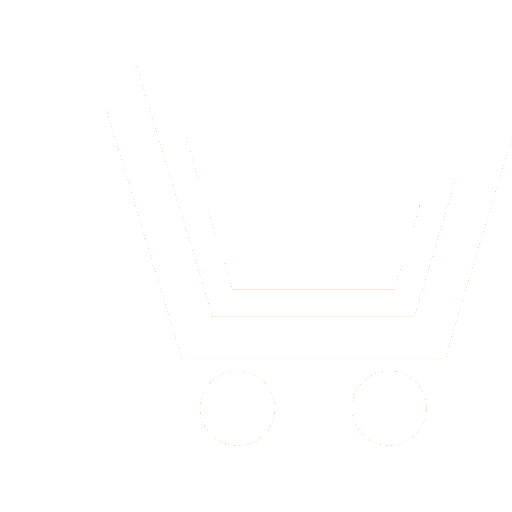
E.V. Kotelnikov – Ph. D. (Eng.), Associate Professor, Department of Applied Mathematics and Computer Science, Vyatka State University
E-mail: kotelnikov.ev@gmail.com
V.R. Milov – Dr. Sc. (Eng.), Professor, Head of Department «Electronics and Computers Networks», Nizhny Novgorod State Technical University n.a. R.E. Alekseev
E-mail: vladimir.milov@gmail.com
Automatic opinion mining in texts is connected with research of opinions, emotions, appraisals towards products, services, organizations, individuals, events, topics, and their attributes. The task of opinion mining contains three subtasks: identification of the object, the author and the time of opinion; recognition of the sentiment of opinion; determination of the aspects of object to which the opinion is expressed. The main subtask is the second one. The sentiment is the degree of opinion emotionality and it can be positive, negative, neutral or contradictory.
In the paper the TextJSM methodology of intellectual opinion mining in texts is proposed. To apply the TextJSM methodology, the linguistic resources are needed – annotated text corpus and sentiment lexicon. This methodology is based on the conception of JSM-reasoning and consists of plausible reasoning methods, such as induction, analogy and abduction, and also the text preprocessing method.
At the preprocessing method the input texts are converted to convenient way of the representation with the help of text segmentation and morphological parser. Also, the post-morphological analysis is carried out, including the removal of rare and stop words.
In the induction method the hypotheses set is generated based on the annotated text corpus. Hypotheses are the possible reasons for the presence or absence of sentiment in the training texts.
In the analogy method the sentiment of new texts is predicted with the help of the set of generated hypotheses and sentiment lexicon.
The method of abduction is required to verify the ability of the generated hypotheses to explain the training data, and to accept the hypotheses if the explanation is successful.
To apply the TextJSM methodology, the linguistic resources are needed – annotated text corpus and sentiment lexicon. The example of application of the TextJSM methodology for opinion mining of movie review is considered.
The proposed methodology makes it possible to achieve high classification performance, strict validity of the results and transparency of the solution process.
- Liu B. Sentiment Analysis and Opinion Mining. Synthesis Lectures on Human Language Technologies. Morgan & Claypool Publishers. 2012. V. 5(1).
- Yue L., Chen W., Li X., Zuo W., Yin M. A survey of sentiment analysis in social media. Knowledge and Information Systems. 2019. V. 60(2). P. 617–663.
- Kotelnikov E.V., Milov V.R. Issledovanie soobshhenij polzovatelej socialnyh media na osnove analiza reljacionnyh ponjatij. Nejrokompjutery: razrabotka, primenenie. 2017. № 7. P. 58–65. [in Russian]
- Sun S., Luo C., Chen J. A review of natural language processing techniques for opinion mining systems. Information Fusion. 2017. V. 36. P. 10–25.
- Goyal A., Gupta V., Kumar M. Recent Named Entity Recognition and Classification techniques: A systematic review. Computer Science Review. 2018. V. 29. P. 21–43.
- Schouten K., Frasincar F. Survey on Aspect-Level Sentiment Analysis. IEEE Transactions on Knowledge and Data Engineering. 2016. V. 28. P. 813–830.
- Finn V.K. Epistemologicheskie osnovanija DSM-metoda. Ch. I. NTI. Ser. 2. Informacionnye processy i sistemy. 2013. № 9. P. 1–29. [in Russian]
- Kotelnikov E.V. Povyshenie bystrodejstvija DSM metoda v zadachah obrabotki tekstovoj informacii. Trudy Chetyrnadcatoj nacionalnoj konferencii po iskusstvennomu intellektu s mezhdunarodnym uchastiem KII-2014. 2014. T. 2. P. 274–282. [in Russian]
- Kotelnikov E.V. Metod analiza tonalnosti tekstov TextJSM. Nauchno-tehnicheskaja informacija. Ser. 2. 2018. № 2. P. 8–20. [in Russian]
- Kotelnikov E.V., Peskisheva T.A., Kotelnikova A.V., Razova E.V. A comparative study of publicly available Russian sentiment lexicons. Artificial Intelligence and Natural Language. AINL 2018. Communications in Computer and Information Science. 2018. V. 930. P. 139–151.
- Andrews S. Making use of empty intersections to improve the performance of CbO-type algorithms. Proceedings of the 14th International Conference on Formal Concept Analysis. Springer. 2017. P. 56–71.
- Kotelnikov E.V. Funkcija ocenki informativnosti gipotez dlja analiza tonalnosti tekstov na osnove DSM-metoda. Fundamentalnye issledovanija. 2014. № 11(10). P. 2150–2154. [in Russian]