350 rub
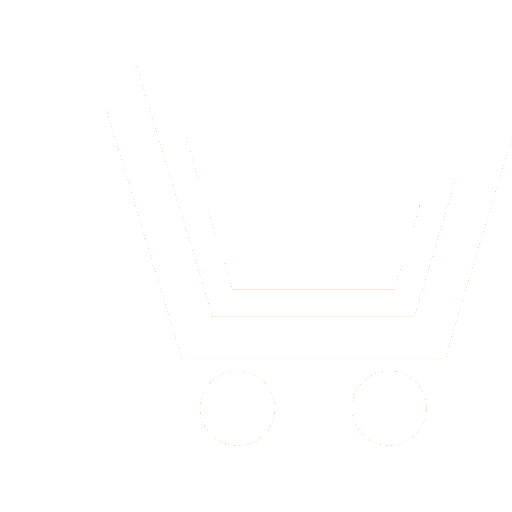
Journal Neurocomputers №10 for 2013 г.
Article in number:
The automated program complex for testing of speaker verification algorithms
Authors:
Yu.G. Spazhakin - Dr.Sci. (Eng.), Professor, Vladimir State University. E-mail: ludm@vlsu.ru
L.T. Sushkova - Post-graduate Student, Vladimir State University. E-mail: nwp_@mail.ru
L.T. Sushkova - Post-graduate Student, Vladimir State University. E-mail: nwp_@mail.ru
Abstract:
During this work algorithms of verification, such as Bayes's solving criterion, discriminant functions and models of the hidden Markov processes were studied. According to these researches and the chart in drawing 13 it was received that HMM possess the greatest accuracy among the presented algorithms, the maximum value of an error of the first and second sort in was made by 2% and 0,16% respectively whereas for other systems these values are much higher.
HMM are more preferable in comparison with Bayes's criterion and discriminant functions as the last model a voice path of the announcer worse, considering only voiced sites of voice signal. Bayes's criterion and discriminant functions in this work were based on a method of reference points. Sites of a speech signal with the greatest short-term energy - the sites of voiced speech corresponding to vowel sounds are called as reference points. At inclusion in the analysis of not voiced speech there is a sharp decrease in accuracy of work of system of verification because of noise and imposing of the next sounds. For this reason these criteria don't estimate all phrase entirely whereas HMM analyze all speech message that allows to consider at recognition a manner of pronouncing by the announcer of voice teams. However because all voice message volume of data for the analysis and calculations is considered increases in proportion to duration of voice signal.
As showed experiments, models of the hidden Markov processes, algorithms of determination of parameters and comparison of models show very high precision of work, but enough big computing expenses thus demand. Training of models occupies the greatest part of time as the program during this moment operates with big data files, than at verification. Verification of voice team on time borrows about one second whereas training of model can take about an hour depending on a training sample. Time of training can be lowered at the expense of fine tuning of process of training under demanded accuracy of verification by a way of reduction of a training sample, number of parameters describing a voice path etc.
The comparative analysis which allows to make the developed tool means, gives the chance to choose this or that method of verification for the solution of specific objectives as at a method choice more often the accent becomes on accuracy of work, complexity of algorithm, feature of operation and cost of system of verification. This program complex allows to estimate accuracy and features of operation. At level of design of a program complex it is possible to judge complexity of realization of algorithms, from work with a complex of it it is impossible to tell.
Pages: 28-38
References
- Axmad X.M., Zhirkov V.F. Vvedenie v czifrovuyu obrabotku rechevy'x signalov: Ucheb. posobie. Vladimir: Izd-vo Vladim. gos. un-ta. 2007.
- Bojkov F.G., Starozhilova T.K. Primenenie vejvlet-analiza signala v sisteme raspoznavaniya rechi / Doklady' XI Vseros. konf. «Matematicheskie metody' raspoznavaniya obrazov». M.: 2003. S. 247-250.
- Galunov V.I. Verifikacziya i identifikacziya govoryashhego. S-Peterburgskij gos. un-t. 2007. http://www.auditech.ru/ article/cntrid/click.php-action=download&id=21
- Geppener V.V., Xajdar A.S. Sravnitel'noe issledovanie e'ffektivnosti razlichny'x opisanij rechevy'x signalov v zadache verifikaczii diktorov // Doklady' 11-j Vseros. konf. «Matematicheskie metody' raspoznavaniya obrazov». M.: 2003.
- Zubov G.N., Xitrov M.V. «Centr rechevy'x texnologij». Sostoyanie i perspektivy' golosovoj biometrii // Voice Biometrics Conference. 2007.
- Levin A.M., Ul'dinovich S.V. Osobennosti realizaczii sistem identifikaczii diktora // Trudy' 10-j Mezhdunar. nauch.-texn. konf. «Cifrovaya obrabotka signalov i ee primenenie - DSPA-2008». M.: 2008.
- Levin E.K. E'ffektivnoe kodirovanie i raspoznavanie rechevy'x signalov: Metod. ukazaniya k lab. rabotam. Vladimir: Vladim. gos. un-t. 2002.
- Leonovich A.A. Vejvlet-obrabotka rechevy'x signalov v raspoznavanii rechi // Trudy' V Vseros. konf. molody'x ucheny'x po mat. modelirovaniyu i informaczionny'm texnologiyam s uchastiem inostranny'x ucheny'x. IVT SO RAN. 2004. http://www.ict.nsc.ru/ws/YM2004/8532/Leonovich_Title.htm.
- Leonovich A.A. Modul' raspoznavaniya rechi v sisteme MATLAB // Trudy' II Vseros. nauch. konf. «Proektirovanie inzhenerny'x i nauchny'x prilozhenij v srede MATLAB». M.: IPU RAN. 2004.
- Mixajlov V.G., Zlatoustova L.V. Izmerenie parametrov rechi / Pod red. M.A. Sapozhkova. M.: Radio i svyaz'. 1979.
- Pervushin E.A. Obzor osnovny'x metodov raspoznavaniya diktorov // Matematicheskie struktury' i modelirovanie. 2011. Vy'p. 24. S. 41-54.
- Proxorov A. Sistemy' avtomaticheskogo raspoznavaniya rechi. Komp'yuter Press. 2003. № 7(163).
- Rabiner L.R. Skry'ty'e Markovskie modeli i ix primenenie v izbranny'x prilozheniyax pri raspoznavanii rechi: Obzor // TIIE'R. 1989. T. 77. № 2. S. 86-120.
- Ronzhin A.L., Karpov A.A., Li I.V. Rechevoj i mnogomodal'ny'j interfejsy'. M.: Nauka. 2006.
- Sady'xov R.X., Rakush V.V. Modeli gausovskix smesej dlya verifikaczii diktora po proizvol'noj rechi // Doklady' BGUIR № 4 Belorusskij gos. un-t informatiki i radioe'lektroniki. Minsk. 2003
- Sarana D.V., Ikonin S.Ju. Sistema avtomaticheskogo raspoznaniya rechi SpiritASREngine // Cifrovaya obrabotka signalov. 2003. № 4.
- Sergienko A.B. Cifrovaya obrabotka signalov. Uchebnik dlya vuzov. Izd. 2-e. Piter. 2006.
- Sorokin V.N., V'yugin V.V., Tanany'kin A.A. Raspoznavanie lichnosti po golosu: Analiticheskij obzor. Informaczionny'e texnologii v texnicheskix i soczial'no e'konomicheskix sistemax // Informaczionny'e proczessy'. 2012. T. 12. № 1. S. 1-30.
- Spazhakin Ju.G., Sushkova L.T. Programmny'j kompleks dlya opredeleniya granicz rechi v zadachax avtomaticheskoj verifikaczii diktora // Biomediczinskaya radioe'lektronika. Biomediczinskie texnologii i radioe'lektronika. 2012. № 6. S. 68-77.
- Tkachenya A.V. Kiselyov V.V., Davy'dov A.G. Sistema opredeleniya e'moczional'nogo sostoyaniya diktora po golosu // Trudy' II mezhdunar. nauchno-texn. konf. «Otkry'ty'e semanticheskie texnologii proektirovaniya intellektual'ny'x sistem» OSTIS-2012. Minsk. 2012.
- Tu Dzh., Gonsales R. Princzipy' raspoznavaniya obrazov. M.: Mir. 1978.
- Sharij T.V. O probleme parametrizaczii rechevogo signala v sovremenny'x sistemax raspoznavaniya rechi // Vestnik Doneczkogo naczional'nogo universiteta. Ser. A: Prirodnichі nauki. 2008. Vy'p. 2
- Miller D. Senior Analyst, Opus research // Voice Biometrics Conference. Washington. 2007.
- Ghulam Muhammad. Extended average magnitude difference function based pitch detection // The International Arab Journal of Information Technology. 2011. V. 8. № 2.
- Young-Hwan Song, Doo-Heon Kyun, Jong-Kuk Kim, Myung-Jin Bae, On SNR Estimation by the Likelhood of near Pitch for Speech Detection. World Academy of Science, Engineering and Technology. 32. 2007.
- Rechevy'e texnologii. http://www.spirit.ru/
- Segmentacziya ry'nka biometricheskix texnologij. http://www.ibgweb.com.
- Solov'ev V.D. Texnologii izvlecheniya iz tekstov informaczii o soby'tiyax v real'nom vremeni // Nejrokomp'yutery': razrabotka, primenenie. 2013. № 1. S. 23.
- Koroticzkij A.V., Gromov S.V. Intellektual'naya sistema dlya analiza formovosstanovleniya v splavax s pamyat'yu formy' // Nejrokomp'yutery': razrabotka, primenenie. 2013. № 1. S. 68.
- Rudometkina M.N., Spiczin V.G., Romanenko V.V., E'tel' V.A. Razrabotka logicheskoj seti dlya morfologicheskoj modeli russkogo yazy'ka // Nejrokomp'yutery': razrabotka, primenenie. 2013. № 8. S. 22.