350 rub
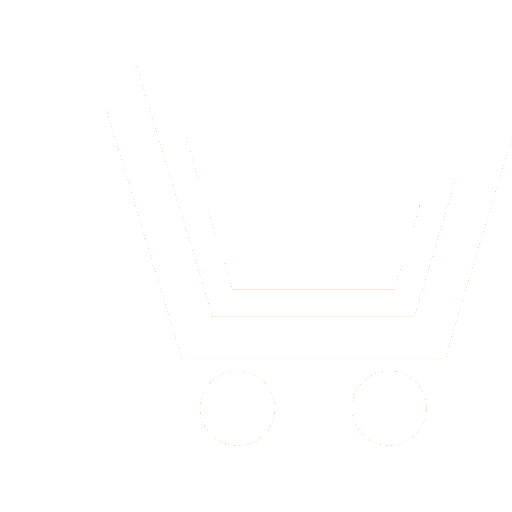
Journal Neurocomputers №7 for 2016 г.
Article in number:
The genetic learning algorithm of fuzzy inference system
Authors:
M.I. Zernov - Dr.Sc. (Mil.), Professor, Department of Computer Engineering, Branch of National Research University «Moscow Power Engineering Institute» in Smolensk. E-mail: zmmioml@yandex.ru
V.I. Sak-Sakovsky - Dr.Sc. (Mil.), Professor, Department of Computer Engineering, Branch of National Research University «Moscow Power Engineering Institute» in Smolensk. E-mail: zmmioml@yandex.ru
A.V. Senkov - Ph.D.(Eng.), Associate Professor, Department of Computer Engineering, Branch of National Research University «Moscow Power Engineering Institute» in Smolensk. E-mail: a.v.senkov@mail.ru
D.S. Bukachev - Ph.D. (Phys.-Math.), Associate Professor, Department of Mathematics and Computer Science, Smolensk State University.
Abstract:
Optimization problems have an important place in various areas of management, including support systems adopted-making. In the traditional approach to solve optimization problems using a variety of methods of mathematical programming, but the results of solving optimization problems are largely dependent on the accuracy of the source data. In the wild face, bringing-cerned with the decision maker usually has to deal with inaccurate data, and classic algorithms for solving optimization problems are not always effective.
Inaccurate raw data - not the only problem in the real world optimization problems. Often, it is difficult to form a very target-function-hand, and so that it is consistent with the preferences of decision makers (decision maker).
To solve these problems is proposed to calculate the value of the objective function using the fuzzy inference algorithm, as a universal approximator, and adjust the parameters of the algorithm taking into account the preferences of the decision maker in the process of learning.
The most common algorithms for fuzzy inference algorithms include Mamdani and Sugeno 0-th order.
In this paper we propose a learning algorithm fuzzy production models using elements of genetic algorithms, compares the training of fuzzy production model experiment and assesses each of the proposed fuzzy inference algorithm in terms of speed and efficiency of training.
Pages: 57-60
References
- Perepelica V.A., Tebueva F.B. Diskretnaja optimizacija i modelirovanie v uslovijakh neopredelennosti dannykh. M.: Izdatelstvo «Akademija estestvoznanija». 2007. 151 s.
- Borisov V.V., Kruglov V.V., Fedulov A.S. Nechetkie modeli i seti. Izd. 2-e stereotip. M.: Gorjachaja linija-Telekom. 2012. 284 s.
- Rutkovskaja D., Pilinskijj M., Rutkovskijj L. Nejjronnye seti, geneticheskie algoritmy i nechetkie sistemy. M.: Gorjachaja linija-Telekom. 2006. 452 s.
- Borisov V.V., Fedulov A.S., Zernov M.M. Osnovy nechetkogo logicheskogo vyvoda. Serija «Osnovy nechetkojj matematiki». Kniga 4. Uchebnoe posobie dlja vuzov. M.: Gorjachaja linija-Telekom. 2014. 122 s.
- Kruglov V.V. Sravnenie algoritmov Mamdani i Sugehno v zadache approksimacii funkcii // Nejjrokompjutery: razrabotka, primenenie. 2003. № 5. S. 34-38.