350 rub
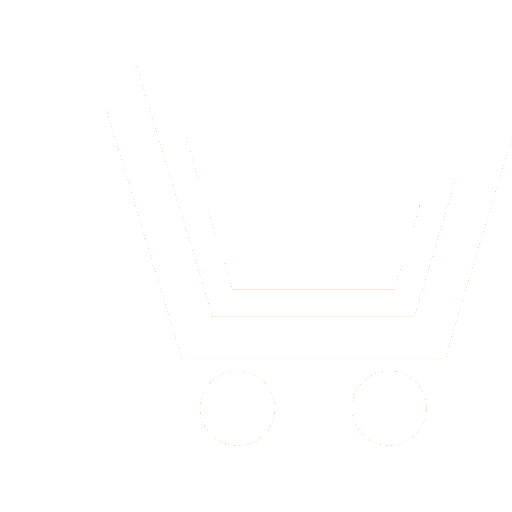
Journal Neurocomputers №10 for 2016 г.
Article in number:
The application of the multi-alternative approach in intelligent systems: active neural network models
Keywords:
intelligent systems
neural networks active
multi-alternative approach
facet memory organization
Authors:
S.L. Podvalny - Dr.Sc. (Eng.), Professor, Head of Department of Automated and Computer Systems, Voronezh State Technical University
E-mail: spodvalny@yandex.ru
E.M. Vasiljev - Ph.D. (Eng.), Associate Professor, Department of Automation and Informatics in Technical University, Voronezh State Technical University
E-mail: vgtu-aits@yandex.ru
Abstract:
The article deals with the construction of intelligent systems based on artificial neural networks. There have formal grounds the use of neural networks. We discuss the properties of non-compliance of artificial neural networks and their biological counterparts. Artificial neural networks have the property retrained, and storing in natural neural networks is cumulative and selective. Artificial neural networks are characterized by low generalizing properties, but the biological neural networks have high recognition ability \"private-general\". The artificial network is the problem dimension parameters, in natural prototypes unlimited memory. The main cause of these discrepancies - structural immutability neural network models in the learning process, that is, their passivity. These shortcomings lead to undeserved discredit of artificial neural networks. It is proposed to proceed to the construction of the active, reconfigurable neural networks. Construction of active neural networks is possible on the basis of biological principles multi-alternative: multilevel, diversity, modularity. Multilevel principle is realized in living organisms in several hierarchical levels of control. The principle of diversity is the reconfiguration of the structures and the algorithms. The principle of modularity is the discrete nature of the system structure, with elements of the system can re-combine. A particular method of implementation of these principles, which uses faceted organization in memory and reconfi-gurable neural network, active structure. Faceted memory organization allows to combine network and hierarchical organization of data in the neural network. In every facet of memory external event corresponds to one ensemble of neurons. At the same time memorizing a new event by simply adding a new ensemble. The new ensemble of neurons embedded in the existing structure of the neural network, and existing ensembles are not changed. As a result, the organization facet of memory allows you to build active neural networks with reconfigurable structure. An example of the implementation of the neural network of the active facet of intellectual type electrical distribution system control system. In the event of a critical situation in the system (short circuit or open circuit) are excited by the respective assemblies of neurons. In the aggregate excited ensembles formed by the output of the neural network. This output signal switches off the damaged area, or includes the necessary back-up line. Thus, the use of multi-alternative principles allows you to build a neural network with reconfigurable structure, eliminates the difficulty of retraining, and provides high generalizing ability of artificial neural networks.
Pages: 49-58
References
- Viner N. Kibernetika ili upravlenie i svjaz v zhivotnom i mashine. M.: Nauka. 1983. 344 s.
- EHshbi U.R. Vvedenie v kibernetiku. M.: URSS. 2006. 432 s.
- Anokhin P.K. Sistemnye mekhanizmy vysshejj nervnojj dejatelnosti. M.: Nauka. 1979. 453 s.
- Karpenkov S.KH. Koncepcii sovremennogo estestvoznanija. M.: Akademicheskijj Proekt. 2003. 640 s.
- McCulloch W.A., Pitts W. A logical calculus of the ideas immanent in nervous activity // The Bulletin of Mathematical Biophysics. 1943. № 5. P. 115-133.
- Mak-Kallok U.S., Pitts U. Logicheskoe ischislenie idejj, otnosjashhikhsja k nervnojj aktivnosti // Nejjronnye seti: istorija razvitija teorii. M.: Radiotekhnika. 2001. S. 5-22.
- Redko V.G. EHvoljucija, nejjronnye seti, intellekt: modeli i koncepcii ehvoljucionnojj kibernetiki. M.: Librokom. 2013. 224 s.
- SHamis A.L. Puti modelirovanija myshlenija. M.: Komkniga. 2006. 336 s.
- Podvalnyjj S.L. EHvoljucionnye principy formirovanija struktury vychislitelnykh sistem // Adaptacija v slozhnykh sistemakh upravlenija: sb. nauch. tr. Voronezh: Voronezhskijj politekhnicheskijj institut. 1979. S. 60-63.
- Podvalnyjj S.L. Mnogoalternativnye sistemy: obzor i klassifikacija // Sistemy upravlenija i informacionnye tekhnologii. 2012. T. 48. № 2. S. 4-13.
- Podvalnyjj S.L., Vasilev E.M. Mnogoalternativnoe upravlenie otkrytymi sistemami: koncepcija, sostojanie i perspektivy // Upravlenie bolshimi sistemami. 2014. № 48. S. 6-58.
- Podvalny S.L., Vasiljev E.M. Evolutionary principles for construction of intellectual systems of multi-alternative control // Automation and Remote Control. 2015. V. 76. № 2. P. 311-317.
- Dli M.I., Kruglov V.V. Nechetkaja logika i iskusstvennye nejjronnye seti. M.: Fizmatlit. 2001. 224 s.
- Kruglov V.V. Iskusstvennye nejjronnye seti. Teorija i praktika. M.: Gorjachaja linija-Telekom. 2001. 382 s.
- KHajjkin S. Nejjronnye seti: polnyjj kurs. M.: Viljams. 2006. 1104 s.
- CHernodub A.N., Dzjuba D.A. Obzor metodov nejjroupravlenija // Problemy programmirovanija. 2011. № 2. S. 79-94.
- Vasilev E.M., Merenkov V.V. Metaehvristicheskijj algoritm obuchenija nejjronnykh setejj // EHlektrotekhnicheskie kompleksy i sistemy upravlenija. 2009. № 3. S. 56-59.
- Tkalich S.A., Vasilev E.M. Osnovanija i vozmozhnosti ispolzovanija iskusstvennykh nejjrosetejj v sistemakh prognozirovanija // EHlektrotekhnicheskie kompleksy i sistemy upravlenija. 2008. №2. S. 37-38.
- Manukovskaja M.M., Vasilev E.M. Aktivnye nejjrosetevye modeli prinjatija reshenijj // EHlektrotekhnicheskie kompleksy i sistemy upravlenija. 2008. № 4. S. 76-78.
- Jandel M. Biologically relevant neural network architectures for support vector machines // Neural Networks. 2014. № 49. P. 39-50.
- Schittenkopf C., Deco G., Brauer W. Two Strategies to Avoid Overfitting in Feedforward Networks // Neural Networks. 1997. V. 10. № 3. P. 505-516.
- Weng J., Luwang T., Lu H., Xue X. Multilayer in-place learning networks for modeling functional layers in the laminar cortex // Neural Networks . 2008. № 21. P. 150-159.
- Hastie T., Tibshirani R., Friedman J. The Elements of Statistical Learning: Data Mining, Inference, and Prediction. Springer-Verlag. 2009. 746 p.
- Podvalnyjj S.L., Vasilev E.M. Modeli mnogoalternativnogo upravlenija i prinjatija reshenijj v slozhnykh sistemakh // Sistemy upravlenija i informacionnye tekhnologii. 2014. T. 56. № 2.1. S. 169-173.
- Podvalnyjj S.L., Vasilev E.M. Intellektualnye sistemy mnogoalternativnogo upravlenija: principy postroenija i puti realizacii // XII Vserossijjskoe soveshhanie po problemam upravlenija VSPU-2014. Institut problem upravlenija im. V.A. Trapeznikova RAN: trudy. (Moskva, 16-19 ijunja 2014 g.). M.: IPU RAN. 2014. S. 996-1007.
- Myshev A.V. Informacionnaja model nejjroseti v tekhnologijakh vychislitelnogo intellekta i formakh realizacii kompjutinga // Nejjrokompjutery: razrabotka, primenenie. 2011. № 10. S. 3-12.
- Myshev A.V. Rekonfiguriruemye virtualnye potokovye vychislitelnye sistemy na osnove informacionnojj modeli nejjroseti // XII Vserossijjskoe soveshhanie po problemam upravlenija VSPU-2014. Institut problem upravlenija im. V.A. Trapeznikova RAN: trudy. (Moskva, 16-19 ijunja 2014 g.). M.: IPU RAN. 2014. S. 7307-7318.
- Mugatina V.M., Vasilev E.M. Nejjro-informacionnye struktury v sistemakh prinjatija reshenijj // Sovremennye metody prikladnojj matematiki, teorii upravlenija i kompjuternykh tekhnologijj: sb. nauch. tr. VII Mezhdunar. konf. PMTUKT-2014. Voronezh: Nauchnaja kniga, 2014. S. 265-268.
- Vasilev E.M., Govorov R.A. Aktivnaja nejjrosetevaja model upravlenija kriticheskimi obektami // Vestnik Voronezhskogo gosudarstvennogo tekhnicheskogo universiteta. 2015. T. 11. № 3. S. 31-36.