350 rub
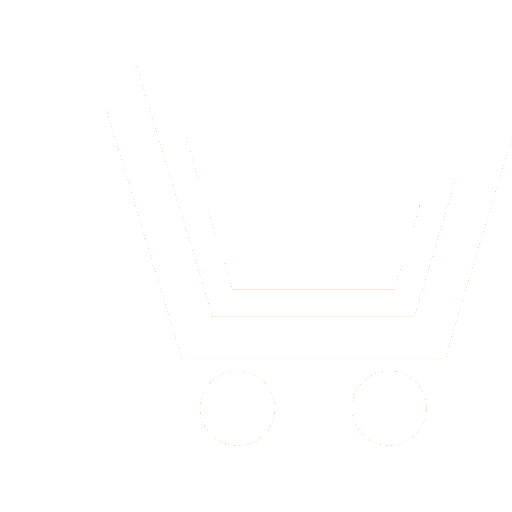
Journal Neurocomputers №6 for 2012 г.
Article in number:
Procedure for Defining Optimal Parameters of Wavelet Transform and Neural Network Classifier for Nonstationary Bio-radiolocation Signals Pattern Recognition
Keywords:
wavelet-transform
neural network classifier
pattern recognition
nonstationary signals
bio-radiolocation
Authors:
M.D. Alekhin
Abstract:
The synthesis of artificial intelligent recognition systems is the relevant direction of modern cybernetics. Automated recognition of patterns of non-stationary signals is especially in demand when creating different control sets and data processing sets in complex ergatic systems.
The basis of the developed procedure for determining the optimal parameters of the wavelet transform and neural network classifier to improve recognition of patterns of non-stationary signals can be divided into two main components. To extract the informative features using wavelet transform, you must first choose a general class of wavelets, and then form a bank of wavelet bases with a set of ordinal indexes for specific families of wavelets, determine the optimal level of decomposition, and then choose the best basis by searching for the minimum value of the modified entropy criterion.
At the stage of synthesis of neural network classifier with optimal properties, in particular the one for multi-layer perceptron with one hidden layer, after a preliminary estimate of the number of hidden neurons is searched such a value at which the maximum average classification accuracy is achieved, and then the best algorithm for neural network training is selected based on the selected indicators of quality.
The evaluating of the effectiveness of the proposed solutions was carried out on clinically verified database of breathing patterns of bio-radar transient signals correlated to the three classes: obstructive sleep apnea, central sleep apnea and normal restful sleep.
The feature space of patterns formed on the basis of the sequence of absolute values of the detailing coefficients of the third level of the wavelet decomposition of the bio-radar quadrature components signal. A multilayer perceptron with one hidden layer was used as a classifier.
In terms of the minimum value of the modified entropy criterion the best wavelet bases for the formation of feature space should be considered the basis Symlet 13, belonging to the general class of orthogonal wavelets with compact support.
Based on the highest average recognition accuracy by the results of ten simulations of neural network training procedure, the optimal number of neurons in the hidden layer of perceptron is 9, which also corresponds to the upper boundary estimate of the number of hidden neurons by a theorem of Arnold-Kolmogorov. The assessment based on modified entropy criterion is to be considered effective.
The best algorithm for neural network training was the Levenberg-Marquardt algorithm based on average values for each of the indicators of quality: the accuracy of classification on the test sample, the number of cycles of the algorithm, the time spent. For the most successful realization with the optimal parameters of the wavelet transformation and multilayer perceptron the maximum recognition accuracy of respiratory patterns of non-stationary bio-radar signals on the test sample was 86,7 % with average accuracy of 84,2 % based on the results of ten simulations of neural network classifier operation.
Pages: 46-54
References
- Искусственный интеллект. В 3-х кн. Кн. 2. Модели и методы: Справочник / Под ред. Д.А. Поспелова. М.: Радио и связь. 1990. 304 с.
- Журавлёв Ю.И., Рязанов В.В., Сенько О.В.«Распознавание». Математические методы. Программная система. Практические применения. М.: ФАЗИС. 2006. 176 с.
- Ушаков И.Б., Богомолов А.В., Кукушкин Ю.А. Паттерны функциональных состояний оператора. М.: Наука. 2010. 390 с.
- Nonlinear and Nonstationary Signal Processing / Ed. by W.J. Fitzgerald, R.L. Smith, A.T. Walden, P.C. Young. Cambridge University Press. 2000. 471 p.
- Bishop C.M. Pattern Recognition and Machine Learning. Springer. 2007. 749 p.
- Митрофанов Д.Г., Сафонов А.В. Применение нейросетевой технологии для распознавания целей по радиолокационным изображениям // Нейрокомпьютеры: разработка, применение. 2006. №3. С. 60 - 68.
- American Sleep Disorders Association Standards of Practice Committee Practice Parameters for the Indications for Polysomnography and Related Procedures. Polysomnography Task Force // Sleep. 1997. V. 20. P. 406 - 422.
- Chesson A.L., Berry R.B. Practice Parameters for the Use of Portable Monitoring Devices in the Investigation of Suspected Obstructive Sleep Apnea in Adults // Sleep. 2003. V. 26. P. 907 - 913.
- Биорадиолокация / Под ред. А.С. Бугаева, С.И. Ивашова, И.Я. Иммореева. М.: Изд-во МГТУ им. Н.Э. Баумана. 2010. 396 с.
- Алёхин М.Д., Анищенко Л.Н., Журавлев А.В.Метод классификации дыхательных паттернов биорадиолокационного сигнала на основе искусственных нейронных сетей и вейвлет анализа // Биомедицинская радиоэлектроника. 2011. № 10. С. 57 - 64.
- Bulayev V.I. Acoustic Logging Data Compression // Proc. of the 7th International Workshop on Computer Science and Information Technologies. Ufa, Russia. 2005. V. 3. P. 240 - 241.
- Makiela W. An Evaluation of Irregularities of Milled Surfaces by the Wavelet Analysis // Manufacturing Engineering. 2010. V. 9. P. 22 - 26.
- Алёхин М.Д., Анищенко Л.Н., Журавлев А.В. Кратномасштабный вейвлет-анализ в формировании пространства признаков двигательных паттернов биорадиолокационного сигнала // Радиотехника. 2011. № 11. С. 20 - 25.
- Щеглов И.Н., Богомолов А.В., Печатнов Ю.А. Исследование влияния репрезентативности обучающей выборки на качество работы методов распознавания образов // Нейрокомпьютеры: разработка, применение. 2002. № 9 - 10. С. 3 - 9.
- Смоленцев Н.К. Основы теории вейвлетов. Вейвлеты в MATLAB. М.: ДМК Пресс. 2005. 304 с.
- Медведев В.С., Потемкин В.Г. Нейронные сети. MATLAB 6. М.: Диалог-МИФИ. 2002. 496 с.