350 rub
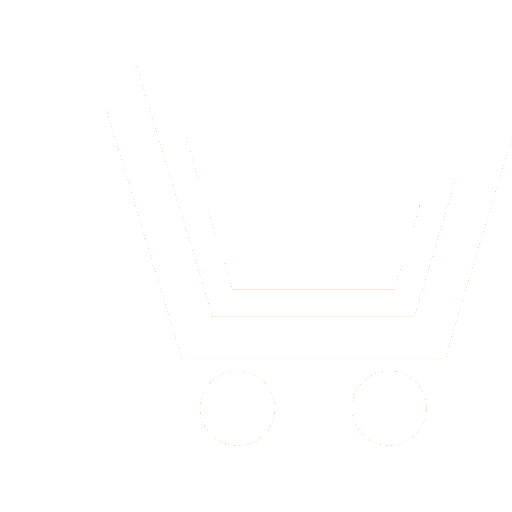
Journal Neurocomputers №10 for 2012 г.
Article in number:
Neural networks for the construction of the permissibility bounds in the «structure - property» problem
Keywords:
artificial neural networks
pattern recognition
classification
«structure - property» problem
QSAR (Quantitative Structure - Activity Relationship)
Authors:
E.I. Prokhorov
Abstract:
The work is devoted to the methods of predicting the properties of chemical compounds («structure - property» problem). As part of the solution of the main problem author proposes formulation of the problem of constructing the recognizing models and the bounds of permissibility for them. Problem of constructing the bounds of permissibility reduces to the problem of classification, called in this paper the problem of the second level. We give the necessary theoretical basis for this approach and show the advantages of using artificial neural networks with one output neuron for the classification of the second level. In the present article the author discusses the adaptation of the description of the training sample for solving the original problem and the problem of the second level. As a method to adapt the description of the training sample we propose the evolutionary selection of descriptors. As a result of adaptation for calculating bounds of permissibility of the ANN we use its special space of descriptors. Among other things we show the necessity of evolutionary selection as a means of reducing the original space of descriptors for solving the problem of overtraining when using neural networks. Moreover, using the adaptive descriptions for calculating bounds of permissibility greatly speeds up the screening of large databases of unexplored compounds. The features of using the artificial neural networks for classification problems in chemistry are described. The necessary recommendations on the choice of architecture of neural networks to solve «structure - property» problem are given. The paper proposes general methodology of research in the prediction of properties of unexplored chemical compounds using artificial neural networks. We describe an approach to the consistent prediction by using sets of recognizing models and its bounds of permissibility. Current paper includes the results of testing of this approach in the analysis of a sample of enzyme inhibitors of cell division.
Pages: 46-56
References
- Джурс О. П., Айзенауэр Т. Распознавание образов в химии: Пер.с англ. М.: Мир. 1977.
- Стьюпер Э., Брюггер У., Джурс П. Машинный анализ связи химической структуры и биологической активности: Пер.с англ. М.: Мир. 1982.
- Baskin, I. I., Palyulin, V. A., Zefirov, N. S., NASA. A computer program for performing QSAR/QSPR studies using artificial neural networks // QSAR and Molecular Modeling: Concepts, Computational Tools and Biological Applications. Barcelona: Prous Science Publ. 1995. P. 30-31.
- Baskin I. I., Keshtova S. V., Palyulin V. A., Zefirov N. S.Combining Molecular Modeling with the Use of Artificial Neural Networks as an Approach to Predict Substituent Constants and Bioactivity // Molecular Modeling and Prediction of Bioactivity; K. Gundertofte; F.S. Jorgensen, Eds. Klumer Academic / Plenum Publishers: NY. 2000. Р. 468-469.
- Prokhorov E. I., Ponomareva L. A., Permyakov E. A. and Kumskov, M. I., Fuzzy classification and fast rules for refusal in the QSAR problem // Pattern Recognition and Image Analysis, 2011. Vol. 21. Number 3. P. 542-544.
- Прохоров Е. И. «Нечеткое» прогнозирование свойств химических соединений: Использование нечеткой функции классификации на кластерах обучающего множества в задаче «структура - свойство», Saarbrucken, Germany: LAPLambertAcademicPublishing. 2012.
- Прохоров Е. И., Перевозников А. В., Пономарева Л. А. Кумсков М. И.Нейронная сеть как инструмент реализации кусочно-линейного классификатора при массовом скрининге молекул в задаче «структура - свойство» // Нейрокомпьютеры: разработка, применение. № 3. С. 39-45.
- Гиллер С. А., Глаз А. Б., Растригин Л. А., Розенблит А. Б.Распознавание физиологической активности химических соединений на перцептроне со случайной адаптацией структуры // ДАН СССР. 1971. Т. 199. № 4. С. 851-853.
- Hiller, S. A., Golender, V. E., Rosenblit, A. B., Rastrigin, L. A., Glaz, A. B.,Cybernetic methods of drug design. I. Statement of the problem - the perceptron approach // Comput. Biomed. Res. 1973. V. 6. № 5. P. 411-421.
- Zupan, J., Gasteiger, J.,Neural networks: a new method for solving chemical problems or just a passing phase - // Anal. Chim. Acta. 1991. V. 248. № 1. С. 1-30.
- Минский М., Пейперт С.Персептроны. М.: Мир. 1971.
- Стариков А. Применение нейронных сетей для задач классификации [Электронный ресурс] (URL: http://www.basegroup.ru/library/analysis/neural/classification/дата обращения 14.06.2012).
- Кумсков М. И., Митюшев Д. Ф. Применение метода группового учета аргументов для построения коллективных оценок свойств органических соединений на основе индуктивного перебора их «структурных спектров» // Проблемы управления и информатики. 1996. № 4. С.127-149.
- Kumskov, M.I., Zyryanov, I.L., Svitan?ko,I. V.,ANewMethodforRepresentingSpatialElectronicStructuresofMoleculesinthe Problem of Structure-Biological Activity Relationship. Pattern Recognition and Image Analysis. 1995. № 3. P. 477-484.
- Stone,M.,Cross-Validatory Choice and Assessment of Statistical Predictions. Journal of the Royal Statistical Society, B. 36.1974. P. 111-147.
- Вапник В.Н., Червоненкис А. Я. Теория распознавания образов (статистические проблемы обучения) М.: Наука. 1974.
- Кумсков М. И., Смоленский Е. А., Пономарева Л. А., Митюшев Д. Ф., Зефиров Н. С. Системы структурных дескрипторов для решения задач «структура - активность». ДокладыАкадемииНаук. 1994. 336. п. 1. С.64-66.
- Perevoznikov, A. V., Shestov A. M., Permyakov E. A., Kumskov M. I., A Way to Increase the Prediction Quality for the Large Set of Molecular Graphsby Using the k_NN Classifier // Pattern Recognition and Image Analysis. 2011. V. 21. № 3.P. 545-548.