350 rub
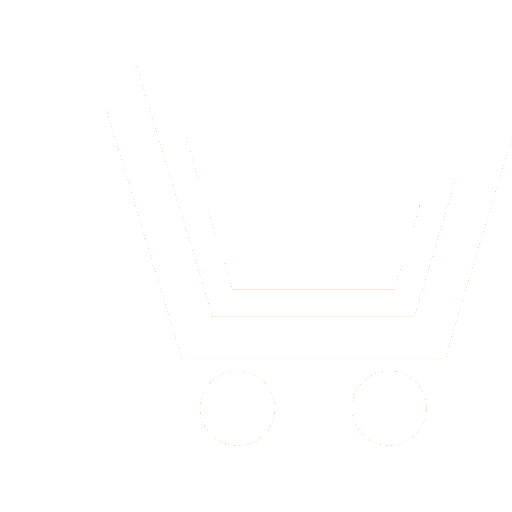
Journal Neurocomputers №9 for 2012 г.
Article in number:
Application of neural networks for solving inverse problems of image deconvolution and removal of distortions such as grease and defocusing
Keywords:
deconvolution of images
image processing
grease
blur
neural networks
neural network processing
Authors:
P.V. Skribtsov, P.A. Kazantsev, A.V. Dolgopolov
Abstract:
The article describes the application of neural nets to solve the inverse problem of deconvolution of images and distortions such as motion blur and defocusing. Proposed neural network model PSF, which allows for the deconvolution of complex cases such as distortion and defocusing of lubricant that does not quite match the standard model. Application of neural PSF can more accurately restore the image with distortions such as motion blur and defocusing.
Pages: 47-54
References
- Родионов С. А. Основы оптики. Конспект лекций. СПб: СПб ГИТМО (ТУ). 2000.
- Домненко В. М., Бурсов М. В. Моделирование формирования оптического изображения. Учеб. пособие. СПб: СПбГУИТМО. 2005.
- Charu Khare, Kapil Kumar Nagwanshi, Implementation and Analysis of Image Restoration Techniques // International Journal of Computer Trends and Technology. May to June Issue 2011.
- Dr. P.Subashini , Ms. M. Krishnaveni, Mr. Vijay Singh, Image Deblurring Using Back Propagation Neural Network // World of Computer Science and Information Technology Journal (WCSIT). 2011. V. 1. № 6. Р. 277-282.
- Нейрокомпьютеры в прикладных задачах обработки изображений // под ред. А.Н. Балухто, А.И. Галушкина. М.: Радиотехника. 2007.
- Хайкин С. Нейронные сети: полный курс. М.: Вильямс. 2006.
- Christopher M. Bishop, Neural Networks for Pattern Recognition // Oxford University Press. 2005.
- ООО «ПАВЛИН ТЕХНОЛОГИИ» / Решение обратных задач на основе математических моделей формирования пространственно-временных характеристик русел рек для оперативных краткосрочных прогнозов паводков и половодья с использованием многоядерных суперЭВМ. - Государственный контракт № 16.515.11.5004 от «29» апреля 2011 г., шифр 2011-1.5-515-020-037, Отчет о НИР, этап № 2 «Теоретические исследования поставленных перед НИР задач», 2011 г.
- Richardson, W. H., Bayesian-based iterative method of image restoration // Journal of The Optical Society of America. 62(1):55. 1972.
- Shepp, L. A. and Vardi, Y., Maximum likelihood reconstruction for emission tomography // IEEE Transactions on Medical Imaging. MI-2: 113-122. 1982.
- Landweber, L., An iteration formula for Fredholm integral equations of the first kind // American Journal of Mathematics. 73:615-624. 1951.
- Biemond, J., Lagendijk, R., and Mersereau, R., Iterative methods for image deblurring // The Proceedings of the IEEE. V. 78. № 5. 1990. P. 856-883.
- Vincent L., Soille P. Watersheds in Digital Spaces: An Efficient Algorithm Based on Immersion Simulations // IEEE Trans. on Pattern Analysis and Machine Intelligence. 1991. V. 13. № 6. Р. 583-598.