350 rub
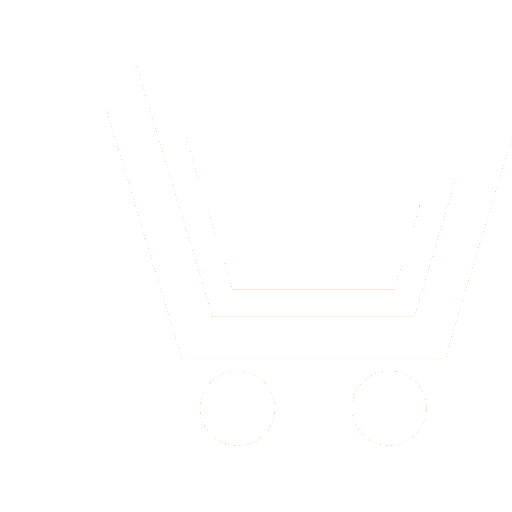
Journal Neurocomputers №9 for 2016 г.
Article in number:
The neural network classification problem approach based on the relation-features
Keywords:
classification
categorization
clustering
classification problems
neural network classification problem approaches
features of classifying instances
relations on the sets of the features of instances
Authors:
K.P. Korshunova - Post-graduate Student, The Branch of National Research University «Moscow Power Engineering Institute» in Smolensk. E-mail: ksenya-kor@mail.ru
Abstract:
The research is devoted to developing classification problem approaches for complicated objects. The target of the research is increasing the quality of classification systems (pattern recognition systems). By complicated object we mean object that have a few features yielded little information and can be considered as complex system with its features as subsystems. A concise classification problem statement for complicated objects based on the relation-features is defined. The approaches are based on the relations (with a different number of \"places\") on the sets of the probabilistic features of instances. We introduced the concept of "relation-features": thecartesian products of dyads or triplets of the probabilistic features of instances (this is dyadic or binary relation and triadic or ternary relation respectively). We added steps of features preconditioning during the stages of training and classifying (solving the clustering subproblem) to form these "relation-features". Then we can use them in any classification algorithms. The algorithms of using the approach and decision support tools to solve classification problems in medicine are developed. Neural network procedures and statistical classification methods are applied and compared with breast cancer diagnostics using features we got from some medical tests. Experimental results show that both the neural network and statistical classification problem approaches based on the "relation-features" allows the quality of classification problem solution (precision and recall) increase.
Pages: 57-63
References
- Tu Dzh., Gonsales R. Principy raspoznavanija obrazov. M.: Mir. 1978. 412 s.
- Bongard M.M. Problema uznavanija. M.: Nauka. 1967. 321 s.
- Peregudov F.I., Tarasenko F.P. Vvedenie v sistemnyjj analiz. M.: Vysshaja shkola. 1989. 367 s.
- Ljamec L.L. Podkhod k formalnomu opisaniju obektov v zadachakh raspoznavanija na osnove principa sistemnosti // Matematicheskaja morfologija. EHlektronnyjj matematicheskijj i mediko-biologicheskijj zhurnal. 2014. T. 13. № 2.
- Korshunova K.P., Ljamec L.L. Postanovka zadachi klassifikacii obektov po mnozhestvam verojatnostnykh priznakov i otnoshenijam mezhdu nimi // Sb. trudov XI Mezhdunar. nauch.-tekhn. konf. studentov i aspirantov «Informacionnye tekhnologii, ehnergetika i ehkonomika». 2014. T. 1. S. 171-173.
- CHizhkov A.V., Seitova S.V. Klassifikacija nejjrosetevykh arkhitektur // Izv. JUzhnogo federalnogo universiteta. Ser.Tekhnicheskie nauki. 2009. T. 93. Vyp. № 4. S. 220-225.
- Rutkovskaja D., Pilinskijj M., Rutkovskijj L. Nejjronnye seti, geneticheskie algoritmy i nechetkie sistemy. M.: Gorjachaja linija - Telekom. 2006. 452 s.
- SHrejjder JU.A. Ravenstvo. Skhodstvo. Porjadok. Moskva: Nauka. 1971. 256 s.
- Coates A., Andrew Y. Ng. Learning feature representations with K-means. Stanford University. 2012.
- Korshunova K.P., Borisov V.V. Reshenie zadachi klassifikacii na osnove ucheta binarnykh i ternarnykh otnoshenijj mezhdu priznakami // Sb. trudov IV Mezhdunar. nauch.-tekhn. konf. «EHnergetika, informatika, innovacii-2014». 2014. T. 1. S. 195-201.
- Abrosimov S.JU. Proverka gipotezy o vozmozhnosti identifikacii stromy biologicheskikh tkanejj v norme, pri predopukholevykh i opukholevykh processakh, nauchnyjj otchet po provedennomu nauchnomu issledovaniju. Smolensk. 2006.
- Softmax function // Wikipedia, the free encyclopedia. 2015. URL: http://en.wikipedia.org/wiki/Softmax_function (data obrashhenija: 01.06.2015).
- Osovskijj S. Nejjronnye seti dlja obrabotki informacii. M.: Finansy i statistika. 2002. 344 s.
- Manning C., Raghavan P., Schutze H. An Introduction to Information Retrieval. Cambridge: Cambridge UniversityPress. 2009.
- Vald A. Posledovatelnyjj analiz. M.: Fizmatgiz. 1960. 328 s.
- Korshunova K.P. Sposob reshenija zadachi klassifikacii slozhnykh obektov na osnove ucheta otnoshenijj mezhdu verojatnostnymi priznakami // Informacionnye tekhnologii i sistemy 2014 (ITS 2014): Materialy mezhdunar. nauch. konf. 2014. S. 266-267.