350 rub
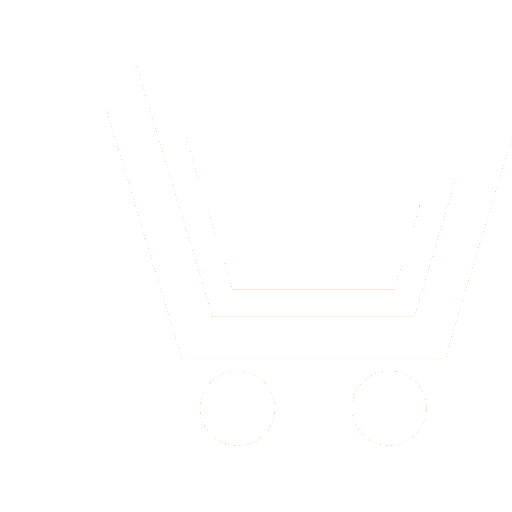
Journal Neurocomputers №4 for 2011 г.
Article in number:
Effective technique of classification flying objects
Keywords:
artificial intelligence
neural network
multilayer perceptron
passive optical system
plane
helicopter
Authors:
P. E. Khrustalyov
Abstract:
In some works as authors a number of the techniques, allowing to receive the information on a class or type of flying object means of passive optical system is offered. However described systems have a number of lacks.
In the given work the approach at which use there are no the negative consequences described proceeding from results of the analysis of existing systems of recognition of flying objects is offered.
For increase of probability of successful recognition of object for a short time interval it is recommended to execute in the beginning binarization of image and only after that to spend search of applicants for a role of flying objects. Then to the allocated contours, objects it is necessary to apply a number of actions: to scale an image segment, to define orientation of flat graphic object, to carry out procedure of classification of object.
Image scaling is carried out according to algorithm of interpolation on the nearest to the neighbor. Definition of orientation of flat graphic object is carried out by an axis finding along which the object and the subsequent combination of the given straight line with a horizontal is extended.
For classification of objects, the multilayered neural network trained on algorithm of back error propagation was used. With a view of reception of high probability of true recognition of object for a short time interval it is necessary to use the optimized ways of training a combination to a technique of increase of generalizing ability of a neural network.
The received results allow to establish the fact of overcoming of lacks of classification of the flying objects inherent in existing systems, by means of application of a technique of the recognition described in given work.
Pages: 62-67
References
- Zhenyu Wang, Yi Wu, Jinqiao Wang, Hanqing Lu, Target Tracking in Infrared Image Sequences Using Diverse AdaBoostSVM. Materials of the First International Conference on Innovative Computing, Information and Control (ICICIC 2006), 30 August - 1 September 2006. Beijing, China. V. 2. P. 233 - 236.
- Dijk, J., van Eekeren, A. W. M., Schutte, K., de Lange, D. J., van Vliet, L. J., Superresolution reconstruction for moving point target detection. OpticalEngineering 47(09). 2008.
- Пережигин А. А., Хачумов В. М. Обнаружение и автоматическое определение параметров летательного объекта на видеопотоке // Информационные технологии и вычислительные системы. 2005. № 1. С. 38-48.
- Борисова И. В., Ерошкин Р. Э., Кузнецов А. А., Опарин А. Н., Осьминко А. Н., Попов П. Г., Шишкин В. А. Обнаружение динамических малоразмерных объектов в различных спектральных диапазонах // Прикладная физика. 2006. № 5. С. 100-102.
- Талалаев А. А., Тищенко И. П., Фраленко В. П., Хачумов В. М. Анализ эффективности применения искусственных нейронных сетей для решения задач распознавания, сжатия и прогнозирования // Искусственный интеллект и принятие решений. 2008. № 2. С. 24 - 33.
- Хорн Б. К. П.Зрение роботов: Пер с англ. М.: Мир. 1989.
- Хрусталев П. Е.Разработка программного комплекса «Миранда» для распознавания образов // Межрегиональная студенческая конференция «Роль студенческих научных обществ в инновационном развитии регионов»: Сб. материалов / под общ. ред. М. С. Хозяинова. Дубна: Междунар. ун-т природы, о-ва и человека «Дубна». 2008.
С. 34 - 36. - Хрусталев П. Е.Продуктивное развитие обобщающей способности многослойного персептрона // Нейрокомпьютеры: разработка, применение. 2011. № 2. С. 17 - 20.