350 rub
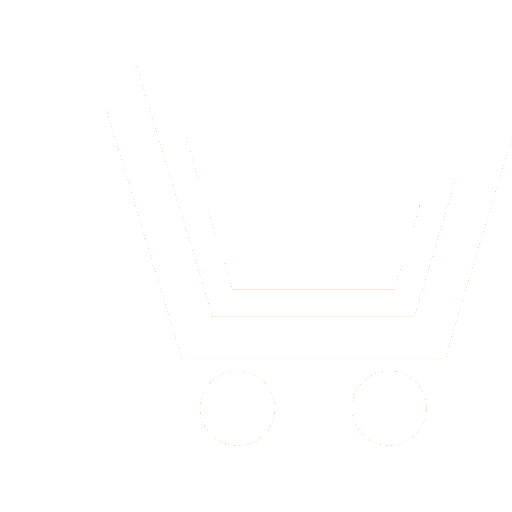
Journal Neurocomputers №2 for 2014 г.
Article in number:
Specificities of computer simulation of spiking recurrent neural networks
Keywords:
simulation software
recurrent neural network
spiking neurons
reservoir computing
dynamic synapses
bio¬inspired models
Authors:
E.N. Benderskaya - Ph.D. (Eng.), St.-Petersburg State Polytechnical University. E-mail: helen.bend@gmail.com
K.V. Nikitin - Post-graduate Student, St.-Petersburg State Polytechnical University. E-mail: execiter@mail.ru
K.V. Nikitin - Post-graduate Student, St.-Petersburg State Polytechnical University. E-mail: execiter@mail.ru
Abstract:
In this paper a classification of spike recurrent neural networks (RNN), analyzed the features of the different classes of models, learning algorithms and their respective RNN computational capabilities. The results of the analysis of the spike in the RNN tank calculations. Switching neurons are considered and the momentum representation of information, the classification of a spike coding information. A comparison of the simulation environment spike RNN. Is a special algorithm simulation of spike RNN, through which can be modeled by all major models of spike neurons, electrical and chemical synapses, synapses with plasticity and dynamic synapses. The advantage of the algorithm is that the addition of new models of neurons, synapses, requires no changes to the algorithm itself.
Pages: 54-65
References
- Benderskaya E.N., Nikitin K.V. Analiz modeli impul'snoj rekurrentnoj nejronnoj seti v sostave mashiny' neustojchivy'x sostoyanij // Nejrokomp'yutery': razrabotka, primenenie. 2013. № 12.
- Benderskaya E.N., Zhukova S.V. Osczillyatorny'e nejronny'e seti s xaoticheskoj dinamikoj v zadachax klasternogo analiza // Nejrokomp'yutery': razrabotka, primenenie. 2011. № 7. S. 74-86.
- Benderskaya E.N., Nikitin K.V. Rekurrentnaya nejronnaya set' kak dinamicheskaya sistema i podxody' k ee obucheniyu // Nauchno-texnicheskie vedomosti SPbGPU. 2013. № 4(176). S. 29-40.
- Nikolls Dzh.G., Martin A.R., Vallas B.Dzh., Fuks P.A. Ot nejrona k mozgu. M.: LKI. 2008. 672 s.
- Abarbanel' G.D. i dr. Sinxronizacziya v nejronny'x ansamblyax // Uspexi fizicheskix nauk. 1996. T. 166. № 4. S. 363-390.
- Xajkin S. Nejronny'e seti. M.: Vil'yams. 2000. 1104 s.
- Cukerman V.D. Matematicheskaya model' fazovogo kodirovaniya soby'tij v mozge // Matematicheskaya biologiya i bioinformatika. 2006. T. 1. № 1. S. 97-107.
- Buonomano D.V., Maass W. State-dependent computations Spatiotemporal processing in cortical networks // Neuroscience. 2009. V. 10. P. 113-125.
- Buonomano D.V., Merzenich M.M. Temporal information transformed into a spatial code by a neural network with realistic properties // Science. 1995. V. 267. № 5200. P. 1028-1030.
- Gerstner W., Kistler W.M. Spiking Neuron Models. Single Neurons, Populations, Plasticity. Cambridge: University Press. 2002. 480 p.
- Hines M.L., Carnevale N.T. Discrete event simulation in the NEURON environment // Neurocomputing. 2004. V. 58. P. 1117-1122.
- Izikevich E.M. Dynamical systems in neuroscience: the geometry of excitability and bursting. Cambridge: The MIT Press. 2007. 505 p.
- Maass W., Natschläger T., Markram H. Real-time computing without stable states: a new framework for neural computations based on perturbations // Neural Computation. 2002. V. 11. P. 2531-2560.
- Natschläger T., Ruf B. Spatial and temporal pattern analysis via spiking neurons // Network. 1998. V. 9. № 3. P. 319-332.
- Schrauwen B., VerstraetenD., Campenhout J.V. An overview of reservoir computing theory, applications and implementations // Proc. of the 15th European Symposium on Artificial Neural Networks. 2007. P. 471-482.
- Siegelmann H.T. Neural and super-Turing computing // Minds and Machines. 2003. V. 13. P. 103-114.
- Siegelmann H.T., Sontag E.D. On the computational power of neural nets // Journal of Computer and System Sciences. 1995. V. 50. № 1. P. 132-150.
- Brette R. et al. Simulation of networks of spiking neurons: a review of tools and strategies // Journal of Computational Neuroscience. 2007. V. 23. № 3. P. 349-398.
- Smith E., Lewicki M.S. Efficient coding of time-relative structure using spikes // Neural Computation. 2004. V. 17. P. 19-45.
- Rozhnov A.V., Zharkov I.D. Algoritmizatsiya intellektual'noy obrabotki dannykh v zadachakh slabo formal'nykh sistem // Neyrokomp'yutery: razrabotka, primenenie. 2008. № 1-2. S. 35-42.