350 rub
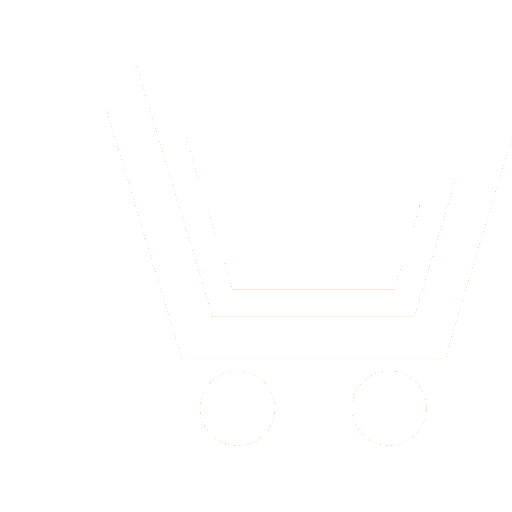
Journal Neurocomputers №11 for 2013 г.
Article in number:
The solution of problem of identification of nonlinear discrete stationary dynamic object identification by use a multilayer neural network (MLNN)
Keywords:
nonlinear
discrete dynamic object
identification
multilayer neural network
neural network training
prediction
Authors:
R. A. Fayzrakhmanov - Dr.Sc. (Econ.), Professor, Head of Department, Perm National Research Polytechnic University
I. N. Lipatov - Ph.D. (Eng.), Associate Professor, Perm National Research Polytechnic University
Е. V. Dolgova - Dr.Sc. (Econ.), Professor, Head of Department, Perm National Research Polytechnic University
I. N. Lipatov - Ph.D. (Eng.), Associate Professor, Perm National Research Polytechnic University
Е. V. Dolgova - Dr.Sc. (Econ.), Professor, Head of Department, Perm National Research Polytechnic University
Abstract:
The current state of the theory and practice of identification is characterized by intensive development of the statistical methods focused on computer data processing. Inverse problems are characterized ill-posedness. Many known computing schemes are unstable even at insignificant operational margin of data. Therefore the problem of an incorrectness is important at static identification of dynamic objects. The model of neuronetwork identification of nonlinear discrete dynamic object are given in this article. For example, it is power consumption system which casual deviations influence. Coefficients of model are calculated by means of the discrete filter of the bottom frequencies of Butterworth. The Neural Network Toolbox system is used during modeling. Parameters of neuronal model are optimized on this basis.
Five tests with method of Levenberg-Marquard use were carried out. In each test modelled a multilayer neuronal network with various initial values of weight coefficients. Results of these tests are described in article. Mean square deviations for a test set and training set differ slightly. Therefore the neuronal network model is adequate to a task and is suitable for practical use. The trained model is capable to generalize results for new supervision. This model correctly describes real nonlinear object.
Pages: 89-95
References
- Grop D. Metody' identifikaczii sistem / per. s angl. M.: Mir. 1979.
- Dejch A.M. Metody' identifikaczii dinamicheskix ob''ektov. M.: E'nergiya. 1974.
- E'jkxoff P. Osnovy' identifikaczii sistem upravleniya. M.: Mir. 1975.
- Fajzraxmanov R.A., Lipatov I.N. Cifrovoe modelirovanie sluchajnogo proczessa s ispol'zovaniem formiruyushhego fil'tra // Vestnik IzhGTU. 2011. № 1. S. 135-138.
- L'yung L. Identifikacziya sistem. Teoriya dlya pol'zovatel / per s angl. / pod red. Ja.Z. Cy'pkina. M.: Nauka. 1991.
- Metody' robastnogo, nejro-nechetkogo i adaptivnogo upravleniya: Uchebnik / pod red. N.D. Egupova. M.: Izd-vo MGTU im. N.E'. Baumana. 2002.
- D'yakonov V., Kruglov V. MatLab. Analiz, identifikacziya i modelirovanie sistem. Speczial'ny'j spravochnik. SPb.: Piter. 2002.
- Sergienko A.B. Cifrovaya obrabotka signalov. SPb.: Piter. 2006.
- Medvedev V.S., Potemkin V.G. Nejronny'e seti. MatLab 6 / pod red. V.G. Potemkina. M.: Dialog-MIFI. 2002.
- Kruglov V.V., Dli M.I., Golunov R.Ju. Nechetkaya logika i iskusstvenny'e nejronny'e seti. M.: Fizmatgiz. 2001.