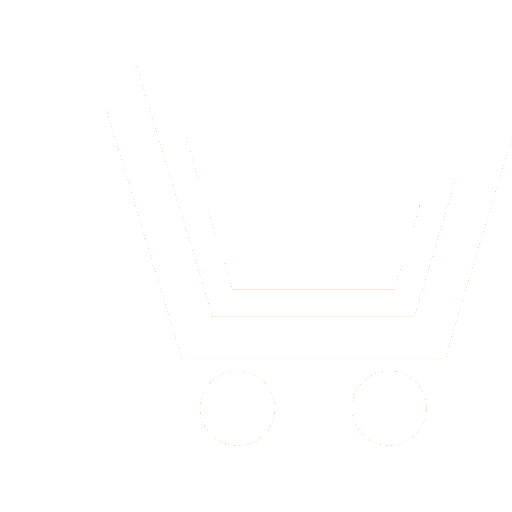
O.I. Garanin – Post-graduate Student, National Research University “MPE” (Moscow)
E-mail: hedgehog91@mail.ru
In this article we analyze the multiscale model for detecting visual objects in a convolutional neural network, which provides a compromise between the accuracy and speed of their detection. We propose a tuning method of multiscale model for detecting visual objects in a convolutional neural network. This method differs from the existing one by choosing the sizes of the default boxes for a particular training dataset using the k-means clustering method and calculating the empirical receptive field. In the article has been proved experimentally that the proposed method allows increasing the accuracy of detecting visual objects in a convolutional neural network.
- Krizhevsky A., Sutskever I., Hinton G. Imagenet classification with deep convolutional neural networks // Proceedings of the Neural Information Processing Systems conference. NIPS. 2012. P. 1–9.
- Sánchez, J., Perronnin, F. High-dimensional signature compression for large-scale image classification // Computer Vision and Pattern Recognition. CVPR. 2011. P. 1665–1672.
- Ren S., He K., Girshick R., Sun J. Faster R-CNN: Towards real-time object detection with region proposal networks // Proceedings of the Neural Information Processing Systems conference. NIPS. 2015.
- Redmon J., Divvala S., Girshick R., Farhadi A. You only look once: Unified, real-time object detection // Computer Vision and Pattern Recognition. CVPR. 2016.
- Liu W., Anguelov D., Erhan D., Szegedy C., Reed S. E. SSD: single shot multibox detector // CoRR. 2015.
- Simonyan K., Zisserman A. Very deep convolutional networks for large-scale image recognition // Proceedings of the Neural Information Processing Systems conference. NIPS. 2015.
- Garanin O.I. Sposob vy'deleniya e'mpiricheskogo reczeptivnogo polya svertochnoj nejronnoj seti // Nejrokomp'yutery' razrabotka i primenenie. 2017. № 3.
- Russakovsky O., Deng J., Su H., Krause J., Satheesh S., Ma S., Huang Z., Karpathy A., Khosla A., Bernstein M., Berg A.C., Fei-Fei L. Imagenet large scale visual recognition challenge // IJCV. 2015.
- Everingham M., Van Gool L., Williams C., Winn J., Zisserman A. The pascal visual object classes (voc) challenge // International journal of computer vision. 2010. № 88(2). P. 303–338.
- Everingham M., Eslami S. M. A., Van Gool L., Williams C., Winn J., Zisserman A. The Pascal visual object classes challenge: A retrospective // IJCV. 2015. P. 98–136.