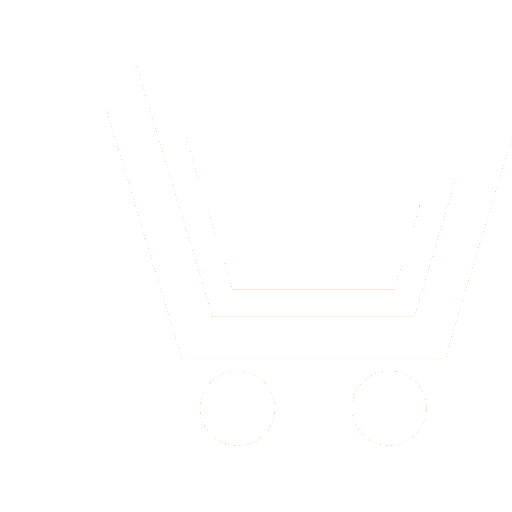
Yu.Yu. Petrunin – Dr.Sc. (Phil.), Professor, Head of the Department of Mathematical Methods and Information Technologies in the Management; Head of the Center of Big Data Analysis, Faculty of Public Administration, Lomonosov Moscow State University
E-mail: Petrunin@spa.msu.ru
Yu.A. Siluyanova – Post-graduate Student, Department of Sociology, Faculty of Public Administration, Lomonosov Moscow State University
E-mail: zernovaju@gmail.com
M.G. Myagkov – Dr.Sc. (Phys.-Math.), Professor, University of Oregon; Leading Research Scientist, Department of Mathematical Methods and Information Technologies in the Management; Scientific Supervisor of the Center for Big Data Analysis in Social Sciences, Faculty of Public Administration, Lomonosov Moscow State University
E-mail: myagkov@uoregon.edu
I.V. Kozitsin – Post-graduate Student, Moscow Institute of Physics and Technology
E-mail: kozitsin.ivan@mail.ru
S.D. Osipov − Student, Moscow Institute of Physics and Technology
E-mail: Petrunin@spa.msu.ru
Problem statement: on the eve of the presidential elections in 2018, specialists of the Center for Analysis of Big Data in Social Sciences of the Faculty of Public Administration of the Lomonosov Moscow State University conducted a large-scale study, based on open data available in the social network Vkontakte. Distinctive features of Big Data in addition to huge amounts of information, is its diversity, heterogeneity, unstructuredness and a large amount of noise. Working with such data sets, it is important to clean and organize them a way that they become suitable for further effective application in solving specific tasks.
The aim of the work is to identify patterns of electoral behavior of different groups of voters based on open data from the VKontakte social network. To build reliable prognostic and analytical models, it is necessary to select tools that can overcome distortions. Such tools include combining Big Data technologies, statistical methods, and neural network algorithms.
Results: Users, participating in online-voting, were put in a separate sample in order to use the methods of statistical analysis to reveal the peculiarities of the electorate of each candidate. The study included the results of preliminary voting for Putin, Grudinin, Zhirinovsky, Sobchak, Yavlinsky.
The peculiarity of this study is the use of Big Data. The most important conclusions and observations that we made. «Trolls», «clones» and «partisans» can greatly distort the result of research based on data from social networks. Women demonstrate much less political activity than men. Of all the candidates, women from the sample most often choose Putin. Of all candidates, men from the sample most often vote for Grudinin. Generation Z (born 1990−2000) − the most politically active of the presented VKontakte, youth − the largest group of all candidates. Generation Y (born 1980−1990) manifests itself much weaker, the only candidate who received active support of this group is Grudinin. Most often voters adhere to liberal and «moderate» views. Grudinin and Putin are the most popular candidates.
Practical significance: the combination of the methods of classical statistics with the neural network model allowed us to carry out a full and meaningful analysis of the electoral behavior of various groups, despite significant distortions in the data and a small number of variables. A successful combination of analytical tools helped to identify interesting trends and patterns, as well as to form an adequate forecast of how users will vote.
Our conclusions were confirmed on the basis of voting results, which once again proves the effectiveness of the presented analytical model. Conclusions about the peculiarities of the electorate of each of the candidates give us useful information about which social groups the candidates are based on. The results of the research can be used for further in-depth study of the electoral behavior of Russ
- Petrunin YU.YU., Zernova YU.A. Statisticheskie i nejrosetevye metody issledovaniya politicheskoj situacii vo Francii na primere regional'nyh vyborov 1998 i 2004 godov. Gosudarstvennoe upravlenie. Elektronnyj vestnik. 2008. № 14 (In Russia).
- Petrunin YU.YU., Zernova YU.A. Statisticheskie i nejrosetevye metody prognozirovaniya elektoral'nogo povedeniya na primere prezidentskih vyborov vo Francii 2007 g. Nejrokomp'yutery: razrabotka, primenenie. 2011. № 1. S. 11−24 (In Russia).
- Petrunin YU.YU. Issledovanie elektoral'nogo povedeniya: samoorganizuyushchiesya karty Kohonena versus statistiches- kogo analiza. Vestnik Moskovskogo universiteta. Seriya 21: Upravlenie (gosudarstvo i obshchestvo). 2009. № 3. S. 45−55 (In Russia).
- Petrunin YU.YU. Nejrokomp'yuting: mezhdu naukoj i lzhenaukoj. Nejrokomp'yutery: razrabotka, primenenie. 2015. № 8. S. 52−64 (In Russia).
- Petrunin YU.YU. Nejrokomp'yuternaya paradigma i obshchestvo. M.: Izdatel'stvo MGU. 2012. 288 s.
- Savel'ev A.V. Rasshirenie ponyatiya nejrokomp'yutera i nejrokomp'yutinga. Nejrokomp'yutery: razrabotka, primenenie. 2013. № 7. S. 58-68 (In Russia).
- Savel'ev A.V. Nejrosociometodologiya problemy dialoga mezhdu nejrobiologiej i nejromodelirovaniem. Nejrokomp'yutery: razrabotka, primenenie. 2011. № 1. S. 47-63 (In Russia).
- Petrunin YU.YU. Iskusstvennyj intellekt i metodologicheskie voprosy upravleniya znaniyami. Filosofskie nauki. 2016. № 8. S. 67−74 (In Russia).
- Petrunin YU.YU. Informacionnye tekhnologii analiza dannyh: Ucheb. posobie. M.: Izdatel'stvo MGU. 2008. 292 s. (In Russia).
- Kohonen T. Samoorganizuyushchiesya karty. M.: BINOM. 2008. 655 s. (In Russia).