350 rub
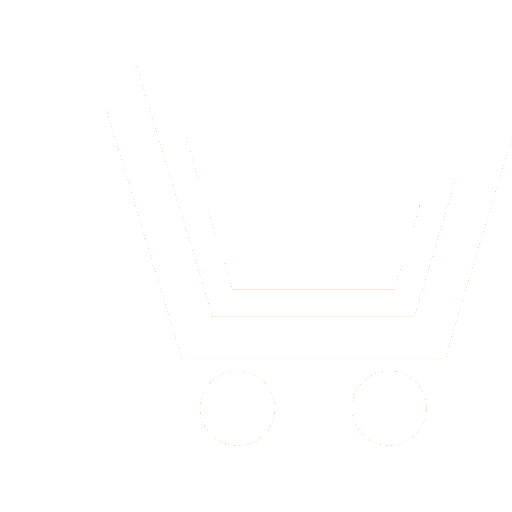
Journal Neurocomputers №3 for 2016 г.
Article in number:
Comparative study of iterative and direct neural network short term electricity load forecasting of a city
Keywords:
artifical neural network
multilayer perceptron
short term electricity load forecasting
metaparameters
iterative and direct forecasting
Authors:
I.E. Shepelev - Ph.D. (Eng.), Senior Research Scientist, A.B. Kogan Research Institute for Neurocybernetics, Academy of biology and biotechnology, Southern Federal University (Rostov-on-Don). E-mail: shepelev@krinc.ru
I.I. Nadtoka - Dr.Sc. (Eng.), Professor, Head of the Department, Platov South-Russian State Polytechnic University (NPI) (Novocherkassk). E-mail: ii_nadtoka@mail.ru
S.A. Vialkova - Engineer, Platov South-Russian State Polytechnic University (NPI) (Novocherkassk). E-mail: mazaeva_sveta@mail.ru
S.O. Gubski - Ph.D. (Eng.), Platov South-Russian State Polytechnic University (NPI) (Novocherkassk). E-mail: hromo@inbox.ru
Abstract:
In the paper a comparison between multi-step iterative and one-step direct neural network short term electricity load forecasting for data from Moscow-city is conducted. An identification of optimal metaparameters for neural network model is considered. The metaparameters are neural network input lag length, size of neural network hidden layer, depth of training set, radius of training neighbourhood, a set of significant inputs and neural network regularization coefficient. Forecast designing is based on multilayer perceptron. It has been shown that one-step direct forecasting is more accurate then multi-step iterative one for our case.
Pages: 21-30
References
- Feinberg E. A., Genethliou D. Load forecasting. // In Applied Mathematics for Restructured Electric Power Systems. J. H. Chow, F. F.Wu, & J. A. Momoh (Eds.). Springer. 2005. P.269−285.
- Dordonnat V.,Koopman S., Ooms M., Dessertaine A.., Collet J.An hourly periodic state space model for modelling French national electricity load // International Journal of Forecasting. 2008. V.24. № 4. P.566-587.
- CanceloJ., Espasa A., Grafe R. Forecasting the electricity load from one day to one week ahead for the Spanish system operator // International Journal of Forecasting. 2008. V.24. № 4. P.588-602.
- Soares L., Medeiros M. Modeling and forecasting short-term electricity load. A comparison of methods with an application to Brazilian data // International Journal of Forecasting. 2008. V.24. № 4. P.630-644.
- Hippert H.S., Pedreira A.C., Souza R.C. Neural networks for short-term load forecasting // IEEE Transactions on Power Systems. 2001. V.16. P.44−55.
- Tashman L. Out-of-Sample Tests of Forecasting Accuracy: An Analysis and Review // International Journal of Forecasting. 2000. V. 16. № 4. P. 437-450.
- Gill F., Mjurrejj U., Rajjt M. Prakticheskaja optimizacija: Per. s angl. M.: Mir.1985. 509 s.
- KHajjkin S. Nejjronnye seti: polnyjj kurs. Izd. 2-e. M.: Viljams. 2006. 1104 c.
- Hong T. Energy Forecasting: Past, Present and Future // Foresight: The International Journal of Applied Forecasting. 2014. V.32. P.43-48.
- Hippert H.S., Bunn D.W., Souza R.C. Large neural networks for electricity load forecasting: Are they overfitted - // International Journal of Forecasting. 2005. V. 21. № 3. P. 425-434.
- Osovskijj S.Nejjronnye seti dlja obrabotki informacii: Per. I. D. Rudinskogo. M.: Finansy i statistika.2004. 344 s.