350 rub
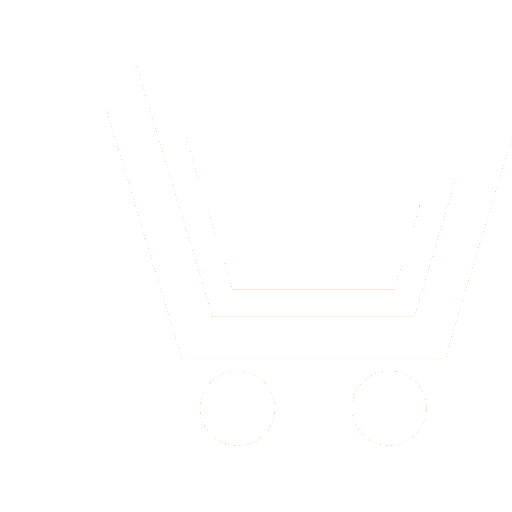
Journal Neurocomputers №7 for 2011 г.
Article in number:
Oscillatory neural networks with chaotic dynamics in application to cluster analysis
Authors:
E. N. Benderskaya, S. V. Zhukova
Abstract:
The development of up to date cluster analysis techniques that respond to requirements on real-time processing with high quality results of pattern recognition solutions still stands to be actual as the variety and velocity of data increased greatly since last decade.
One of promising trends in pattern recognition belongs to application of oscillatory neural networks with chaotic dynamics (OCNN). This class of neural networks attracts ones attention because of similarity to biological prototype (human brain) that consists in self-organized generalization of knowledge by means of inner synchronization emerging between highly unstable elements. Under main focus in this paper are the opportunities of OCNN application to general clustering problem solution when there is no a priori information about number and topology of clusters.
The phenomenology of oscillatory clusters formation for the first time was discovered by K. Kaneko when he modeled multidimensional difference equations. Chaotic globally coupled maps with homogeneous weak linkage generated abstract oscillatory clusters. The heterogeneous mean field assignment by means of triangulation metric allows inner synchronization tuning of chaotic neurons in order to obtain clustering solution without an expert opinion. The proposed method to reveal fragmentary synchronization (typical for OCNN) predetermined correct interpretations of chaotic oscillatory dynamics under the condition when there is no coincidence between instant states of neurons that belong to the same cluster neither by amplitude, nor by phase or fixed phase lag.
The role of chaos in fragmentary synchronization emergence was investigated. The substitution of logistic map to more simple transfer functions like sinus, logistic map with parameter = 1.3, circle map with parameters К = 3, Ω = 0.2 brings to coherent dynamics of all neurons that corresponds to negative clustering results (all objects are recognized to be in one cluster). On the opposite application of other well-known chaotic maps (Baker-s map, tent map, Gauss map, circle map with parameters К = 0.32 , Ω = 0.2) as transfer functions give evidence to the existence of OCNN set applica-ble to cluster analysis.
To demonstrate the advantages of new clustering method based on oscillatory neural network with chaotic dynamics the comparative analysis with 42 hierarchical clustering techniques and iterative k - means is given. Classical clustering techniques are based on inner (Euclidean, square Euclidean, Mahalanobis, Chebyshev, Minkovski, cityblock, cosine) and between clusters (single linkage, complete linkage, average linkage, centroid linkage, median linkage, weighted linkage) distance metrics. The advantages of OCNN clustering method were demonstrated on fundamental clustering problems suite: all test input datasets were clustered by one unified way with no mistakes.
OCNN embedding into automatic pattern recognition systems is considered to be possible after development of clustering technique hardware implementation.
Pages: 74-86
References
- Борисюк Г. Н., Борисюк Р. М., Казанович Я. Б., Лузянина Т. Б., Турова Т. С., Цымбалюк Г. С. Осцилляторные нейронные сети. Математические результаты и приложения // Математическое моделирование. 1992. Т. 4. № 1. С. 3-43.
- Борисюк Г. Н., Борисюк Р. М., Казанович Я. Б., Иваницкий Г. Р. Модели динамики нейронной активности при обработке информации мозгом - итоги «десятилетия» // Успехи физических наук. 2002. Т. 172. №10. C. 1189-1214.
- Лечебная электрическая стимуляция мозга и нервов человека / под общ. ред. акад. Н. П. Бехтеревой. Act ; Сова, М. - СПб. 2008.
- Магницкий Н. А., Сидоров С. В. Новые методы хаотической динамики. М.: УРСС. 2004.
- Кузнецов С. П. Динамический хаос. М.: Физматлит. 2001.
- Kaneko, K., Phenomenology of spatio-temporal chaos // Directions in chaos. Singapore. 1987. P. 272-353.
- Kaneko, K.,Сhaotic but regular posi-nega switch among coded attractors by cluster-size variations // Phys. Rev. Lett. 1989. № 63(14). P. 219-223.
- Потапов А. Б., Али М. К. Нелинейная динамика обработки информации в нейронных сетях // Известия высших учебных заведений. Прикладная нелинейная динамика. 2001. Т. 9. № 6. С. 3-44.
- Aihara, K., Takabe, Т., Toyoda, M.,Сhaotic Neural networks // Phys. Lett. 1990. A 144. C. 333-340.
- Adachi, М., Aihara, K., Associative dynamics in a chaotic neural network // Neural networks. 1997. № 10. P. 83-98.
- Абарбанель Г. Д., Рабинович М. И., Селверстон А., Баженов М. В., Хуэрта Р., Сущик М. М., Рубчинский Л. Л. Синхронизация в нейронных ансамблях // Успехи физических наук. 1996. № 166. C. 363-390.
- Журавлев Ю. И., Рязанов В. В., Сенько О. В.Распознавание. Математические методы. Программная система. Практические применения. М.: Фазис. 2006.
- Дюк В. Data mining: учебный курс, СПб.: Питер. 2001.
- Васильев В. И. Распознающие системы. Киев: Наукова Думка. 1984.
- Горелик А. Л., Скрипкин В. А. Методы распознавания. М.: Высшаяшкола. 2004.
- Ultsch, A., Density Estimation and Visualization for Data containing Clusters of unknown Structure // Proceedings 28th Annual Conference of the German Classification Society (GfKl 2004). Dortmund. Germany. Springer. Heidelberg. P. 232-239.
- Budayan, C., Dikmen, I., Birgonul, M. T., Comparing the performance of traditional cluster analysis, self-organizing maps and fuzzy C-means method for strategic grouping // Expert Systems with Applications. 2009. V. 36. P. 11772-11781.
- Ultsch, A., Clustering with SOM: U*C // Proc. Workshop on Self-Organizing Maps. 2005. Paris. France. P. 75-82.
- Борисюк Р. М., Чик Д., Казанович Я. Б. Осцилляторная модель зрительного внимания // Нейроинформатика-2010. XII Всероссийскаянаучно-техническаяконференция: Сб. науч. трудов, Т. 2. М.: МИФИ. С. 19-28.
- Raiko, T., Valpola, H., Oscillatory Neural Network for Image Segmentation with Biased Competition for Attention // From Brains to Systems, Preprints of the BICS 2010 Conference on Brain-Inspired Cognitive Systems 14-16 July 2010. Madrid. P. 208-219.
- Borisyuk, R, Denham, M, Hoppensteadt, F., Kazanovich, Y., Vinogradova, O., An oscillatory neural network model of sparse distributed memory and novelty detection // Biosystems. 2000. №58 (1-3). P. 265-272.
- Magosso, E., Cuppini, C., Ursino, M., Object segmentation and reconstruction via an oscillatory neural network: interaction among learning, memory, topological organization and γ-band synchronization // Bio Systems. 2006. №85(3). P. 201-218.
- Hendin, O, Horn, D, Tsodyks, M. V., Associative memory and segmentation in an oscillatory neural model of the olfactory bulb // J Computer Neuroscince. 1998. №5(2). P. 157-69.
- Miller, V. H., Jansen, B. H., Oscillatory neural network for pattern recognition: trajectory based classification and supervised learning // Biological Cybernetics. 2008. V. 99. Issue 6. P. 459-471.
- Burwick, T., Oscillatory Neural Networks with Self-Organized Segmentation of Overlapping Patterns // Neural Computation. 2007. V. 19. Issue 8. P. 2093-2123.
- Wong, M. H. Y., Lee, R., Liu, J., Wind shear forecasting by Chaotic Oscillatory-based Neural Networks (CONN) with Lee Oscillator (retrograde signalling) model // Neural Networks. 2008. P. 2040 - 2047.
- Angelini, L., Carlo, F., Marangi, C., Pellicoro, M, Nardullia, M., Stramaglia, S., Clustering by inhomogeneous chaotic maps in landmine detection // Phys. Rev. Lett. 2001. № 86. P. 89-132.
- Angelini, L., Carlo, F., Marangi, C., Pellicoro, M., Nardullia, M., Stramaglia, S., Clustering data by inhomogeneous chaotic map lattices // Phys. Rev. Lett. 2000. № 85. P. 78-102.
- Скворцов А. В. Триангуляция Делоне и ее применение. Томск: Томскийуниверситет. 2002.
- Benderskaya, E. N., Zhukova, S. V., Clustering by chaotic neural networks with mean field calculated via Delaunay triangulation, Proceedings of Third international workshop on Hybrid artificial intelligence systems, Lecture Notes in Computer Science. 2008. V. 5271. Springer-Verlag. P. 408-416.
- Sprott, J. C., Chaos and time-series analysis. Oxford University Press. 2003. P. 507.
- Pikovsky, A., Rosenblum, M., and Kurths,J., Synchronization: A Universal Concept in Nonlinear Sciences. Cambridge University Press. Cambridge. 2003.
- Benderskaya, E. N., Zhukova, S. V., Fragmentary synchronization in chaotic neural network and data mining, Proceedings of 4th international conference on Hybrid artificial intelligence systems, Lecture Notes in Computer Science. 2009. V. 5572. Springer-Verlag.P. 319-326.