350 rub
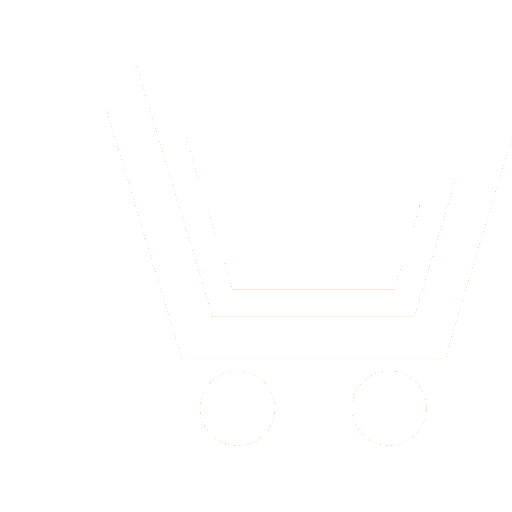
Journal Neurocomputers №2 for 2015 г.
Article in number:
The research of neural network identification of aircraft planes by impulse characteristics
Keywords:
impulse characteristic (IC)
artificial neural network
frequency tuning signals
objects identification
Authors:
Mitrofanov D.G. - Dr. Sc. (Eng.), Professor, Vasilevsky Military Academy of Air Defense Forces RF (Smolensk). E-mail: mdgvapvo@yandex.ru
Safonov A.V. - Ph. D. (Eng.), Associate Professor, Head of Department, Tyumen Higher Military Engineering Com-mand School named after marshal A.I. Proshlyakov. E-mail: sulaco33@yandex.ru
Bortovik V.V. - Ph. D. (Eng.), Associate Professor, Vasilevsky Military Academy of Air Defense Forces RF (Smolensk)
E-mail: bvv30@yandex.ru
Kichulkin D.A. - Main Engineer, Smolensk Scientific Innovative Centre of Radioelectronic Systems «ZAVANT». E-mail: info@zavant.ru
Krasavtsev O.O. - Senior Engineer, Smolensk Scientific Innovative Centre of Radioelectronic Systems «ZAVANT». E-mail: info@zavant.ru
Abstract:
The researches actuality of informative features of air objects identification is mentioned. The hypothesis about using possibility for Impulse Characteristic identification as a total of impulse response from individual scatterers on the surface of aircraft plane is formu-lated. The most significant factors, decreasing impulse characteristic informativeness as feature identification, are indicated. The di-lemma of the most perspective apparatus of making decision on impulse characteristic vectors, as an individual task, is marked. The neural network technologies, which advantages are stated, are suggested to be used as such apparatus. The conditions and parameters of mathematical experimentations with models of air objects and also a recommended approach to the formation of object impulse characteristics by modeling method are described. This approach supposes the radiation of objects by signals with carrier frequency tuning from impulse to impulse, the reception and receiving a multifrequency reflective characteristic and carrying out discrete Fourier transformation with it. The results of comparison of estimation experimental three-alternative object classification by different neural networks are represented and as a result of which the probabilistic network is the best. The decision of five-alternative neural network task identification within three classes of aircraft planes models, differed by geometrical dimension, is described. The received estimate of identification is represented in the form of graphical characteristics, depending on the level of noise change in the accepting realization. The analysis results of neural network identification of objects models is made with the help of probabilistic neural network. The conclusion on conducted mathematical experiments results is formulated.
Pages: 57-63
References
- Radiolokacionnye sistemy. Spravochnik. Osnovy postroenija i teorija / Pod red. JA.D. SHirmana. M.: Radiotekhnika. 2007. 510 s.
- Mitrofanov D.G. Sposob postroenija dvumernogo radiolokacionnogo izobrazhenija vozdushnojj celi s proizvolnojj nestabilnojj traektoriejj poleta // Izmeritelnaja tekhnika. 2002. № 8. S. 7−11.
- Mitrofanov D.G. Kompleksnyjj adaptivnyjj metod postroenija radiolokacionnykh izobrazhenijj v sistemakh upravlenija dvojjnogo naznachenija // Izvestija RAN. Teorija i sistemy upravlenija. 2006. № 1. S. 101−118.
- Gorelik A.L., Barabash JU.L., Krivosheev O.V., EHpshtejjn S.S. Selekcija i raspoznavanie na osnove lokacionnojj informacii. M.: Radio i svjaz. 1990. 270 s.
- http://kbradar.by/text/pages-view-15.html.
- http://www.pandia.ru/text/77/451/4990-32.php.
- Bashkirov L.G., Sablin V.N., CHapurskijj V.V., SHejjko A.P. Radiolokacionnoe raspoznavanie vozdushnykh obektov po radioakusticheskim spektralnym portretam s ispolzovaniem obuchaemykh nejjronnykh setejj // Polet. 2002. № 8. S. 23−33.
- Sosulin JU.G., Fam CHung Zung. Nejjrosetevoe raspoznavanie dvumernykh izobrazhenijj // Radiotekhnika i ehlektronika. 2003. T. 48. S. 969−978.
- CHapurskijj V.I. Raspoznavanie vozdushnykh celejj po strukture tenevykh spektralnykh portretov pri ispolzovanii nejjronnykh setejj // Radioehlektronika. 2003. T. 33. № 1. S. 1−25.
- Safonov A.V. Razrabotka metodiki obuchenija nejjrosetevogo klassifikatora letatelnykh apparatov po ikh radiolokacionnym izobrazhenijam // Voprosy radioehlektroniki (M.: OAO CNII «EHlektronika»). 2008. T. 2. № 3. 195 s.
- Farkhat N.KH. Formirovanie radiolokacionnykh izobrazhenijj metodom raznesenija v diapazone SVCH i avtomatizirovannaja identifikacija celejj, osnovannaja na ispolzovanii modelejj nejjronnykh setejj // TIIEHR. 1989. T. 77. № 5. S. 43−56.
- Galushkin A.I. Nejjrokompjutery: razrabotka i primenenie. T. 1. M.: IPRZHR. 2000.
- Golovko V.A. Nejjronnye seti: obuchenie, organizacija i primenenie / Pod red. A.I. Galushkina. M.: IPRZHR. 2001. 256 s.
- Kruglov V.V., Borisov V.V. Iskusstvennye nejjronnye seti: teorija i praktika. M.: Gorjachaja linija-Telekom. 2001. 384 s.
- Krispin ml., Maffett. Ocenka radiolokacionnogo poperechnogo sechenija tel prostojj formy // TIIEHR. 1965. T. 53. № 8. S. 960−975.
- Stiven Smit. Cifrovaja obrabotka signalov: prakticheskoe rukovodstvo dlja inzhenerov i nauchnykh rabotnikov / Per. s angl. M.: Dodehka-XXI. 2008. 720 s.
- Medvedev V.S., Potemkin V.G. Nejjronnye seti: Matlab 6. M.: Dialog-MIFI. 2002. 496 s.