350 rub
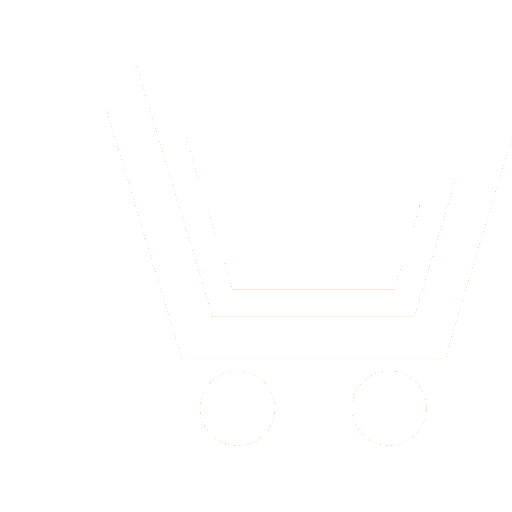
Journal Neurocomputers №7 for 2011 г.
Article in number:
Increase of accuracy of approximation of the non-stationary data linear neural network by a cortege-method of training set
Authors:
E.A. Samoilin, V.M. Chelakhov
Abstract:
The task of definition of parameters of discrete dependence of supervision containing as normal (Gaussian), and non-stationary (abnormal) components is considered. The analysis of applicability of a classical method of the least squares (MLS), method of global numerical optimization and device linear neural networks (NN) to the decision of the given task is carried out (spent). The analysis of these methods has allowed to make important remove (conclusion) consisting that for decrease (reduction) of absolute mistakes estimations of required parameters, the training adaptive linear neuron should begin with abnormal measurements and to come to an end on normal. In a case, when the abnormal measurements take place in index points of time of supervision, the training neuron begins with this anomaly; therefore use of a cortege-method is not required. If Anomaly are in final points of a time interval of supervision, or in regular intervals are distributed (allocated) on this interval, to lower absolute mistakes estimation of parameters probably on the basis of formation of the certain sequence of training pairs (input (entrance) ? output (exit)), such, at which abnormal measurements (at first follow by way of decrease (reduction) «Anomaly»), and then - normal. Thus the sequence of the training pairs generated by the mentioned above rule, is named cortege of training set.
As a cortege-method of training set (i.e. the formations of trains of training pairs) in conditions of the task, formulated in clause, are considered algorithms of construction of variation numbers (lines) deviation of supervision and numbers (lines) of analogues first derivative - local final differences.
Thus, use of a cortege-method defines (determines) a sequence of training adaptive linear neuron, which begins with training pairs having greatest deviation (or the final difference) (i.e. anomaly) and comes to an end on pairs with least deviation (or final difference).
The results of numerical researches submitted in clause, testify about higher accuracy the characteristics linear NN, using an offered method, in comparison with a traditional network, and also classical MLS.
Pages: 20-26
References
- Репин В.Г., Циплихин А.И. Определение точной верхней грани ошибок метода наименьших квадратов // Радиотехника и электроника. 2003. Т. 48. № 1. С. 91-99.
- Шипачев В.С. Высшая математика. М.: Высшая школа. 1998. 479 с.
- Круглов В.В., Борисов В.В. Искусственные нейронные сети. Теория и практика. М.: Горячая линия - Телеком. 2001. 382 с.
- Уидроу Б., Стирнз С. Адаптивная обработка сигналов: Пер с англ. М.: Радио и связь. 1989. 440 с.
- Каллан Р. Основные концепции нейронных сетей: Пер. с англ. М.: Изд. дом «Вильямс». 2003. 228 с.
- Головко В.А. Нейронные сети: обучение, организация и применение. Кн. 10: Учеб. пособие для вузов / под ред. А.И. Галушкина. М.: ИПРЖР. 2000.
- Математика в понятиях, определениях и терминах. Ч. 1 / под ред. Л.В. Сабинина. М.: Просвещение. 1978. 320 с.