350 rub
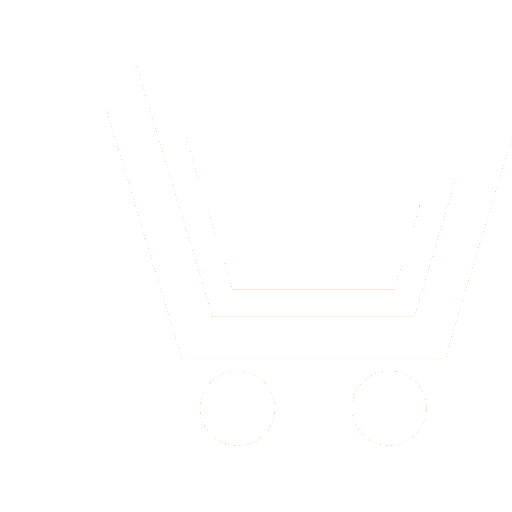
Journal Neurocomputers №4 for 2016 г.
Article in number:
Designing of system the unstructured speech information analysis
Keywords:
speech analysis system
speech recognition technology
human-machine interface
linguistic processor
Authors:
M.P. Farkhadov - Dr. Sc. (Eng.), Head of Laboratory. «Automated queuing systems and signal processing», V.A. Trapeznikov Institute of Control Sciences of RAS (Moscow). E-mail: mais@ipu.ru
S.V. Vaskovsky - Ph.D. (Eng.), Senior Research Scientist, V.A. Trapeznikov Institute of Control Sciences of RAS (Moscow). E-mail: v63v@yandex.ru
V.A. Smirnov - Applicant, V.A. Trapeznikov Institute of Control Sciences of RAS (Moscow). E-mail: v63v@yandex.ru
M.E. Farkhadova - Ph.D. (Philol.) Senior Lecturer, Russian People\'s Friendship University (Moscow). E-mail: muhabbat-2007@mail.ru
Abstract:
In this paper we consider the design of the applied system for the analysis of the unstructured speech data by using the software speech analytics package «ANALYZE» as example, with a key focus on the implementation of its linguistic component and a human-machine interface. We describe the solution architecture and modules interaction logic, the parameters of the key scientific modules and the human-machine interface of the system. As a conclusion, we provide the results of applying the system as a way to improve the quality of the organization of queueing and information services systems.
At present modern storage systems exist, as well as automated data collection implementations collecting data from virtually all sources, including speech data. Obviously, the manual processing and analysis of the modern speech data flow is a complex and time-consuming task. Therefore it is vital to use an automated speech analysis system in order to help users get entirely new opportunities to study and control the situation, take operational management decisions and further plan related activities in the public sphere and business workspace. Research and development of automated systems for unstructured analysis of digitalized audio data which does not contain text transcript or key word indication is a prospective area of science.
The main application of such systems is ensuring security (national, business or personal) and improving the quality of service (public, contractors and customers), which allows including them into such priority areas of science, technology and engineering of Russian Federation as \"Security and Terrorism Prevention\" and \"Information and communication technologies.\" It is notable that by providing the improved security and quality of service the system entails significant cost reduction thanks to a more rapid response to critical situations, and continuous effectiveness improvement of interaction with partners of modern organizations.
Pages: 25-36
References
- http://newzealand.nuance.com/news/20060801_dns.asp.
- http://australia.nuance.com/news/20070426_recognizer.asp.
- http://speech-drive.ru/recognize/.
- www.loquendo.com.
- Ney H. et al. The RWTH Large vocabulary continuous speech recognition system // In IEEE ICASSP. USA. 1998. P. 853-856.
- Loof J. et al. The RWTH 2007 TC-STAR Evaluation system for european english and spanish // In Proc. of Interspeech 2007. P. 2145-2148. Belgium. 2007.
- Greenberg S., Chang S. Linguistic dissection of switchboard-corpus automatic speech recognition systems. ISCA Workshop on Automatic Speech Recognition: Challenges for the New Millennium. Paris, 2000.
- Martin A., Pryzbocki M., Fiscus J., Pallet D. The 2000 NIST evaluation for recognition of conversational speech over the telephone. Presentation at the NIST Speech Transcription Workshop. 2000.
- Evermann G. et al. Development of the 2003 CU-HTK conversational telephone speech transcription system // In Proc. ICASSP. 2004.
- Furui S. Selected topics from 40 years of research on speech and speaker recognition // In Proc. of Interspeech 2009. P. 1-8.
- Akita Y., Mimura M., Kawahara T.Automatic transcription system for meetings of the japanese national congress. In Proc. of Interspeech 2009. P. 84-87.
- Nouza J., Cerva P., Zdansky J.Very large vocabulary voice dictation for mobile devices // In Proc. of Interspeech 2009. P. 995-998.
- Marasek K. Polish LVCSR in the Janus system. Preliminary results for the SpeeCon database // Archives of Acoustics. 2007.V. 32.№ 1.P. 119 - 126.
- Loof J. Gollan Ch., Ney H. Cross-language bootstrapping for unsupervised acoustic model training: rapid development of a polish speech recognition system // In Proc. of Interspeech 2009. P. 88-91.
- http://newsdesk.pcmag.ru/node/5975.
- Yamada M., et al. Improvement of rejection performance of keyword spotting using anti-keywords derived from large vocabulary considering acoustical similarity to keywords // In Proc. of Interspeech 2005.
- Heracleous P., Shimizu T. An efficient keyword spotting technique using a complementary language for filler models training. 8th european conference on speech communication and technology (Eurospeech 2003). 2003. P. 921-924.
- Smidl L., Muller L. Keyword Spotting for Highly Inflectional Languages // In Proc. of the 8th. ICSLP. USA. 1996. P. 2067-2070.
- Lin Q., Das S., Lubensky D., Picheny M. A New Confidence measure based on rank-ordering subphone scores // In Proc. ICSLP \'98.Australia. 1998.
- Manos A., Zue V. A segment based wordspotter using phonetic filler models 1997 // In Proc. of the 1997 IEEE ICASSP. 1997.V. 2. P. 899.
- Szoke I. et al. Comparison of keyword spotting approaches for informal continuous speech // In Proc. Of INTERSPEECH 2005. P. 633-636.
- www.speechpro.ru.
- www.nexidia.com.
- Hazen T., Richardson F., Margolis A.Topic identification from audio recordings using word and phone recognition lattices // In Proc. ASRU, Kyoto, December 2007.
- Hazen T., Margolis A. Discriminative feature weighting using mce training for topic identification of spoken audio recordings // In Proc. ICASS. USA, 2008.
- Gish H. et al. Unsupervised training of an HMM-based speech recognizer for topic classification // In Proc. of Interspeech 2009. P. 1935-1938.
- Smirnov V.A. Ermilov S.N. Slovo ne vorobejj... Rechevaja analitika dlja sluzhby bezopasnosti // Direktor po bezopasnosti. Nojabr 2010. № 11. C. 28-37.
- Smirnov V.A., Gusev M.N., Farkhadov M.P. Funkcija lingvisticheskogo processora v sisteme avtomaticheskogo analiza nestrukturirovannojj rechevojj informacii // Avtomatizacija i sovremennye tekhnologii. 2013. № 8. S. 22-28.
- Smirnov V. A., Gusev M. N., Farkhadov M. P. Funkcija modulja akusticheskogo modelirovanija v sisteme avtomaticheskogo analiza nestrukturirovannojj rechevojj informacii // Upravlenie bolshimi sistemami. M.: IPU RAN. 2013. Vyp. 45. S.181-205.
- www.speech-drive.ru
- Bilik P.B., ZHozhikashviljj V.A., Petukhova N.V., Farkhadov M.P. Analiz rechevogo interfejjsa v interaktivnykh servisnykh sistemakh // I. Avtomatika i telemekhanika. 2009. № 2. S. 80-89.
- ZHozhikashvili V.A., Petukhova N.V., Farkhadov M.P. Kompjuternye sistemy massovogo obsluzhivanija i rechevye tekhnologii // Problemy upravlenija. 2006. № 2. S. 3-7.
- ZHozhikashvili V.A., Bilik R.V., Vertlib V.A., ZHozhikashvili A.V., Petukhova N.V., Farkhadov M.P. Otkrytye sistemy massovogo obsluzhivanija s raspoznavaniem rechi // Problemy upravlenija. 2003. № 4. S. 55-62.
- Petukhova N.V., Vaskovskijj S.V., Farkhadov M.P., Smirnov V.A. Arkhitektura i kharakteristiki sistem raspoznavanija rechi // Nejjrokompjutery: razrabotka, primenenie. 2013. № 12. S. 22-30.
- Farkhadov M.P.Raspoznavanie rechi v sistemakh massovogo obsluzhivanija naselenija // Trudy SPIIRAN. 2011. Vyp. 4 (19). S. 65-86.
- Chechkin A.V., Pirogov M.V. Radical programming technology based on radical modeling // Nejjrokompjutery. Razrabotka, primenenie. 2016. № 1. S. 3-16.
- Pavlovskijj I. S.Smyslovaja integracija nauchno-tekhnicheskojj informacii v oblasti razrabotki i primenenija nejjrosetevykh tekhnologijj // Nejjrokompjutery. Razrabotka, primenenie. 2016. № 3. S. 47-53.