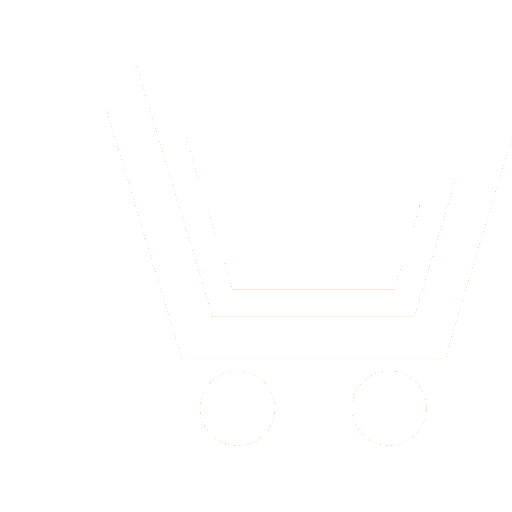
E. A. Rabchevsky – Director, JSC “SEUSLAB” (Perm, Russia)
E-mail: e.rabchevskiy@seuslab.ru
A. N. Rabchevsky – Technical Director, JSC “SEUSLAB” (Perm, Russia)
E-mail: a.rabchevskiy@seuslab.ru
V. S. Zayakin – Programmer, JSC “SEUSLAB” (Perm, Russia)
E-mail: v.zayakin@seuslab.ru
L. N. Yasnitsky – Dr.Sc. (Eng.), Professor, Department of Applied Mathematics and Informatics, Perm State National Research University (Perm, Russia)
E-mail: yasn@psu.ru
In connection with the modern success of telecommunication technologies, the problem of controlling the distribution of destructive information in social networks and involving users in socially dangerous phenomena and processes is becoming more and more urgent. The purpose of this work is to create an intelligent system that allows you to determine the role played by users of social networks in the formation and dissemination of information. Creating such a system encounters difficulties related to obtaining examples of domain behavior in a volume sufficient for high-quality training of the neural network. To solve this problem, an original method called expert is used. The essence of this method is that examples for neural network training are created by an expert who puts their knowledge of the subject area in them. Moreover, the expert sets not individual numbers that characterize the behavior of the subject area, but intervals within which they can change. The values of the domain parameters themselves are generated by the random number sensor within the limits set by the expert. The neural network created in this way can be used for solving problems of preventing the spread of destructive information in social networks and involving users in socially dangerous phenomena and processes, as well as for conducting marketing and sociological research. The expert method developed in the article is recommended to be used in the implementation of neural network projects in cases where the use of other methods of forming examples of the behavior of subject areas is difficult. In addition, this method is sometimes useful to use in combination with classical methods to strengthen the useful forces of synaptic connections of neural networks.
Rabchevsky E.A., Rabchevsky A.N., Zayakin V.S., Yasnitsky L.N. Expert method of training samples forming on the example of creating a neural network classification system for social network users. Neurocomputers. 2020. Vol. 22. No. 5. P. 54–63. DOI: 10.18127/j19998554-202005-05. (in Russian)
- Yasnitskij L.N. Intellektual'nye sistemy. Uchebnik. M.: Laboratoriya znanij. 2016. (in Russian)
- Osovskij S. Nejronnye seti dlya obrabotki informatsii: Per. s pol'skogo. M.: Finansy i statistika. 2002. (in Russian)
- Rumyantsev M.A., Yasnitskij L.N. Povyshenie kachestva obobshcheniya nejronnoj seti s pomoshch'yu dobavleniya shuma v obuchayushchuyu vyborku na primere zadachi opredeleniya prochnosti betona po sostavu vkhodyashchikh v nego komponentov. V sb.: Iskusstvennyj intellekt v reshenii aktual'nykh sotsial'nykh i ekonomicheskikh problem KhKhI veka. Ch. I. Sb. statej po materialam Chetvertoj Vseros. nauch.-praktich. konf., provodimoj v ramkakh Permskogo estestvennonauchnogo foruma «Matematika i global'nye vyzovy XXI veka». 2019. S. 57–62. (in Russian)
- Arularasan A.N., Suresh A., Seerangan K. Identification and classification of best spreader in the domain of interest over the social networks. Cluster Computing. 2019. V. 22. P. 4035–4044. DOI: 10.1007/s10586-018-2616-y.
- Doran D. On the discovery of social roles in large scale social systems. Social Network Analysis and Mining. 2015. V. 5. № 49. DOI: 10.1007/s13278-015-0290-0.
- Ruas P.H.B., Machado A.D., Silva M.C., Meireles M.R.G., Cardoso A.M.P., Zárate L.E., Nobre C.N. Identification and characterization of Facebook user profiles considering interaction aspect. Behaviour & Information Technology. 2019. V. 38. № 8. P. 858–872. DOI: 10.1080/0144929X.2019.1566498.
- Svidetel'stvo Rospatent o gos. registratsii programmy dlya EVM № 2014618208. Nejrosimulyator 5.0. F.M. Cherepanov, L.N. Yasnitskij. 12.07.2014. (in Russian)
- Yasnitskij L.N., Brzhevskaya A.S., Cherepanov F.M. O vozmozhnostyakh primeneniya metodov iskusstvennogo intellekta v sfere turizma. Servis plus. 2010. № 4. S. 111–115. (in Russian)
- Yasnitskij L.N., Vauleva S.V., Safonova D.N., Cherepanov F.M. Ispol'zovanie metodov iskusstvennogo intellekta v izuchenii lichnosti serijnykh ubijts. Kriminologicheskij zhurnal Bajkal'skogo gosudarstvennogo universiteta ekonomiki i prava. 2015. T. 9. № 3. S. 423–430. DOI: 10.17150/1996-7756.2015.9(3).423-430. (in Russian)
- Yasnitskij L.N., Gratsilev V.I., Kulyashova Yu.S., Cherepanov F.M. Vozmozhnosti modelirovaniya predraspolozhennosti k narkozavisimosti metodami iskusstvennogo intellekta. Vestnik Permskogo universiteta. Ser. «Filosofiya. Psikhologiya. Sotsiologiya». 2015. № 1 (21). S. 61–71. (in Russian)