350 rub
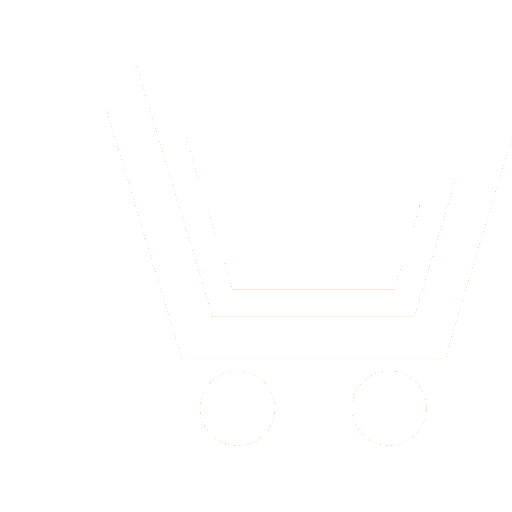
Journal Neurocomputers №8 for 2013 г.
Article in number:
Problems of modeling brain functions (Neural cybernetic approach)
Keywords:
algorithmic model of the prediction mechanism
neural similar systems
model operationality
sequence learning
unconscious learning
procedural memory
functional systems theory
Authors:
E.A. Umryukhin
Abstract:
In the article on the conceptual level of a nominal value advances made generalizations of neuroscience about the brain mechanisms of goal-directed behavior. The main results of the experimental study (based on the model) consistent training people were published in the 70s (Umryuhin E.A., 1972, 1976). At the same time it was shown that an intuitive unconscious acquisition and use information by person (Umryuhin E.A., 1972, 1976, 1979) has the principal role in such behavior and training. In the foreign literature the theme of sequence learning and experimental study of unconscious learning are remaining largely outside of specific problems scope still to be solved by mathematicians working in the field of neural network models and neuroinformatics, artificial intelligence. The results of modern ideas analyzes about this complex of problems with both neurophysiological side, and from the neurocybernetics are presented. The main problems of natural and artificial intelligence understanding are formulated, and brain generation examples of fundamentally new products are shown. It is suggested that the higher creative expressions of the human intellect are new science area. The area requires a fundamentally different approaches to the understanding of the phenomena compared with the usual approaches (deterministic Turing, mathematical). The novelty of this approach should be first of all in clear understanding of the nature of the emergent properties of the human intellect. The emergence of intelligence can be understood in terms of creative and largely unpredictable nature of the properties themselves intellect caused by special mechanisms of the brain. The unpredictability of creative intelligence in this case is means the output description of its properties beyond the existing mathematical paradigm in which the prediction of future behavior of the system trajectories (trajectories in the broadest sense of the word) is based on strict rules of the game (including the probabilistic or stochastic) on rules emerged as one of the ways to reflect the reality of the human brain with the help of modern mathematics.
Pages: 54-59
References
- Red'ko V.G. Problemy' intellektual'nogo upravleniya - obshhesistemny'e, e'volyuczionny'e i nejrosetevy'e aspekty' (priglashenie k kruglomu stolu) http://spkurdyumov.narod.ru/reeedddko.htm
- Sudakov K.V. Sistemny'e osnovy' intellekta (stat'ya predstavlennaya dlya kruglogo stola). Informaczionny'j fenomen zhiznedeyatel'nosti M. 1999; Sudakov K.V. Obshhaya teoriya funkczional'ny'x sistem. M.: Mediczina. 1984. Novy'e princzipy' i polozheniya v teorii funkczional'ny'x sistem, razrabotanny'e K.V. Sudakovy'm i ego shkoloj - Material, predstavlenny'j na krugly'j stol.
- Umryuxin E.A. Mexanizmy' mozga - informaczionnaya model' i optimizacziya obucheniya. M.: Gorizont. 1999. Umryuxin E.A. (ssy'lki na drugie upominaemy'e v tekste raboty' avtora soderzhatsya v e'toj knige, eaumin@mail.ru). Modelirovanie i ob''ektivnaya oczenka sistemny'x mexanizmov psixicheskoj deyatel'nosti // V kn.: Aleksandrov Ju.I., Brushlinskij A.V., Sudakov K.V., Umryuxin E.A. Sistemny'e aspekty' psixicheskoj deyatel'nosti. M.: E'ditorial URSS. 1999.
- Nissen M.J., Bullerner P. Attentional requirements of learning: evidence from performance measures // Cognitive psychol. 1987. V. 19; Schacter D.L. Implicit memory: history and current status // J. Exp. Psychol.: Learn. Mem. Cogn. 1987. 13. P. 501-518; Schacter D.L. Understanding implicit memory: a cognitive neuroscience perspective // Am. Psychol. 1992. 47. P. 559-569; Squire L.R. Declarative and nondeclarative memory: multiple brain systems supporting learning and memory. In: Memory systems. London: Bradford Book. 1994. Barretto G.A., Araujo A.F. Unsupervised learning and recall of temporal sequences: an application to robotics // Int. J Neural Syst. 1999. V 9. №3. P. 235-242; Cleeremans A. Mechanisms of implicit learning: connectionist models of sequential processing. MIT Press. 1993; Hikosaka O., Nakahara H., Rand M.K., Sakai K., Lu X., Nakamura K., Miyachi S., Doya K. Parallel neural networks for learning sequential procedures // Trends Neurosci. 1999. V. 22. №10. P. 464-471; Koch I., Hoffmann J. Patterns, chunks, and hierarchies in serial reaction-time tasks // Psychol. Res. 2000. V. 63. №1. P. 22-35; Heuer H., Schmidtke V., Kleinsorge T. Implicit learning of sequences of tasks // J. Exp. Psychol. Learn. Mem. Cogn. 2001. V 27. №4. P. 967-983.
- Schultz W., Dayan P., Montague P.R. A neural substrate of prediction and reward // Science. 1997. 125. P. 1593-1599; Ambrose-Ingerson J., Granger R., Lynch C. Simulation of Paleocortex perfoms hierarchical clustering // Nature. 1999. 247. 4948. P. 1344-48.
- Dehaene S., Changeux J.P. A hierarchical neuronal network for planning behavior. Proc. Nat. Acad Sciences USA. 94. 13293-13298, 1997. Dehaene S., Changeux J.P. Reward-dependent learning in neuronal network for planning and decision making. Prog. Brain Res. 126, 217-229. 2000. Grossberg S. The link between brain learning, attention and consciousness. Conscious Cogn. 1999. 8. 1. P. 1-4 4.
- Umryuxin E.A., Sudakov K.V. Teoriya xaosa: organizuyushhaya rol' funkczional'ny'x sistem // Rossijskij fiziologicheskij zhurnal im. I.M. Sechenova. 1997. T. 83. № 5-6. S. 190-216.
- Nejroinformatika-99. Diskussiya o nejrokomp'yuterax. M.: MIFI. 2000.
- Umryuxin E.A. Informaczionnaya model' sistemnoj organizaczii deyatel'nosti mozga // Mozg: teoreticheskie i klinicheskie aspekty'. M.: Mediczina. 2003.
- Sudakov K.V., Umryuxin E.A. Komp'yuternaya model' oczenki sistemnoj organizaczii psixicheskoj deyatel'nosti cheloveka // Nejrokomp'yutery': razrabotka, primenenie. 2012. №1. S. 48-53.