniye. 2000. S. 35–38. (in Russian).
- Balukhto A.N., Bulayev V.I., Buryy E.V. i dr. Neyrokompyutery v sistemakh obrabotki izobrazheniy. Ser. Biblioteka zhurnala «Neyrokompyutery: razrabotka. primeneniye». Radiotekhnika. 2003. S. 15–16. (in Russian).
- Artemyev B.V., Popov D.V., Dmitriyev V.E. Analiz osobennostey obnaruzheniya obyektov. raspolozhennykh na odnoy osi otnositelno nablyudatelya. metodom radiolokatsii v millimetrovom diapazone. Kontrol. Diagnostika. 2019. № 6. S. 42–47. (in Russian).
- Krashennikov V.R. Statisticheskie metody obrabotki izobrazhenij: Ucheb. posobie. Uljyanovsk: UlGTU. 2015. 167 s. (in Russian).