350 rub
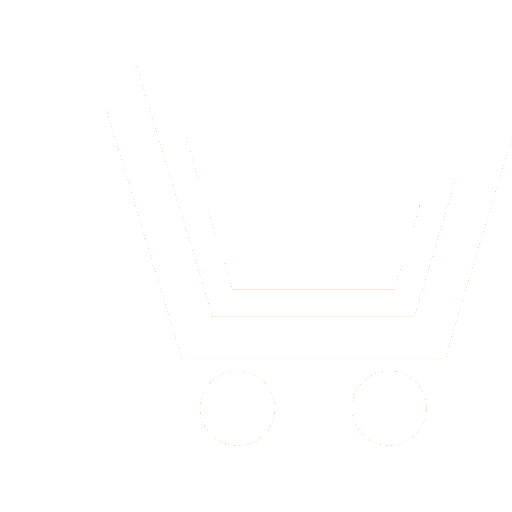
Journal Neurocomputers №2 for 2012 г.
Article in number:
Neural network modeling of heat supply systems
Authors:
A.E. Misnik
Abstract:
District heating system is a complex of various buildings, plants and equipment, technologically interconnected with process of production, transportation, distribution and consumption of thermal energy.
With limited energy resources, rational consumption and effective management are tasks of great importance. To ensure efficient use of resources you need to know how the system will behave under different conditions, and have the ability to efficiently manage it, that is possible only with automated control system. Construction of the automated control system requires reliable model of the heating system.
We propose a hybrid approach to modeling of these systems, the essence of which is as follows.
The initial modeling of heat supply system on the basis of structural matrix method is performed, the result is determined by the incidence matrix of nodes and branches.
After that, we construct a system of equations (based on Bernoulli's equation) describing the state of the system. The system of equations is solved using the method of gradient descent. By varying of initial data, we obtain values of the system states.
However, each cycle of calculations requires a significant amount of time. In this situation, to find the optimal state of the system, it is proposed to use neural network approach, in which each structural element of the heating system is described by a single neural network, inputs and outputs of which are connected in according to previously obtained the incidence matrix.
To simulate the structural elements of the heating system the library of typical neural modules is designed.
In accordance with the results of simulation runs training of these neural networks. Implementation and training of the typical neural modules are based on the developed dynamic link library.
When the structural changes in the existing model are happen, the new typical elements are added, and then the model is immediately ready for a "use". In this case additional training of neural modules is running on a working model.
Process of additional training of neural modules is controlled by supervisor, which is also an artificial neural network, the inputs of which is fed all the raw data, and outputs are the parameters of the system, similar to the outputs of elements: technical restrictions and the amount of heat.
A neural network-supervisor and the boundary data from analyzing of structure-matrix method is used to identify possible errors in structure or training mistakes.
The proposed combined approach to the modeling of heat supply systems based on a hierarchical neural network model and developed simulation tools can significantly improve quality and efficiency of management decisions, as well as the flexibility of modeling and simulation capabilities at the expense of structural and parametric optimization of model based on the information on the heating system, obtained from the real time database
Pages: 62-73
References
- Соколов Е.Я. Теплофикация и тепловые сети: Учебник для вузов. Изд. 8-е, стереот. М.: МЭИ. 2006.
- Тарасик В.П. Математическое моделирование технических систем: Учебник для вузов. Минск: ДизайнПРО. 2004.
- Хайкин С. Нейронные сети: полный курс. Изд. 2-е, испр.: Пер. с англ. М.: Издательский дом «Вильямс». 2006.
- Борисов В.В., Мисник А.Е. Иерархическая нейросетевая модель систем теплоснабжения // Энергетика, Информатика, Инновации-2011. ЭИИ-2011: Сб. трудов Междунар. науч.-техн. конф. В 2 т. Т. 2. Секции 3, 4, 5. Смоленск: РИО филиала ГОУВПО МЭИ(ТУ) в г. Смоленске. 2011. С. 205-207.
- Борисов В. В., Федулов А. С. Способы гибридизации нечетных и нейронный сетей // Нейрокомпьютеры: разработка, применение. 2007. № 1. С. 5 - 11.