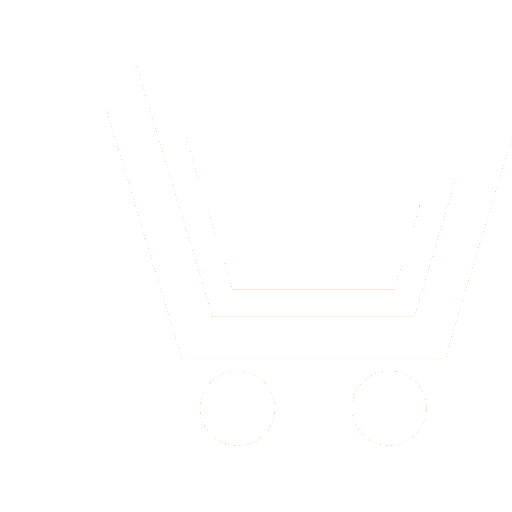
N. S. Konnova – Ph.D. (Eng.), Associate Professor of Bauman Moscow State Technical University
M. A. Basarab – Dr.Sc. (Phys.-Math.), Head of Department of Information Security of Bauman Moscow State Technical University
E-mail: bmic@mail.ru
D. A. Basarab – Ph.D. (Med.), Head of Cardiovascular Department of St. Ioasaf's Belgorod Regional Hospital (Belgorod)
D. V. Minin – Post-graduate Student, Bauman Moscow State Technical University
V. M. Achildiev – Ph.D. (Eng.), Chief Designer, SPU Geophizika-NV (Moscow)
V. A. Soldatenkov – Dr.Sc. (Eng.), General Director of SPU Geophizika-NV (Moscow)
N. A. Bedro – Head of Department, Deputy Chief Designer, SPU Geophizika-NV (Moscow)
Yu. K. Gruzevich – Ph.D. (Eng.), Deputy General Director for Science, SPU Geophizika-NV (Moscow)
Yu. N. Evseeva – SPU Geophizika-NV (Moscow)
A. D. Levkovich – Ph.D. (Eng.), SPU Geophizika-NV (Moscow)
M. N. Komarova – Leading Engineer, SPU Geophizika-NV (Moscow)
There are presented the concept and implementation of a decision support system in cardiology on the basis of various recorded indices of the human cardiovascular system: electro-, seismic cardiography, flowmetry, etc. Analysis of developments in the field of machine learning methods application to decision support problems in cardiology has been given: the achievable values of the cardiovascular system states classification accuracy have been considered when using neural networks of various architectures and various learning algorithms.
The focus of the article is on the possibilities of application and preparation of seismic cardiography data for use in the diagnostics. Analysis of the seismic cardiography data parameters variability (from person to person and in different states of one person) has been given, in particular with respect to the characteristic points taken into account in phase analysis. The results and discussion of spectra, noise characteristics, phase portraits, autocorrelation functions of signals of angular rates and apparent accelerations along three axes have been presented. The methods of digital signal processing which are most suitable for use in diagnostics according to the SCG, including using machine deep learning, have been identified.
- Konnova N.S., Basarab M.A. Application of neural networks in cardiovascular decision support systems // Proc. of 7th International Conference on Advances in Computing, Electronics and Communication (ACEC 2018). 2018. P. 73–78. DOI: 10.15224/9781-63248-157-3-27
- Konnova N.S., Basarab M.A., Basarab D.A. Image processing using artificial intelligence methods in cardiovascular decision support systems // Proc. SPIE 10836. 2018 International Conference on Image and Video Processing, and Artificial Intelligence. DOI: 10.1117/12.2515343
- Konnova N.S., Basarab M.A. Identification of functional states of the cardiovascular system according to flowmetry data using machine learning methods // Proc. of 11th International Symposium on Computational Intelligence and Design (ISCID 2018). 2018. In press.
- Konnova N.S., Basarab M.A., Basarab D.A. Tsifrovaya obrabotka signalov v doplerovskoj floumetrii. Metody i algoritmy. Monografiya. Lambert Academic Publishing. 2018.
- Basarab M.A., Konnova N.S., Mitrokhin V.N., Basarab D.A., Matsievskij D.D. Diagnostika sostoyanij serdechno-sosudistoj sistemy metodami nelinejnoj tsifrovoj obrabotki signalov doplerovskikh izmeritelej skorosti krovotoka // Biomeditsinskaya radioelektronika. 2017. № 5. S. 3–11.
- Konnova N.S., Basarab M.A., Basarab D.A., Matsievskij D.D. Fazovyj analiz krivykh skorosti krovotoka po dannym vremennykh ryadov signala doplerovskoj floumetrii // Biomeditsinskaya radioelektronika. 2014. № 11. S. 16–29.
- Achildiev V.M., Soldatenkov V.A., Bedro N.A., Gruzevich Yu.K., Evseeva Yu.N., Levkovich A.D., Basarab M.A., Konnova N.S. Cardioseismometer unit based on micromechanical sensors // Proc. of 25th Anniversary Saint Petersburg International Conference on Integrated Navigation Systems. 2018. P. 272–281.
- Konnova N.S. Programmnyj kompleks obrabotki vremennykh ryadov pri pomoshchi metodov nelinejnoj dinamiki i vejvlet-analiza // Svid. o gos. reg. progr. dlya EVM № 2014661986 RF. Opubl. 20.12.2014.
- Konnova N.S., Basarab M.A. Cardiovascular states identification using machine learning techniques based on artificial neural networks. 2018. Unpublished.
- Yan H., Jiang Y., Zheng J., Peng Ch., Li Q. A multilayer perceptron-based medical decision support system for heart disease diagnosis // Expert Systems with Applications. 2006. V. 30. № 2. P. 272–281. DOI: 10.1016/j.eswa.2005.07.022
- Das R., Turkoglu I., Sengur A. Effective diagnosis of heart disease through neural networks ensembles // Expert Systems with Applications. 2008. V. 36. № 4. P. 7675–7680. DOI:10.1016/j.eswa.2008.09.013
- Kurt I., Ture M., Kurum A.T. Comparing performances of logistic regression, classification and regression tree, and neural networks for predicting coronary artery disease // Expert Systems with Applications. 2008. V. 34. № 1. P. 366–374. DOI: 10.1016/j.eswa.2006.09.004
- Jadhav S.M., Nalbalwar S.L., Ghatol A.A. Artificial neural network models based cardiac arrhythmia disease diagnosis from ECG signal data // International Journal of Computer Applications. 2012. V. 44. № 15. DOI:10.1109/ICEIE.2010.5559887
- Xiong Z., Stiles M.K., Zhao J. Robust ECG signal classification for detection of atrial fibrillation using a novel neural network // Computing in Cardiology.2017. V. 44. DOI:10.22489/CinC.2017.066-138
- Acharya U.R., Fujita H., Lih O.S., Hagiwara Y., Tan J.H., Adam M. Automated detection of arrhythmias using different intervals of tachycardia ECG segments with convolutional neural network // Information Sciences. 2017. V. 405. P. 81–90. DOI: 10.1016/ j.ins.2017.04.012
- Acharya U.R., Fujita H., Oh S.L., Hagiwara Y., Tan J.H., Adam M. Application of deep convolutional neural network for automated detection of myocardial infarction using ECG signals // Information Sciences. 2017. V. 415. P. 190–198. DOI: 10.1016/ j.ins.2017.06.027
- Acharya U.R., Fujita H., Lih O.S., Adam M., Tan J.H., Chua C.K. Automated detection of coronary artery disease using different durations of ECG segments with convolutional neural network // Knowledge-Based Systems. 2017. V. 132. P. 62–71. DOI: 10.1016/j.knosys.2017.06.003
- Tan J.H., Hagiwara Yu., Pang W., Lim I., Lih O.S., Adam M., Tan R.S., Chen M., Acharya U.R. Application of stacked convolutional and long short-term memory network for accurate identification of CAD ECG signals // Computers in Biology and Medicine. 2018. V. 94. P. 19–26. DOI: 10.1016/J.COMPBIOMED.2017.12.023
- Sejsmokardiografiya [Elektronnyj resurs] // Bol'shaya meditsinskaya entsiklopediya. URL: http://bme.org/index.php/ SEJSMOKARDIOGRAFIYa (data obrashcheniya: 28.10.2018).
- Wang L., Huang K., Sun K., Wang W., Tian Ch., Xie L., Gu Q. Unlock with your heart: heartbeat-based authentication on commercial mobile phones // Proc. ACM Interact. Mob. Wearable Ubiquitous Technol. 2018. V. 2. № 3.
- Zhvalevskij O.V. Kontseptual'naya model' matematicheskoj obrabotki tenzotremorogramm // Sb. trudov mezhdunar. nauch. konf. «Matematicheskie metody v tekhnike i tekhnologiyakh». V 12-ti tomakh. T. 8 / Pod obshch. red. A.A. Bol'shakova. SPb.: Izd-vo Politekhn. un-ta. 2018. S. 44–50.