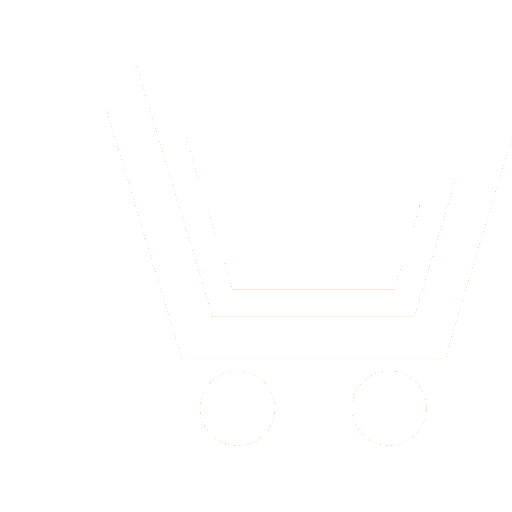
T.M. Volosatova – PhD (Eng.), Associate Professor, Department RK6, Bauman Moscow State Technical University
E-mail: tamaravol@gmail.com
T.A. Sokolova – Student, Department RK6, Bauman Moscow State Technical University
E-mail: tanya.sokolova195@gmail.com
A.Y. Spasenov – Student, Department RK6, Bauman Moscow State Technical University
E-mail: a.spasenov@bmstu.ru
K.V. Kucherov – Student, Department RK6, Bauman Moscow State Technical University
E-mail: cvkucherov@yandex.ru
This work is devoted to the development of the method of non-contact pulse wave detection to calculate the heart rate (HR).
Heart rate is an important indicator of the state of the body during exercise or illness and mental stress.
There are many ways to determine the number of heartbeats, such as: using a compact (wearable) ECG device, pulseoximetry and fitness trackers working on the technology of photoplethysmogram. It is worth noting that all of the above methods imply the presence of any sensor or detector that is on the human body during the determination of heart rate. In this regard, the ability to measure the heart rate completely contactless will be a great advantage for many areas where the value of the pulse is essential, such as in medical diagnosis, monitoring the condition of workers, motorists, etc.
It is shown that with the help of the data obtained from the video stream it is possible to determine the heart rate of a person with a sufficiently high degree of reliability. The proposed approach can be used for remote assessment of the psycho-emotional state of a person with high accuracy. The results have shown high accuracy of pulse detection using a high-resolution webcam. Such results were achieved by using an ensemble of convolutional neural networks for facial recognition and receiving a signal from key segments of the face, the most saturated networks of capillary vessels. The subsequent use of the wavelet transforms signals from the areas of interest allowed us to identify the signal is well correlated with the reference one.
Volosatova T.M., Sokolova T.A., Spasenov A.Y., Kucherov K.V. Method of contactless detection of the heart pulse wave. Neurocomputers. 2020. V. 22. № 2. P. 35–42. DOI: 10.18127/j19998554-202002-03
- Rahman H., Ahmed M. U., Begum S. Non-Contact Physiological Parameters Extraction Using Camera. The 1st Workshop on Embedded Sensor Systems for Health through Internet of Things, School of Innovation, Design and Engineering. Mälardalens University, Västerås, Sweden, 2015.
- Rahman H., Ahmed M. U., Begum S. Vision-Based Remote Heart Rate Variability Monitoring using Camera. in: Internet of Things (IoT) Technologies for HealthCare, Västerås, Sweden, Springer, 2016. P.10-18.
- Rahman H., Ahmed M. U., Begum S. Non-Contact Heart Rate Monitoring Using Lab Color Space. 13th International Conference on Wearable Micro and Nano Technologies for Personalized Health, 2016.
- Shao D., Liu C., Tsow F., Yang Y., Du Z., Iriya R. Noncontact Monitoring of Blood Oxygen Saturation Using Camera and DualWavelength Imaging System. IEEE Transactions on Biomedical Engineering. 2016. V. 63. P. 1091- 1098.
- Al-Naji A. Computer Vision for Remote Cardiorespiratory Monitoring. Middle Technical University, 2018.
- Alghoul K., Alharthi S., Osman H. A., Saddik A. E. Heart Rate Variability Extraction From Videos Signals: ICA vs. EVM Comparison. IEEE. 2017. V. 5. P. 4711-4719.
- Al-Naji A., Gibson K., Lee S., Chahl J. Monitoring of Cardiorespiratory Signal: Principles of Remote Measurements and Review of Methods. IEEE. 2017. V. 5. P. 15776-15790.
- Rahman H. Real Time Heart Rate Monitoring from Facial RGB Color Video Using Webcam. The 29th Annual Workshop of the Swedish Artificial Intelligence Society, 2016.
- Viola P., Jones M. J. Robust Real-Time Face Detection. International Journal of Computer Vision. 2001. V. 57. P. 137–154.
- Shu C., Ding X., Fang C. Histogram of the oriented gradient for face recognition. Tsinghua Science and Technology. 2011. V. 16. P. 216-224.
- Liy J., Wangz Y., Wangz C., Taiz Y., Qiany J., Yangy J., Wangz C., Liz J., Huang F. DSFD: Dual Shot Face Detector [Elektronny resurs]. – URL: https://arxiv.org/abs/1810.10220v3 (data obrascheniya: 26.05.2019).
- Simonyan K., Zisserman A. Very Deep Convolutional Networks For Large-Scale Image Recognition [Elektronny resurs]. – URL:https://arxiv.org/abs/1409.1556 (data obrascheniya: 25.05.2019).
- Liu W., Anguelov D., Erhan D., Szegedy C., Reed S., Fu1 C., Berg A. C. SSD: Single Shot MultiBox Detector. [Elektronny resurs]. – URL:https://arxiv.org/abs/1512.02325 (data obrascheniya: 25.05.2019)).
- Riedel R. B. Infrared Identification of Faces and Body Parts. Biometrics Springer. 2006. P.191-212.
- Raspberry PI [Elektronny resurs]. – URL: https://www.raspberrypi.org/ (data obrascheniya: 25.05.2019).
- ImageNet [Elektronny resurs]. – URL: http://www.image-net.org/ (data obrascheniya: 25.05.2019).
- Volosatova T.M., Spasenov A.I., Logunova A.O. Automated ECG Analysis and Interpretation System. Radio Engineering. 2016. V. 1. P. 1-18.