350 rub
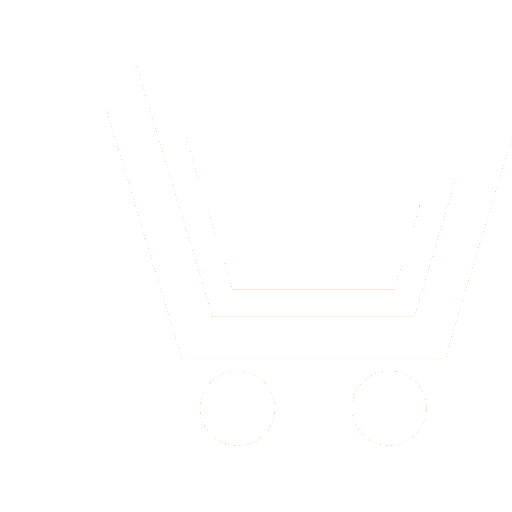
Journal Neurocomputers №7 for 2015 г.
Article in number:
Investigation of the effect of the choice of activation functions of the performance of the multi-layer perceptron
Authors:
W.A. Al-Haidri - Post-graduate Student, Department of Biomedical and Electronic Systems and Technology, Vladimir State University. E-mail: fawaz_tariq@mail.ru
R.V. Isakov - Ph.D.(Eng.), Associate Professor, Department of Biomedical and Electronic Systems and Technology, Vladimir State University. E-mail: Isakov-RV@mail.ru
L.T. Sushkova - Dr.Sc.(Eng.), Professor, The Head of Biomedical and Electronic Systems and Technology Department, Vladimir State University. E-mail: ludm@vlsu.ru
Abstract:
Artificial neural networks (ANN) are a set of models of biological neural networks connected by a synaptic connection. The network processes the input information and the process of changing its state by time generates a set the output signal [1].
There are various models of neural networks (ANN), the most famous of which is the multilayer perceptron (MLP), which has great potential for solving various problems. Thanks to its stable capacity and simple design, it has been widely used in various applications. However, in some cases, the MP can not provide a good solution, which may be due to the wrong choice of architecture, the initialization of weights or selecting data. Another factor that affects the learning process is the choice of transfer function (activation function) [3]. The purpose of this paper is a comparative analysis of the impact of the most common and frequently used activation functions on the result of MP used in electrocardiographic (ECG) artifacts detection.
Pages: 60-66
References
- Zaencev I. V. Nejjronnye seti: osnovnye modeli. Uchebnoe posobie k kursu «Nejjronnye seti». Voronezh: VoronezhskijjGosudarstvennyjjuniversitet. 1999.
- Cos¸kun Özkan and Filiz Sunar Erbek. The Comparison of Activation Functions for Multispectral Landsat TM Image Classification // Photogrammetric Engineering & Remote Sensing. November 2003. V. 69. № 11. P. 1225-1234.
- Piekniewski F.,Tybicki L. Visual comparison of performance for different activation functions in MLP networks // Proceedings of International Joint Conference on Neural Networks: IJCNN - 04. 2004. V. 4. P. 2947-2952.
- Duch W.,Jankovski N. Survey of neural transfer functions, Neural Computing Surveys 1999. V. 2. P. 163-212.
- JAsnickijj L.N. Iskusstvennyjj intellekt: populjarnoe vvedenie dlja uchitelejj i shkolnikov // http://inf.1september.ru/view_article.php-ID=200902304.
- Al-KHulejjdi N.A.,Isakov R.V., Sushkova L.T. Rezultaty issledovanija nejjronnykh setejj v zadachakh raspoznavanija variabelnosti serdechnogo ritma. // Nejjrokompjutery: razrabotka, primenenie. 2012. № 6. S. 61-67.
- Pavlova O.N., Pavlov A.N. Registracija i predvaritelnaja obrabotka signalov s pomoshhju izmeritelnogo kompleksa MR 100: Ucheb. posobie. Saratov: Nauchnaja kniga. 2008.
- Rangajjjan R.M. Analiz biomedicinskikh signalov. Prakticheskijj podkhod: Per. s angl. pod red. A.P. Nemirko. M.: Fizmatlit. 2007. 440 s.
- Al-KHajjdri V.A., Isakov R.V., Sushkova L.T. Obzor osnovnykh metodov obnaruzhenija artefaktov v biomedicinskikh signalakh // Materialy KH Mezhdunar. nauch. konf. «Fizika i radioehlektronika v medicine i ehkologii». 2014. S. 379-382.
- Salekh M.A., Isakov R.V., Sushkova L.T. Segmentarnyjj podkhod k obrabotke ehlektrokardiograficheskikh pri postroenii sistem avtomatizirovannogo analiz // Proektirovanie i tekhnologija ehlektronnykh sredstv. 2010. № 4. S. 24-29.