350 rub
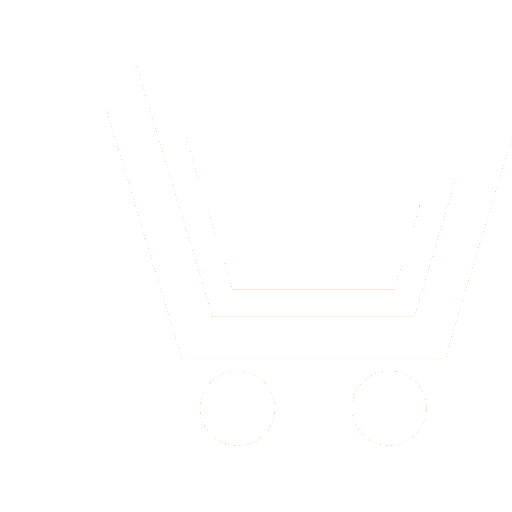
Journal Neurocomputers №2 for 2014 г.
Article in number:
Neural network methods for the problems of cluster analysis
Keywords:
cluster analysis
model Kuramoto
self-organized map
chaotic neural networks
oscillatory neural networks
Authors:
A.V. Novikov - Post-graduate Student, St.-Petersburg State Polytechnical University. E-mail: spb.andr@yandex.ru
E.N. Benderskaya - Ph.D. (Eng.), Professor, St.-Petersburg State Polytechnical University. E-mail: helen.bend@gmail.com
E.N. Benderskaya - Ph.D. (Eng.), Professor, St.-Petersburg State Polytechnical University. E-mail: helen.bend@gmail.com
Abstract:
The article presents results of research of neural networks methods for solving problems of cluster analysis. Described general problems of input data pre-processing and output data post-processing.
The content of article is divided into three main parts. In first part present presented study results as well as features and restrictions of usage oscillatory neural networks based on Kuramoto model with dynamic and static structures. The second and third parts contain study results of self-organized maps and chaotic neural networks accordingly.
In conclusion summarized the major features and proposed practical recommendation for usage neural networks for cluster analysis.
Pages: 48-53
References
- Ultsch A. Clustering with SOM: U*C // Workshop on Self Organizing Feature Maps. 2005. R. 31-37.
- Ultsch A. U*-Matrix: a Tool to visualize Clusters in high dimensional Data // Technical Report 36, CS Department, Philipps-University Marburg, Germany, 2004.
- Benderskaya E.N., Zhukova S.V. Osczillyatorny'e nejronny'e seti s xaoticheskoj dinamikoj v zadachax klasternogo analiza // Nejrokomp'yutery': razrabotka, primenenie. 2011. № 7. S. 74-86.
- Kazanovich Ja.B., Shmatchenko V.V. Osczillyatorny'e nejrosetevy'e modeli segmentaczii izobrazhenij i zritel'nogo vnimaniya // Nauch. sessiya MIFI 2004. Ch. 1. Nejroinformatika 2004. Lekczii po nejroinformatike. 2004. S. 15-68.
- Benderskaya E.N., Zhukova S.V. Fragmentary synchronization in chaotic neural network and data mining, Proceedings of 4th international conference on Hybrid artificial intelligence systems, Lecture Notes in Computer Science. 2009. V. 5572. Springer-Verlag. P. 319-326.
- Bohn C., Plant C., Shao J., Yang Q. Clustering by synchronization // KDD -10 Proceeding of the 16th ACM SIGKDD international conference of Knowledge discovery and data mining. 2010. P. 583-592.
- Cumin D., Unsworth C.P. Generalizing the Kuramoto Model for the Study of Neuronal Synchronisation in the Brain // Report University of Auckland School of Engineering. 638. 2006.
- Kohonen T. The Self-Organizing Map // Proceedings of the IEEE. 1990. V. 78. №. 9. P. 1464-1480.
- Kohonen T., Simula O., Visa A., Kangas J. Engineering Applications of the Self-Organizing Map // Proceeding of the IEEE. 1996. V. 84, № 10. P. 1358-1384.
- Kuramoto Y. Chemical Oscillations Waves, and Turbulence / Springer-Verlag Berlin Neidelberg New York Tokyo: 1984. 157 p.
- Miyano T., Tsutsui T. Data Synchronization as a Method of Data Mining // International Symposium on Nonlinear Theory and its Applications. 2007.
- Sprott J.C. Chaos and time-series analysis, Oxford University Press. 2003. P. 507.
- Ultshc A. Clustering with SOM: U*C // Workshop on Self Organizing Feature Maps. 2005. P. 31-37.
- Ultsch A. U*-Matrix: a Tool to visualize Clusters in high dimensional Data // Technical Report 36. CS Department. Philipps-University Marburg. Germany. 2004.
- Krivonozhko V.Ye., Rozhnov A.V., Lychev A.V. Postroenie gibridnykh intellektual'nykh informatsionnykh sred i komponentov ekspertnykh sistem na osnove obobshchyennoy modeli analiza sredy funktsionirovaniya // Neyrokomp'yutery: razrabotka, primenenie. 2013. № 6. S. 3-12.