350 rub
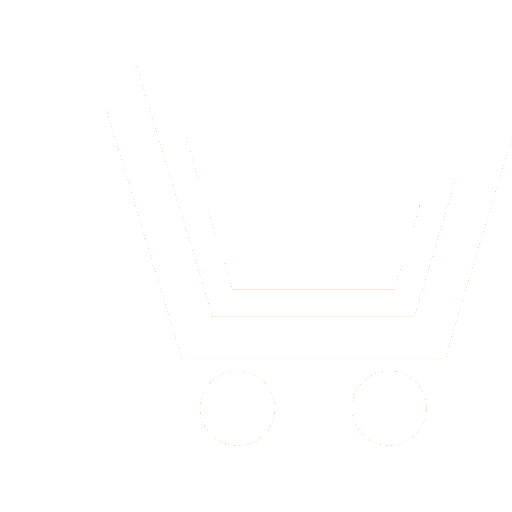
Journal Neurocomputers №7 for 2011 г.
Article in number:
Convergence of weights of a two-layer linear neural network at construction of optimum linear estimations of casual vectors
Keywords:
an artificial neural network
direct and indirect training of a neural network
an optimal linear estimation
casual vectors
Authors:
A. A. Sirota, E. Yu. Mitrofanova
Abstract:
Possibilities of creation optimal in a class of the linear estimations of casual processes and fields on the basis of implementation of procedures of direct and indirect training of two-layer neural networks on set of implementations statistically the bound input and output casual vectors are considered. Theoretical substantiations of convergence of weight factors of a two-layer linear neural network with the reduced number of neurons in the latent layer to components of the latent vectors received at the decision of the generalized task on own values are received. It is shown that on an output of a neural network approach of an optimal linear estimation of an input casual vector in the form of expansion under the first latent vectors of a matrix of a covariance of the estimation which number is equal to number of neurons in the latent layer is formed.
Possibilities of creation optimal in a class of the linear estimations of vectors of unobservable parameters are considered at indirect training of two-layer neural networks on set of implementations of observable casual vectors. It is shown that at adjustment of coefficients of a neural network in the conditions of indirect training under the observable data in the latent layer it is possible to receive an optimal estimation of a vector of unobservable parameters.
Results of statistical simulation modeling on an example of handling of casual fields with a correlation given function are resulted.
Pages: 39-48
References
- Ватолин Д.Методы сжатия данных. М.: Диалог-Мифи. 2002.
- Назаров Л.Е.Применение искусственных нейронных сетей для сжатия РСА - и сканерных изображений земной поверхности // Исследование Земли из космоса. 1999. № 5. С.44-50.
- Хайкин С.Нейронные сети: полный курс. М.: Вильямс. 2005. 1104 с.
- Попов В.Г., Сирота А.А. Свойства сходимости весов автоассоциативной двуслойной линейной нейронной сети при постоении сжимающих отображений случайных // Нейрокомпьютеры: разработка и применение. 2009. № 5. С. 3 - 11.
- Сирота А.А., Попов В.Г., Шульгин В.А. Обнаружение микроколебаний объектов на основе нейросетевых алгоритмов обработки данных голографического интерферометра. Изв. вузов. Радиоэлектроника. 2007. № 4. С. 31-41.
- Алгазинов Э.К., Сирота А.А. Анализ и компьютерное моделирование информационных процессов и систем. М.: Диалог-МИФИ. 2009. 416 с.
- Дрюченко М.А., Воронова Е.В., Сирота А.А. Восстановление регрессионных моделей случайных процессов и полей с использованием нейронных сетей // Вестник Воронежского гос. у-та серия Системный анализ и информационные технологии. 2010 № 1. С. 109 - 119.
- Андерсон Т.Введение в многомерный статистический анализ. Физматлит. 1963. 500 c.
- Фукунага К. Введение в статистическую теорию распознавания образов: Пер. с англ. М.: Наука. 1979. 368 с.
- Корн Г., Корн Т. Справочник по математике (для научных работников и инженеров). Наука. 1974. 832 с.
- Рао С.Р. Линейные статистические методы и их применения. М.: Наука. 1968. 548 с.
- Беллман Р. Введение в теорию матриц. М.:Наука. 1976. 368 с.