350 rub
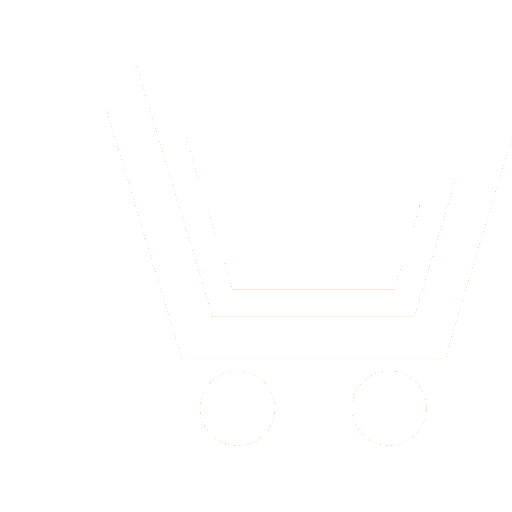
Journal Neurocomputers №7 for 2011 г.
Article in number:
Two level erosion-based clustering method for RBF networks training on incomplete data
Keywords:
erosion
density-based clustering
missing data imputation
RBF neural network
minimum spanning tree
Authors:
V.V. Ayuyev
Abstract:
The paper describes an original model - DEC RBF - for the radial-based neural networks training. The model-s work is based on density-based erosion clustering algorithm. The minimal spanning tree algorithm for cluster size optimization processes initial data domains. Missing data could be imputed independently over each cluster. BF centers are set into the corresponding cluster centers.
Three different domain databases were used as a testing area. An open-access experimental data were differ both by size and attribute number.
Comparative analysis of the proposed model, traditional neural network based architecture, and previously described model was fulfilled. The results showed a slight lack of DEC RBF model-s accuracy in comparison with the best-known solution (which was our previous model), that is due to the much lesser number of BF. In the case of similar amount of BF in a neural network architecture, DEC RBF showed 1,3-2 times better accuracy along with 2-3,5 better performance.
The optimal value of internal parameter was found because of analysis for exogenous and endogenous factors influence on model-s quality rates. One of the key features of the proposed method was fully repeatable result for network train-ing.
Pages: 10-19
References
- Buhmann M.D. Radial Basis Functions: Theory and Implementations. Cambridge: Cambridge University Press. 2003. 271 p.
- Steinbach P.N., Kumar M., Tan V. Introduction to Data Mining. International Edition. NY.: AddisonWesley. 2006. 769 p.
- Хайкин С. Нейронные сети: полный курс, 2-е изд.: Пер. с англ. М.: Вильямс. 2006. 1104 c.
- Тархов Д.А. Нейронные сети. Модели и алгоритмы. Кн. 18. М.: Радиотехника. 2005. 256 с.
- Аюев В.В., Карпухин П.А. Кластерный метод подбора параметров и обучения на неполных данных ИНС Хехт-Нильсона // Информатика и системы управления. 2009. № 1(19). С. 91-103.
- Овсиенко О.С.Гибридная модель кластеризации в сети РБФ // Тр. рег. конф. «Наукоемкие технологии в приборо- и машиностроении». Калуга. 2010. Т. 1. С. 236-240.
- Аунг З.Е., Аюев В.В. Нейронная сеть РБФ на основе аттракторной кластеризации // Системы управления и информационные технологии. 2010. № 4(42). С. 4-8.
- Кормен Т., Лейзерсон Ч., Ривест Р., Штайн К. Алгоритмы: построение и анализ. 2-еизд. // М.: Вильямс. 2005. 1296 с.
- Little R.J.A., Rubin D.B. Statistical Analysis with Missing Data. 2-nd edition. N.Y.: John Wiley and Sons. 2002. 408 p.
- Jiang Y., Zhou Z.H. Editing Training Data for kNN Classifiers with Neural Network Ensemble // Proc. of the 1-st International Symposium on Neural Networks. Dalian. 2004. P. 356-361.
- Bay S.D. Nearest neighbor classification from multiple feature subsets // Intelligent Data Analysis. 1999. V. 3. № 3. P. 191-209.
- I-Cheng Y. Analysis of strength of concrete using design of experiments and neural networks // Journal of Materials in Civil Engineering. 2006. V. 18. № 4. P. 597-604.
- Schafer J.L. Multiple imputation: a primer // Statistical Methods in Medical Research. 1999. V. 8. № 1. P. 3-15.
- Witten I.H., Frank E. Data Mining: Practical machine learning tools and techniques, 3-rd Edition. San Francisco. Morgan Kaufmann. 2011. 629 p.
- Honaker J., King G. What to do About Missing Values in Time Series Cross-Section Data // American Journal of Political Science. 2010. V. 54. № 2. P. 561-581.
- МедведевВ.С., ПотемкинВ.Г. Нейронныесети. MATLAB 6. М.: Диалог-МИФИ. 2002. 496 с.
- Айвазян С.А., Мхитарян В.С. Прикладная статистика. Основы эконометрики. Москва: Юнити-Дана. 2001. 656 с.
- Kolte M.T., Chaudhari D.S., Chopade N.B. Confusion matrix and information transmission analysis using Matlab for evaluation of speech analysis // International Journal of Mathematical Science and Engineering Applications. 2008. V. 2. № 3. P. 243-250.