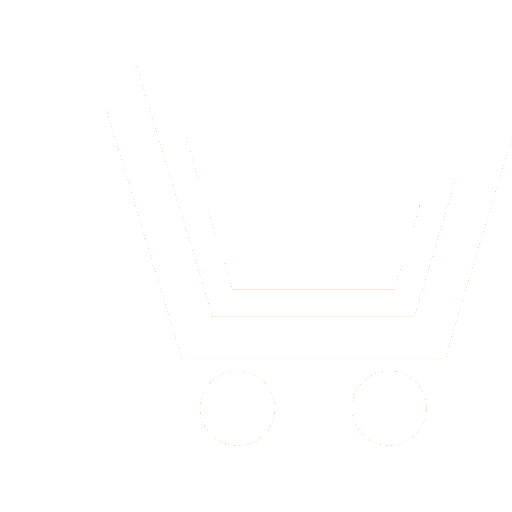
V.A. Skolota – Post-graduate Student, Department of Electronics and Electrical Engineering, Novosibirsk State Technical University
E-mail: sva008@yandex.ru
I.A. Belova – Post-graduate Student, Department of Electronics and Electrical Engineering, Novosibirsk State Technical University
E-mail: ira.belowa@gmail.com
M.V. Martinovich – Ph.D. (Eng.), Associate Professor, Department of Electronics and Electrical Engineering, Novosibirsk State Technical University
E-mail: martinovich_m@mail.ru
The article proposes an analog hardware implementation of an artificial neuron, the nonlinearity of the transformations being maintained not at the circuitry level, but due to the nonlinearity of the physical processes occurring in CMOS transistors, which reduces the hardware costs of up to one transistor per synapse and three transistors per neuron body, increasing its sensitivity And reducing the accuracy of work. A model experiment shows the effect of increasing the stability of a neural network implementation of the same function with respect to a single-neural one.
- Widrow B., Rumelhart D.E., and Lehr M.A. Neural networks: applications in industry, business and science. Communications ofthe ACM. 1994. V. 37. № 3. P. 93–105.
- Muthuramalingam A., Himavathi S., and Srinivasan E. Neuralnetwork implementation using FPGA: issues and application // TheInternational Journal of Information Technology. 2008. V. 4. № 2. P. 86–92.
- Blumer A.A., Ehrenfeucht D.H., Warmuth M.K. Learnability and the Vapnik-Chervonenkis dimension // J. Association for Computing Machinery. 1989. V. 36. P. 929–965.
- Moerland P., Fiesler E. Neural network adaptations to hardware implementations // Handbook of Neural Computation. 1997. V. 1. P. 2.
- Aparin V., Levin J.A. Methods and systems for CMOS implementation of neuron synapse // Apr. 8 2014, US Patent 8,694,452.