350 rub
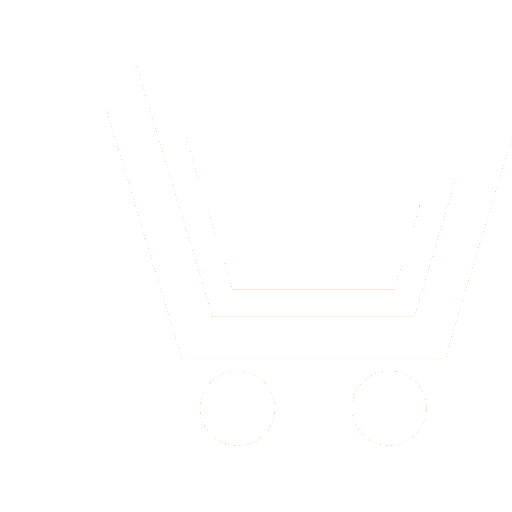
Journal Neurocomputers №2 for 2011 г.
Article in number:
Ann RBF parameters adjustment on the data with missing completely at random information
Keywords:
RBF network
static clustering
data imputation
missing completely at random data
minimum spanning tree
neural-based prediction
Authors:
V. V. Ayuyev, Z. Y. Aung
Abstract:
Artificial neural networks with local approximation abilities become more common approach for a variety of tasks due to their tolerance to a high-dimensional data and large training set volumes. However, missing values in a training set is often a problem for a wide known class of such networks - Radial Basis Network (RBF). Common ways for dealing with missing data is often not an option as an effective solution for that problem.
The work describes a new model for RBF neural network parameters adjustment that based on static clustering approach. Apart from being quite good for handling with missing completely at random data, this method provides an alternative solution for the optimal estimation of basis function number. Radial neuron centers can also be effectively assigned. The model is based on both computational matrixes and cluster data recycling, which were set along with the main cycle of data imputation process. Particularly, two additional minimum spanning tree-based clustering procedures were applied for both large clusters partition, and small clusters merge. This is due to fix some problems with suboptimal setting for clustering algorithm parameter, and processing data characteristics.
The higher overall efficiency of the proposed model compared with traditional RBF neural network was confirmed by a majority of the experimental results on public domain datasets with different fraction of missing data.
Pages: 30-37
References
- Buhmann, M. D., Radial Basis Functions: Theory and Implementations. Cambridge: Cambridge University Press, 2003.
- Gan, G., Ma, C., and Wu, J., Data Clustering: Theory, Algorithms, and Applications / ASA-SIAM Series on Statistics and Applied Probability. Philadelphia: SIAMPress. 2007.
- Хайкин С. Нейронные сети: полный курс, 2-е изд.: Пер. с англ. М.: Вильямс. 2006.
- Тархов Д. А. Нейронные сети. Модели и алгоритмы. Кн. 18. М.: Радиотехника. 2005.
- Marwala, T., Computational Intelligence for Missing Data Imputation, Estimation, and Management: Knowledge Optimization Techniques. N. Y.: Hershey. 2009.
- Little, R. J. A. and Rubin, D. B., Statistical Analysis with Missing Data. 2-nd edition. N. Y.: JohnWileyandSons. 2002.
- Аюев В. В., Тура А., Лайн Н. Н., Логинова М. Б. Метод быстрой динамической кластеризации неоднородных данных // Системы управления и информационные технологии. 2008. № 3(33). С. 26-29.
- Ayuyev, V. V., Jupin, J., Harris, P. W., and Obradovic, Z., Dynamic Clustering-Based Estimation of Missing Values in Mixed Type Data // Proc. 11-th Int. Conf. on Data Warehousing and Knowledge Discovery (Linz, Austria). 2009. P. 366-377.
- Аюев В. В., Карпухин П. А. Кластерный метод подбора параметров и обучения на неполных данных ИНС Хехт-Нильсона // Информатика и системы управления. 2009. № 1(19). С. 91-103.
- Аюев В. В., Аунг З. Е., Тейн Ч. М., Логинова М. Б.Кластерныйметод восстановления пропусков данных для обучения ИНС // Нейрокомпьютеры: разработка, применение. 2009. № 7. С. 23-34.
- Кормен Т., Лейзерсон Ч., Ривест Р., Штайн К. Алгоритмы: построение и анализ. 2-е изд. М.: Вильямс. 2005.
- I-Cheng, Y., Analysis of strength of concrete using design of experiments and neural networks // Journal of Materials in Civil Engineering. 2006. V. 18. No. 4. P. 597-604.
- Uysal, I. and Guvenir, H. A., Instance-Based Regression by Partitioning Feature Projections // Applied Intelligence. 2004. V. 21. No. 1. P. 57-79.
- Schafer, J. L., Multiple imputation: a primer // Statistical Methods in Medical Research. 1999. V. 8, No. 1. P. 3-15.
- Landerman, L. R., Land, K. C., and Pieper, C. F., An Empirical Evaluation of the Predictive Mean Matching Method for Imputing Missing Values // Sociological Methods & Research. 1997. V. 26, No. 1. P. 3-33.
- Gelman, A. and Hill, J., Data Analysis Using Regression and Multilevel / Hierarchical Models. Cambridge: Cambridge University Press. 2007.
- Witten, I. H. and Frank, E., Data Mining: Practical machine learning tools and techniques. 2-nd Edition. San Francisco: Morgan Kaufmann. 2005.
- Oudshoorn, C. G. M., Buuren, V. S., and Rijckevorsel, V., Flexible Multiple Imputation by Chained Equations of the AVO-95 Survey // In TNO Prevention and Health. Report PG/VGZ/99.045. 1999.
- Honaker, J. and King, G., What to do About Missing Values in Time Series Cross-Section Data // American Journal of Political Science. 2010. V. 54. No. 2. P. 561-581.
- Медведев В. С., Потемкин В. Г. Нейронные сети. MATLAB6. М.: Диалог-МИФИ. 2002.