350 rub
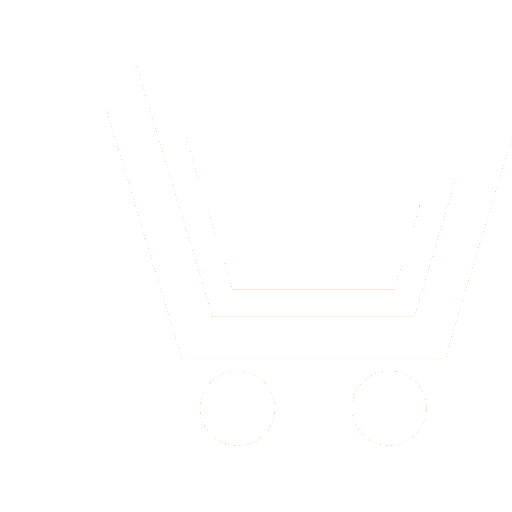
Journal Neurocomputers №10 for 2013 г.
Article in number:
The adaptation mechanism of finite ring neural network to the new module
Authors:
N.I. Chervyakov - Dr. Sc. (Eng.), Professor, North-Caucasian Federal University
M.S. Afonin - Post-Graduate Student, North-Caucasian Federal University
M.S. Afonin - Post-Graduate Student, North-Caucasian Federal University
Abstract:
In this study, for the first time the mechanism of adaptation of the finite rings neural network (FRNN). The specificity of this type of neural networks is to find the reduction of the division of integer digital signal input to the module, which is used to configure the network weights. Previously developed models were FRNN are oriented to training or retraining. Designed for certain applications of modular arithmetic data neural network is configured once before using it. However, there exist applications of modular arithmetic, which may increase the effectiveness of using the mechanism of adaptation of the neural network to the new module. To solve this problem, firstly, a new architecture FRNN oriented learning by example: the number of layers is fixed for every module, is excluded slow approach to the resulting value, the result is less in magnitude and does not require adjustment. Second, developed three training algorithm modified FRNN: training sample inputs and desired outputs, training only on the desired output and training only for the sample input.
Pages: 3-12
References
- Primenenie iskusstvenny'x nejronny'x setej i sistemy' ostatochny'x klassov v kriptografii. M.: Fizmatlit. 2012.
- Komarczova L.G., Maksimov A.V. Nejrokomp'yutery': Ucheb. posobie dlya vuzov. M.: Izd-vo MGTU im. N.E'. Baumana. 2002.
- Galushkin A.I. Nejrokomp'yuter. Kn. 3: Ucheb. posobie dlya vuzov. M.: IPRZhR. 2000.
- Ciet M., Neve M., Peeters E., Quisquater J.-J. Parallel FPGA Implementation of RSA with Residue Number Systems // Pro-ceedings of the 46th IEEE International Midwest Symposium on Circuits and Systems. 2003. V. 2. P. 806-810.
- Alkhoraidly A., Hasan M.A. Error detection and recovery for transient faults in elliptic curve cryptosystems // Technical Report CACR 2009-06. University of Waterloo. 2009.
- Afonin M.S. Obespechenie otkazoustojchivosti e'llipticheskoj kriptografii // Problemy' matematiki i radiofiziki v oblasti informaczionnoj bezopasnosti: I Vseros. konf. Severo-Kavkazskij federal'ny'j universitet (Stavropol', 17-19 oktyabrya 2012 g.). Stavropol': Fabula. 2012. S. 235-239.
- Siu K.-Y., Bruck J., Kailath T., Hofmeister T. Depth efficient neural networks for division and related problems // IEEE Transactions on Information Theory. 1993. V. 39. № 3. P. 946-956.
- Sun H., Yao T.-R. A neural-like network approach to residue-to-decimal conversion // IEEE International Conference on Neural Networks. IEEE World Congress on Computational Intelligence. 1994. V. 6. P. 3883-3887.
- Chervyakov N.I., Saxnyuk P.A., Shaposhnikov A.V., Makoxa A.N. Nejrokomp'yutery' v ostatochny'x klassax. Kn. 11: Ucheb. posobie dlya vuzov. M.: Radiotexnika. 2003.
- Keller T., Liew T.H., Hanzo L. Adaptive redundant residue number system coded multicarrier modulation // IEEE Journal on Selected Areas in Communications. 2000. V. 18. № 11. P. 2292-2301.
- Lee I., Jenkins W.K. The design of residue number system arithmetic units for a VLSI adaptive equalizer // Proceedings of the 8th Great Lakes Symposium on VLSI. 1998. P. 179-184.
- Grolmusz V., Tardos G. Lower bounds for (MOD p-MOD m) circuits // 39th Annual Symposium on Foundations of Computer Science. 1998. P. 279-288.
- Chervyakov N.I., Remizov S.L. Struktury' nejronny'x setej konechnogo kol'cza // Nejrokomp'yutery': razrabotka, primenenie. 2004. № 12. S. 21-30.
- Chervyakov N.I., Evdokimov A.A., Maslennikov E.V. Osobennosti nejrosetevoj kriptografii s otkry'ty'm klyuchom // Nejrokomp'yutery': razrabotka, primenenie. 2012. № 12.