350 rub
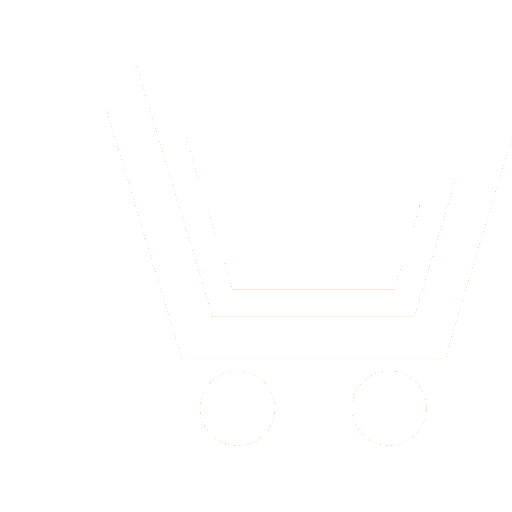
Journal Neurocomputers №6 for 2015 г.
Article in number:
Hybrid multi-agent classifiers in bioengineering systems of diagnosis of the disease and onitoring prescribing
Keywords:
bioactive points
current-voltage characteristics of biomaterials
multi-agent system of classification
informative signs
probabilistic neural networks
indistinct neural networks
hybrid structures
Authors:
М.А. Efremov - Ph. D. (Eng.), Associate Professor, Chair of Information Security and Communication Sys-tems, South-West State University (Kursk). E-mail: efremov_ma@mail.ru
О.V. Shatalova - Ph. D. (Eng.), Associate Professor, Chair of Biomedical Engineering, South-West State University (Kursk). E-mail: shatolg@mail.ru
V.V. Fedyanin - Post-graduate Student, Chair of Biomedical Engineering, South-West State University (Kursk). E-mail: fedyanin.50@mail.ru
А.N. Shutkin - Ph. D. (Phis.-Math.), Deputy Head of the Institute for Studies, Voronezh Institute of the State Fire Service (Voronezh). E-mail: anshutkin@mail.ru
Abstract:
Multiagent structure are usuing for implement classifiers intended for diagnosis and monitoring of adverse reactions prescribing. Each agent of structure works with its feature space, which is formed on bioimpedance researches of \"their\" bioactive point (BAP). BAP are selecting on time and/or on the revolting influence. Agents of lower layer were built according to the probabilistic scheme of the organization of neural networks (PNN).
The task of the meta-classifier includes aggregation solutions, which are taken by agents of lower hierarchy level and integration of lessons learned diagnostic pathology information, removed from BAP. This BAP were included in multi-agent system of classification - a hybrid structure of PNN-FNN. It includes a set of independent modules classification, which analyze the power characteristics of \"their\" BAP. They give conclude only by one special for each agent, disease. The combined solution, taken a single agent - classifier, is accepted in a meta-classifier - FNN.
Layer is added to probabilistic layer of neural network for final decision. Added layer is made by fuzzy technology - FNN, which is based on fuzzy logic decision-making.
Aggregation of decisions on the M groups of signs is carried out in a layer FNN, which has structural and functional organization of fuzzy neural network and comprises three layers: defuzzifikator layer, a layer of aggregators and diffuzifikator. The only difference is the absence of a defuzzifikator at the exit.
Results of other researches such as anamnesis, general and biochemical blood test, ultrasonography of kidneys and other are taken into account to obtain reliable conclusions on the differential diagnosis.
L network structures PNN-FNN, which have 2L output, is necessary for differentiation of the L pathologies.
One more macrolayer FNN* layer is used to aggregation 2L outputs. Each module macrolayers FNN*consist of 2 layers. First layer has 2 neurons, in first of neurons is carried subjective of probability calculus of the presence of a specific disease or ad-verse reaction, in second one is carried subjective probability of it absence.
The second layer classification module macrolayers FNN *has only one neuron, which which performs a role similar to the role defuzzifikator in the fuzzy inference.
Pages: 42-47
References
- Luvsan G.Tradicionnye i sovremennye aspekty vostochnojj refleksoterapi Izd. 3-e., pererab. i dop. Novosibirsk: Nauka. 1991. 432 s.
- Filist S.A., Surzhikova S.E., Kassim KabusD.A. Metod issledovanija ehlektricheskikh kharakteristik bioaktivnykh tochek // Nauchnyjj vzgljad na sovremennyjj ehtap razvitija obshhestvennykh, tekhnicheskikh, gumanitarnykh i estestvennykh nauk. Aktualnye problemy: Sb. statejj po itogam Vseros. nauch.-prakt. konf. sankt-Peterburg. 2014. S. 118-122.
- Akulina M.M., Rybchenko A.A., Solomonov V.T. Ispolzovanie tochek akupunktury v avtomatizirovannojj sisteme profilakticheskikh osmotrov // Teorija i praktika refleksoterapii, mediko-biologicheskie i fiziko-tekhnicheskie aspekty. 1981. S. 216-223.
- Arsenev V.E., Berdashkevich A.P., Glazunov A.S. Issledovanie vozmozhnosti diagnostiki zabolevanijj cheloveka po volt-ampernym kharakteristikam vydelennykh uchastkov kozhi // Teorija i praktika refleksoterapii, mediko-biologicheskie i fiziko-tekhnicheskie aspekty. 1981. S. 232-236.
- Filist S.A., Emeljanov S.g., Rybochkin A.F. Nejjrosetevojj reshajushhijj modul dlja issledovanija zhivykh sistem // Izv. Kurskogo gosudarstvennogo tekhnicheskogo universiteta. 2008. № 2 (23), 2008. S. 77-82.
- FilistS.A. Korenevskijj N.A., shatalova O.v. i dr. diagnosticheskaja sistema na osnove analiza voltampernykh kharakteristik bioaktivnykh tochek// Biotekhnosfera. 2013. № 5 (29). S. 33-38.
- Rutkovskaja D., Pilinskijj M., Rutkovskijj L. Nejjronnye seti, geneticheskie algoritmy i nechetkie sistemy: Per. s polsk. I.D. Rudinskogo. M.: Gorjachaja linija «Telekom». 2004. 452 s.
- FilistS.A., shatalova O. v., efremov M. A. Gibridnaja nejjronnaja set s makroslojami dlja medicinskikh prilozhenijj// Nejjrokompjutery: Razrabotka, primenenie. 2014. № 6. S. 35-39.
- Terzijan A. V., Vitko V. JA. Verojatnostnye metaseti dlja reshenija zadach intellektualnogo analiza dannykh // Iskusstvennyjj intellekt. 2002. № 3. S. 188-197.