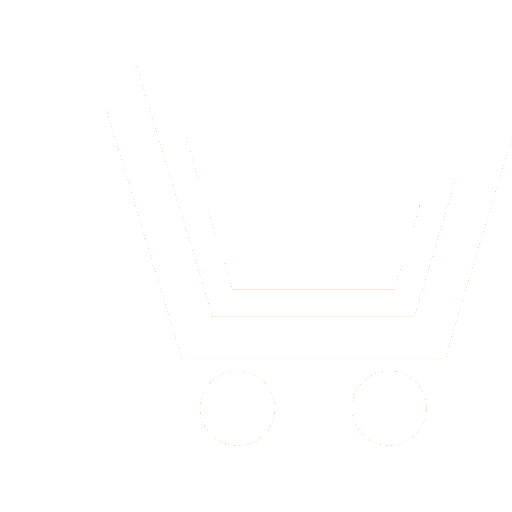
V.V. Belousov1,O.V. Druzhinina2, E.R. Korepanov3, I.V. Makarenkova4, V.V. Maksimova5
1-5 FRС «Computer Science and Control» of RAS (Moscow, Russia)
The development of intelligent methods and the development of tools for solving research problems of modeling and diagnosing the state of technical systems are relevant areas related to the introduction of digital technologies. Such problems include the problems of preparing correct data arrays for diagnosing and predicting the state of elements and nodes of transport systems.
The purpose of the paper is to develop methods for preparing and analyzing data for modeling digital twins on the example of studying the temperature regime of the functioning of a railway car's axle box node, as well as choosing the architecture of neural networks for solving problems of assessing the technical condition of the axle box nodes.
The features of the digital twin technology are characterized and the directions of its use for modeling and diagnostics of railway transport systems are considered. As part of the development of an approach to solving the problem of diagnosing the condition of the axle box units of railway cars, analytical dependences and generalized heat transfer equations related to changes in temperature regimes are considered. Examples of the presentation of such data on temperature series, which contain values for deviations from the normal functioning of the elements of the axle box node, are given. The choice of the neural network architecture adapted for solving the problems of estimating and predicting the temperature values of the axle box unit of the car was made. Options for preparing test data for a neural network model have been developed.
The results can be used in the problems of creating algorithmic and software for preparing correct arrays of input data for technical diagnostics, in the problems of synthesis and analysis of models of intelligent systems, in various machine learning problems. The considered approach to modeling is aimed at developing methods for assessing and predicting the state of transport infrastructure elements and can be used in the development of intelligent transport systems and for improving the technology of digital twins.
Belousov V.V., Druzhinina O.V., Korepanov E.R., Makarenkova I.V., Maksimova V.V. An approach to assessing the technical condition of elements and nodes of transport systems using neural network modeling methods and digital twin technology. Neurocomputers. 2021. V. 23. № 5. 2021. P. 5−20. DOI: https://doi.org/10.18127/j19998554-202105-01 (In Russian)
- Rossijskij rynok iskusstvennogo intellekta: problemy i perspektivy [Jelektronnyj resurs]. www.TAdvisor.ru/index/php/ (дата обращения data obrashhenija 25.05.2021) (In Russian).
- Zacarinnyj A.A., Kiselev Je.V., Kozlov S.V., Kolin K.K. Informacionnoe prostranstvo cifrovoj jekonomiki Rossii. Konceptual'nye osnovy i problemy formirovanija. Pod obshh. red. A.A. Zacarinnogo. M.: FIC IU RAN. 2018. 236 s. (In Russian).
- Koncepcija realizacii kompleksnogo nauchno-tehnicheskogo proekta «Cifrovaja zheleznaja doroga». M.: OAO RZhD. 2017 (In Russian).
- Boschert S., Rosen R. Digital twin − the simulation aspect. Mechatronic Futures: Challenges and Solutions for Mechatronic Systems and their Designers. Springer International Publishing. 2016. P. 59−74 (In Russian).
- Prohorov A., Lysachev M. Cifrovoj dvojnik. Analiz, trendy, mirovoj opyt. Pod red A. Borovkova. M.: OOO «Al'jans Print». 2020 (In Russian).
- Sinicyn I.N., Shalamov A.S. Lekcii po teorii sistem integrirovannoj logisticheskoj podderzhki. M.: TORUS-PRESS. 2019 (In Russian).
- Samsonov A.O. Cifrovoe modelirovanie zheleznodorozhnogo puti. Obrazovatel'nye resursy i tehnologii. 2016. № 3(15). S. 104114 (In Russian).
- Rozenberg E.N., Korovin A.S. Global'nye trendy razvitija intellektual'nyh transportnyh sistem. Bjulleten' Ob’edinennogo uchenogo soveta OAO «RZhD». 2018. № 4. S. 1-22 (In Russian).
- Zamyshljaev A.M. Jevoljucija cifrovogo modelirovanija. Nauka i tehnologii zheleznyh dorog. 2017. T. 1. № 1. C. 82-91 (In Russian).
- Rao D.J. Digital Twin for the Railway Network. Making Trains “Look” for Track Defects. GE Transportation – Digital Solutions, 2018. [Jelektronnyj resurs] https://www.slideshare.net/DattarajRao/digital-twin-for-the-railway-network (data obrashhenija 25.05.2021)
- Pugachev V.S., Sinicyn I.N. Teorija stohasticheskih sistem. M.: Izd-vo «Logos». 2000, 2003 [Angl. per.: Stochastic Systems. Theory and Applications. Singapore. World Scientific. 2001] (In Russian).
- Vasil'ev A.N., Tarhov D.A., Malyhina G.F. Metody sozdanija cifrovyh dvojnikov na osnove nejrosetevogo modelirovanija. Sovremennye informacionnye tehnologii i IT-obrazovanie. 2018. T. 14. № 3. S. 521-532 (In Russian).
- Sapetov M.V. Ideologija po predotkaznomu sostojaniju gruzovyh vagonov v jekspluatacii [Jelektronnyj resurs] https://opzt.ru/wp-content/uploads/2019/06/Vopros-4-Sapetov-M.V.-Ideologiya-po-predotkaznomu-sostoyaniyu-gruzovyhvagonov-v-Ekspluatatsii.pdf (data obrashhenija 25.05.2021) (In Russian).
- Zasedanie Komiteta po gruzovomu podvizhnomu sostavu 19.06.2019. http://мояколея1520.рф/new/5402/ [Jelektronnyj resurs] (data obrashhenija 25.05.2021) (In Russian).
- Mironov A.A. Nauchnye i tehnicheskie osnovy beskontaktnogo teplovogo kontrolja buks zheleznodorozhnogo podvizhnogo sostava: Avtoref. diss. … kand. tehn. nauk. Ekaterinburg. 2009 (In Russian).
- Mironov A.A., Pavljukov A.Je., Saltykov D.N. Kompleks vychislitel'nyh modelej dlja issledovanija processov kontrolja uzlov podvizhnogo sostava po infrakrasnomu izlucheniju. Mir izmerenij. 2014. № 6. S. 21–27 (In Russian).
- Mironov A.A., Obrazcov V.L., Pavljukov A.Je. Teorija i praktika beskontaktnogo teplovogo kontrolja buksovyh uzlov v poezdah. Ekaterinburg: RPF «Assorti». 2012 (In Russian).
- Gudok.ru | Oktjabr'skaja magistral'. Vypusk № 23 26.06.2020 «Men'she trevozhnyh pokazanij» https://gudok.ru/zdr/ 169/?ID=1525607 (data obrashhenija 03.06.2021) (In Russian).
- Hajkin S. Nejronnye seti: polnyj kurs. Izd. 2-e, ispr. M.-SPb: Dialektika. 2019. 1103 s. (In Russian).
- Tarhov D.A. Nejrosetevye modeli i algoritmy. M.: Radiotehnika. 2014 (In Russian).
- Druzhinina O.V., Ljudagovskaja M.A. Intellektual'nye metody dlja razrabotki i sovershenstvovanija informacionno-upravljajushhih sistem na zheleznodorozhnom transporte. Transport: nauka, tehnika, upravlenie. 2019. № 8. S. 3–12 (In Russian).
- Sinicyn I.N., Druzhinina O.V., Belousov V.V., Masina O.N., Petrov A.A. Opyt razrabotki instrumental'no-metodicheskogo obespechenija dlja reshenija zadach modelirovanija upravljaemyh dinamicheskih sistem s primeneniem tehnologij mashinnogo obuchenija i otechestvennyh programmno-apparatnyh sredstv. Nelinejnyj mir. 2019. T. 17. № 4. S. 5-19 (In Russian).
- Druzhinina O.V., Korepanov Je.R., Belousov V.V., Masina O.N., Petrov A.A. Opyt razrabotki metodov i sredstv nejrosetevogo modelirovanija nelinejnyh sistem na baze otechestvennoj vychislitel'noj platformy «Jel'brus 801-PC». Nelinejnyj mir. 2020. T. 18. № 2. S. 5-17 (In Russian).
- Druzhinina O.V., Korepanov Je.R., Belousov V.V., Masina O.N., Petrov A.A. Razvitie instrumental'nogo obespechenija otechestvennoj vychislitel'noj platformy «Jel'brus 801-PC»v zadachah nejrosetevogo modelirovanija nelinejnyh dinamicheskih sistem. Nelinejnyj mir. 2021. T. 19. № 1. S. 19-28 (In Russian).